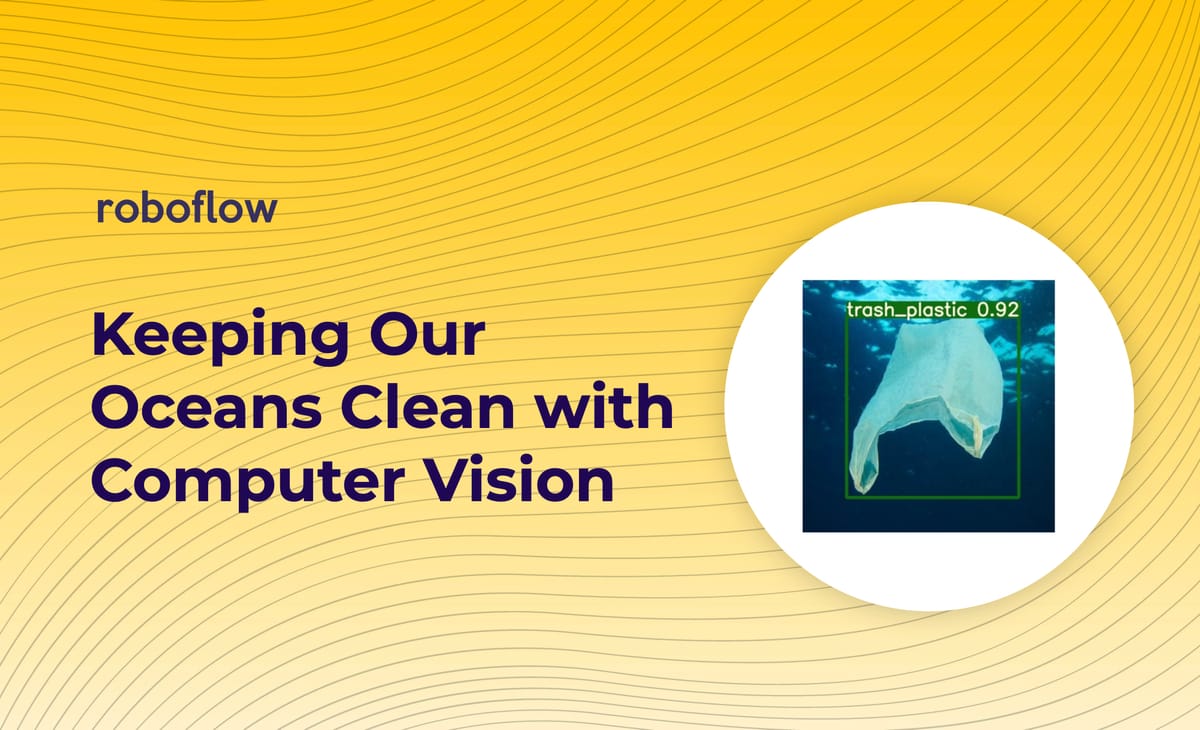
Global plastic production has exceeded 500 million tons. Moreover, estimates from the US Environmental Protection Agency indicate that 30 percent of all produced plastic will end up in the oceans.
With this in mind, researchers Gautam Tata, Sarah-Jeanne Royer, Olivier Poirion, and Jay Lowe from CSU Monterey Bay, The Ocean Cleanup, and UC San Diego considered how computer vision can play a role in keeping our oceans clean.
The group recently published their findings: DeepPlastic: A Novel Approach to Detecting Epipelagic Bound. In the paper, the research team contemplates what the efficacy of autonomous underwater vehicles (AUVs) may be like if tasked with automatically identifying and collecting underwater plastics.
The team focuses on assessing the accuracy of deep learning based approaches to the identification of underwater plastics.
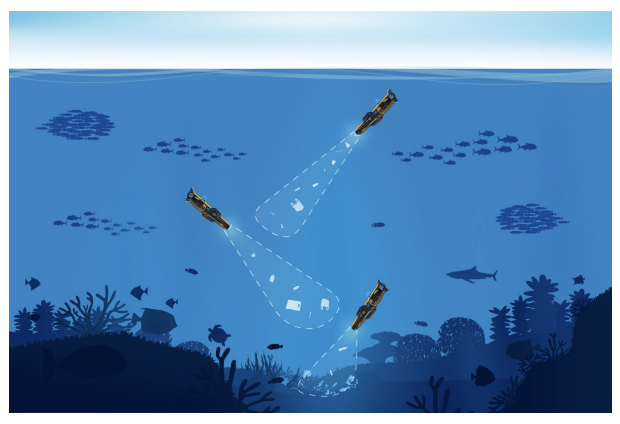
Notably, the researchers focused on collecting a dataset from real world conditions:
The dataset was built from images taken across three sites throughout California (South Lake Tahoe, Bodega Bay, San Francisco Bay) along with a compendium of images hosted by research institutions on the internet to increase the representation of marine debris plastics in different locations. The primary source of internet images were underwater photos taken by the Japan Agency for Marine-Earth Science and Technology (JAMSTEC). The training dataset consists of 3200 total images.
Once having imagery collected, the team leveraged tools like Roboflow for organization, annotation, and augmentation to increase dataset cleanliness and variability.
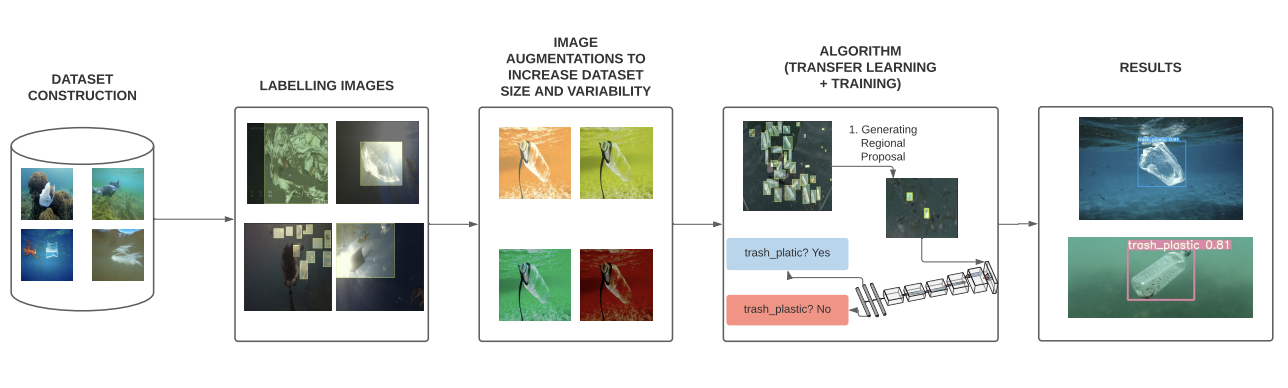
Upon comparing multiple model architectures like YOLOv4, YOLOv4-tiny, and YOLOv5, the researchers conclude YOLOv5 may best balance accuracy and inference speed for an on-device edge deployment, achieving 0.98 mAP and a throughput of 1.4 ms per image (running on a Tesla V100).
Given the team's emphasis on real world data collection from varied conditions, the model generalizes fairly well to plastics in an array of water conditions (color, brightness, and closeness).
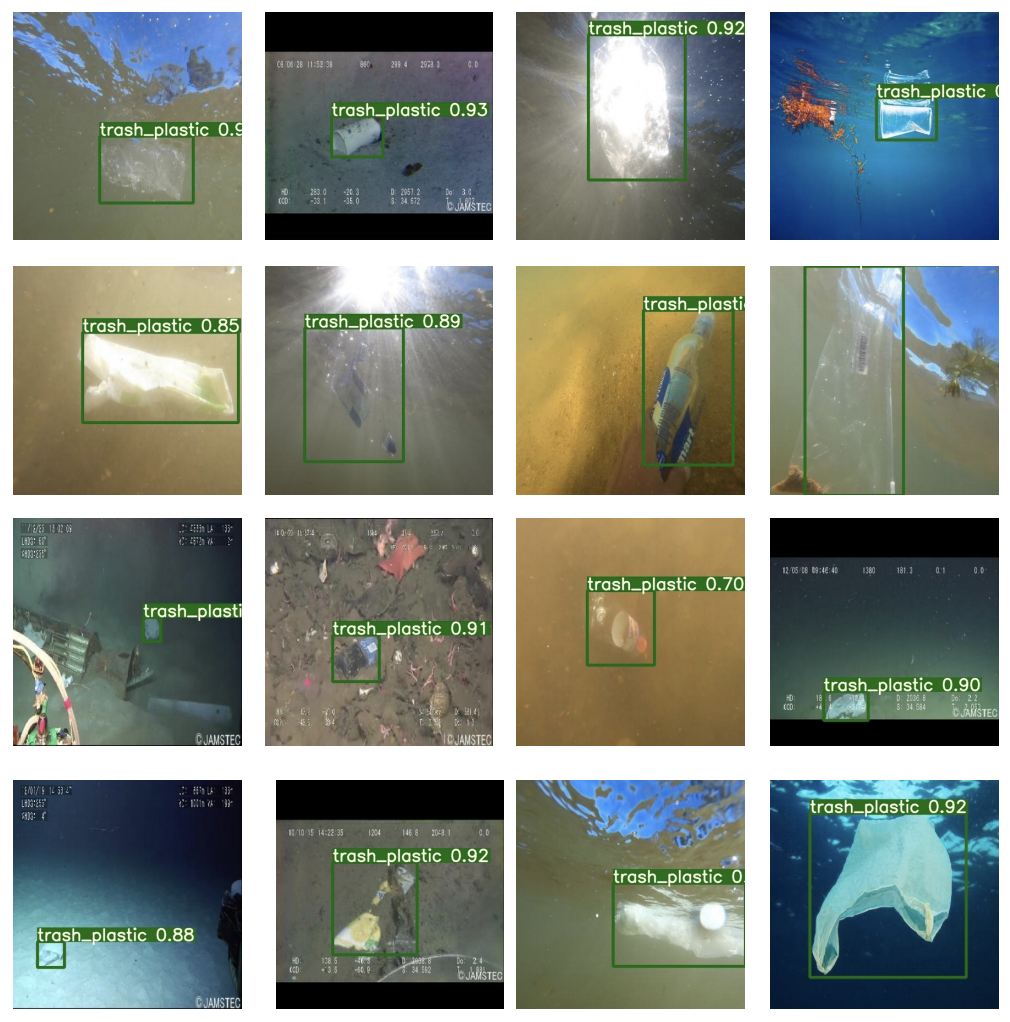
The team notes the model is not perfect, however, as misclassified objects are possible and further dataset collection as well as model iteration should be pursued in future prototypes. Read the team's full research paper on Arxiv.
Ultimately, we're excited to continue to see creative applications of computer vision, like those that will improve environmental health!
Using Roboflow for your own research? Email us hello [at] roboflow.com for your academic use licenses with increased usage limits.
Cite this Post
Use the following entry to cite this post in your research:
Joseph Nelson. (Jul 19, 2021). Using Computer Vision to Clean the World's Oceans. Roboflow Blog: https://blog.roboflow.com/computer-vision-clean-oceans/