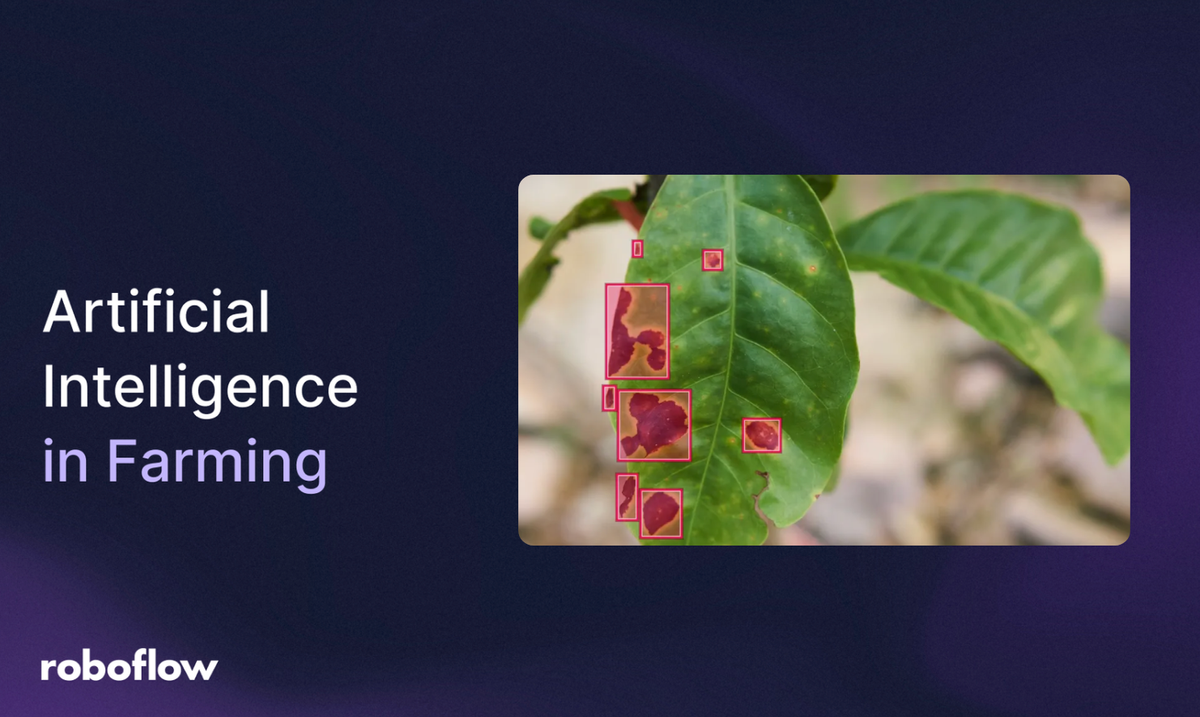
Every year, pests destroy about 40% of global agricultural productivity, costing the industry over $70 billion. But thanks to advancements in computer vision, farmers can now identify and eliminate these destructive pests with precision, boosting crop yields and protecting profits. By leveraging artificial intelligence (AI), farmers can improve efficiency, reduce waste, and safeguard their harvests.
Agriculture is uniquely positioned for disruption by AI according to McKinsey. The industry generates vast amounts of unstructured data, depends heavily on labor, and faces complex supply chain challenges—all while enduring long research and development cycles. With so many farmers seeking customized, cost-effective solutions, AI-powered technology such as computer vision can provide targeted insights that drive efficiency and ensure healthier, more productive crops.
Let's take a look at a few of the essential ways AI is already used in farming today.
Explore AI in Farming
From field management to produce grading, AI-powered solutions enable farmers to tackle challenges that once seemed insurmountable. Traditional methods of managing pests, diseases, and field conditions tended to rely on labor-intensive manual labor or broad application of chemicals. However, computer vision technology now allows for real-time, targeted actions that improve crop yields, reduce waste, and minimize environmental impact.
Agriculture has all the raw elements to be improved with visual sense. From the time of planting and identifying that your rows are equally spaced, to identifying that seeds are the right seeds, to monitoring a given growing season and ensuring that there aren't weeds or pests - there are numerous use cases. Even after harvest you want to identify the quality of the crop, and computer vision can assist. Here are some of the main use cases.
1. Weed identification
One of the most impactful applications of computer vision in agriculture is real-time weed identification, and the corresponding reduction in use of harmful chemicals. Weed species reduce plant production by about 31.5% each year, leading to economic losses of about $32 billion annually. Traditional weed control methods rely heavily on manual labor or indiscriminate herbicide spraying. Now AI-powered vision system can accurately distinguishing between crops and weeds at scale.
Modern AI-enabled sprayers feature booms—large arms equipped with evenly spaced nozzles that can span up to 120 feet wide—to distribute herbicides with precision. Advanced onboard cameras and processors analyze the field in real time, identifying weeds and directing treatment only where necessary. Combined with automated driving technology, which leverages GPS and correction signals, these intelligent sprayers navigate fields with centimeter-level accuracy. For example, John Deere’s See & Spray technology uses AI-enabled cameras and sensors to scan fields in real time.
As a result of these advancements, farmers can reduce herbicide use by up to two-thirds, minimizing chemical runoff while improving overall efficiency.
As another example, Barn Own Tech is using computer vision to assist with faster production of challenger crops and row crops. Using Roboflow and drone-recorded field imagery, they're able to surface where they should spend time to go hand pull weeds, because they're not using herbicides, and time must be used wisely.
2. Plant disease detection
Traditionally disease identification relies on manual scouting, which can be time-consuming and prone to human error. Now AI-powered image recognition enables rapid, large-scale disease detection, helping to prevent outbreaks before they spread.
AI models can identify subtle disease symptoms—such as leaf discoloration, spots, lesions, and wilting—with precision. For example, Microsoft’s AI Sowing App has helped farmers in India detect fungal infections in crops using smartphone cameras. The AI Sowing App draws on more than 30 years of climate data, combined with real-time weather information, and then uses sophisticated forecasting models powered by computer vision software to determine the optimal time to plant, the ideal sowing depth, how much farm manure to apply, and more. That information is then shared with farmers through text messages that they receive on a basic feature phone.
Similarly, PlantVillage, an open-source tool developed by Penn State University, uses deep learning to diagnose diseases in cassava, maize, and wheat with high accuracy. In commercial agriculture, drones equipped with multispectral and hyperspectral cameras scan entire fields, identifying areas of plant stress days before symptoms become visible to the human eye.
3. Field management
Computer vision is redefining field management, enabling farmers to monitor crop health and optimize irrigation with data-driven insights. Drones and satellite imagery, combined with deep learning models, can detect variations in plant health, soil moisture levels, and nutrient deficiencies across vast farmland. For instance, NDVI (Normalized Difference Vegetation Index) imaging helps farmers assess plant vigor by analyzing how crops reflect light.
This technology is also used in predicting overall crop yields by analyzing the number of mature fruits or vegetables present in a field, giving farmers a more accurate forecast of their harvest.
4. Produce grading
Computer vision systems capture detailed images of produce as it moves along the conveyor belt. AI-powered algorithms then analyze these images to assess critical quality factors like size, shape, color, texture, and ripeness.
For example, in apple grading, computer vision systems evaluate the size of the apples, check for skin blemishes, and assess color consistency to classify them into various quality grades. These systems can be trained to detect subtle defects like bruising, rot, or pest damage that might be missed by the human eye. That way damaged or subpar items can be removed before they reach consumers.
5. Autonomous harvesting vehicles
The American Farm Bureau Federation estimates there are roughly 2.4 million farm jobs that need to be filled annually. Autonomous vehicles used for both harvesting and transporting crops can help. Today, self-driving tractors and harvesters use computer vision systems to navigate fields, detect obstacles, and harvest crops with precision. Once harvested, the same vision systems guide the autonomous transport vehicles to collect and move the crops to storage or packing facilities.
With John Deere and Trimble's second-generation autonomous tractor, featuring 16 individual cameras arranged in pods to enable a 360-degree view of the field, farmers can step away from the machine and focus their time on other important jobs during tillage.
Meanwhile, another company is using computer vision to manage farm machinery maintenance more effectively. They're using vision to identify the make and model of their heavy equipment, so that they can better pair technicians with exact expertise to go to the field for fixes.
How to Use Artificial Intelligence in Farming
Agriculture operates at at such a large scale that small percentage changes have big impacts on cost or profits. Furthermore, agriculture is a largely repetitive process to monitor yields and monitor the way fields are working: row crops look pretty similar, so identifying anomalies can be an easier task. Vision is uniquely suited to the field to help improve margins and quality, while keeping costs under control.
Here's how to get started:
- Have a narrowly, impactful defined problem - e.g. one specific pest, one specific weed.
- Start at a place where you already have some amount of image or video data.
- Think through your expertise - do you know specific types of plants...in what areas can you best use your knowledge to label data?
- When you start to think about building a model, don't think of it as an end point. You can deploy a model, but continue to improve it - look at different lighting, etc - so your model doesn't drift.
Already computer vision is changing the way crops are harvested, weeds are removed, and making greenhouses more efficient. There's also a focus on vehicle and human collision monitoring, as well as monitoring to ensure animals and wildlife are kept safe.
Unlock the full potential of AI in agriculture with Roboflow Enterprise. Roboflow simplifies the process of building custom computer vision models, enabling farmers to identify and manage key issues like pests, weeds, and plant diseases with greater accuracy. By leveraging Roboflow's intuitive computer vision platform, you can stay ahead of challenges, optimize operations, and ultimately protect profits while promoting sustainable farming practices.
Cite this Post
Use the following entry to cite this post in your research:
Trevor Lynn. (Feb 17, 2025). AI in Farming: 5 Ways Computer Vision Is Transforming Agriculture. Roboflow Blog: https://blog.roboflow.com/ai-in-farming/
Discuss this Post
If you have any questions about this blog post, start a discussion on the Roboflow Forum.