What is causing the sea lion population to decrease? Is it illegal hunting? Is it shark and killer whale predation? Or maybe it’s overfishing, causing the sea lions to starve? Researchers want to determine the root cause.
This article describes the use of computer vision to help the conservation of this endangered species. Explore the dataset and model used in this story.
Problem: Steller Sea Lion Depopulation
According to the National Oceanic and Atmospheric Administration (NOAA), Steller sea lion numbers in the western Aleutian Islands of Alaska have declined by 94% over the last 30 years, with the root cause still undiscovered. This is puzzling to biologists, since sea lion populations in other regions, such as in the eastern Aleutian Islands, are steadily increasing. You can learn more about the endangered Steller sea lion at the NOAA website.
Studying Sea Lion Behavior
Researchers are studying Steller sea lion behaviors to collect more data that could help them pinpoint the cause of the decline, thereby providing guidance on how to preserve the sea lions. Areas of study include:
- Movement Patterns: Sea lion migration patterns inform biologists of where the sea lions choose to live and whether this location changes during the course of their lifetime.
- Survival Rates: Sea lion survival rates assess the likelihood that pups will survive until the next year of their lives, and how these rates vary depending on the age and location of the animals.
- Birth Rates: Sea lion birth rates track how many pups each female sea lion has throughout its lifetime, and how often females bear pups.
Any information about the sea lions gathered by the researchers could be useful in finding a possible link between population declines and specific environmental factors, allowing NOAA to respond accordingly.
Certainly, the use of advanced technologies, including machine learning, can significantly enhance researchers’ efforts.
Marked Sea Lions and Manual Monitoring
To learn more about the Steller sea lion population, NOAA biologists chose to study specific individuals that would represent the status of the entire western Aleutian population.
Scientists marked a small portion, about 4%, of sea lions when they were pups with letter and number codes that identify where the sea lion was born and marking location. In the following image, a sea lion is marked with “M625”. The “M” indicates that the sea lion was born and marked in Medny Island; “625” is a unique identifier of this specific sea lion.
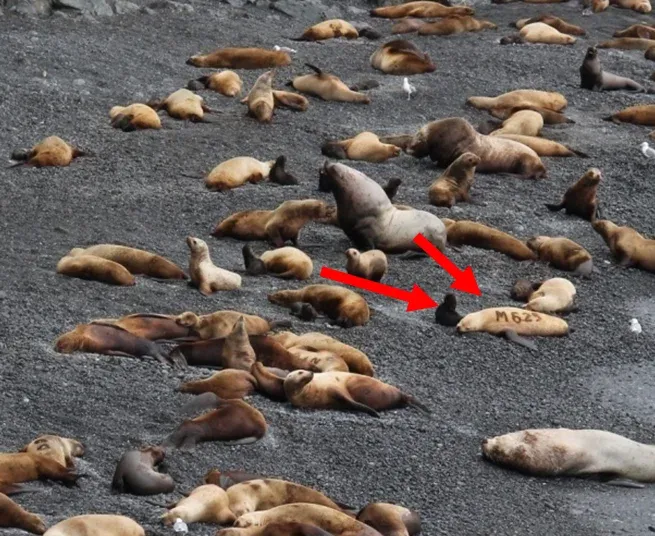
NOAA maintains a database of the marked sea lions, with the associated locations and dates of each animal’s sightings.
For example, sea lion M625 was spotted by Russian scientists on the Kamchatka Peninsula when she was a few years old. Several years later, she was spotted again on Medney Island, where she returned to have her first pup. Through multiple sightings over the years, scientists have been able to observe her travels and count the number of her pups, which are now three as of 2022.
Remote Cameras for Sea Lion Monitoring
NOAA researchers set up remote cameras capturing one photo every 10 minutes during daylight hours, year-round. The 20 remote cameras currently collectively take 400,000 images per year.
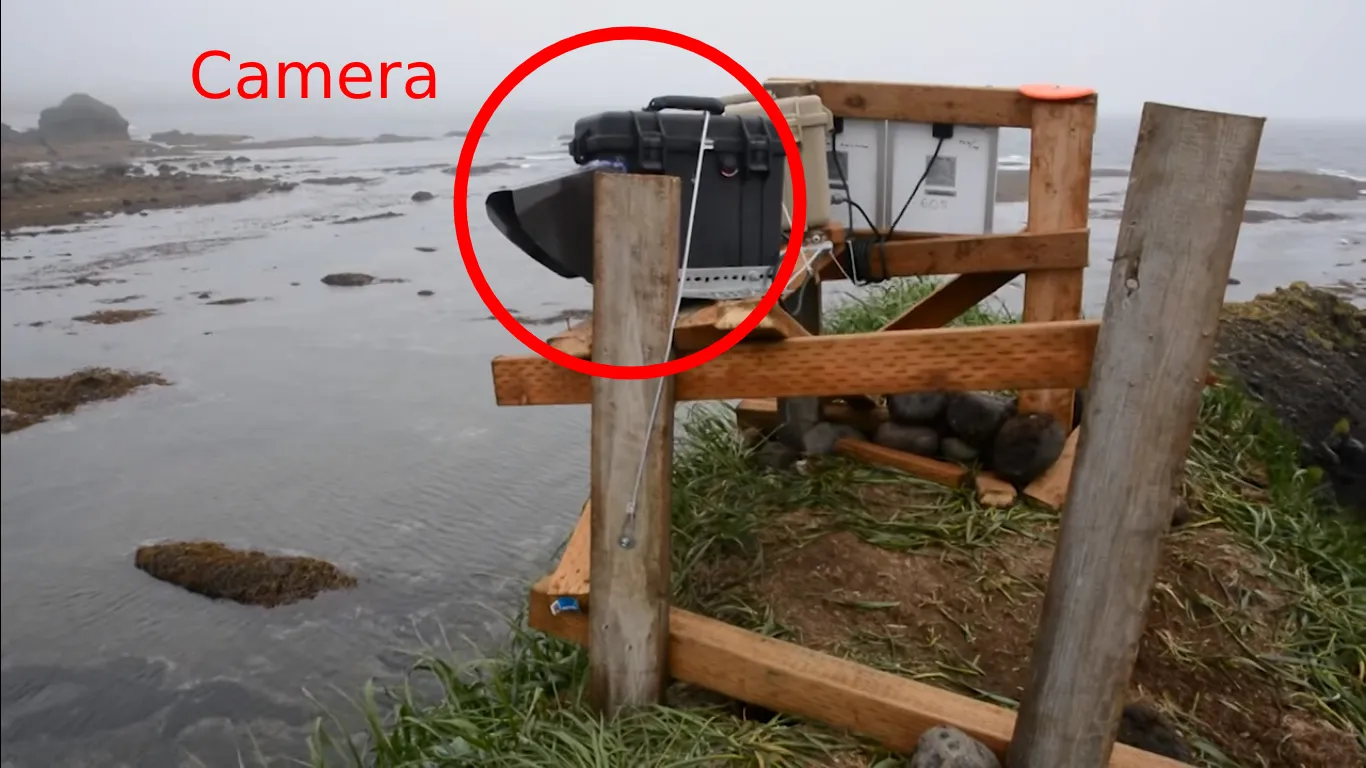
The hope is that marked sea lions would appear in as many images as possible. Researchers and volunteers have to manually sift through the database of images to see which pictures contain marked sea lions – a tedious and time intensive process.
Images from Zooniverse
Making the process even more difficult is the fact that some images do not contain sea lions at all due to being unclear, for a variety of different reasons. The cameras get covered in water droplets, obstructed by snow, blurred by intense fog, or even covered up by eagle feathers!
Currently, when researchers and volunteers go through the images, they perform two steps:
- Filter out the images that are unclear or do not contain sea lions.
- Manually go through the remaining images to see which contain marked sea lions.
This manual and time consuming process is a great area for computer vision to assist researchers.
Steller Sight Automates Filtering Images
Steller Sight was developed, with the support of Roboflow, to automate the first step of this tiresome task, using computer vision technology to quickly determine the presence or absence of sea lions in each image.
The Steller Sight Python script uploads images to Roboflow, retrieving the coordinates of each bounding box and the confidence rating of each detection. Using this information, the software detects the sea lions and draws boxes around each sea lion found.
At the top of the image, the program labels the image as “No Sea Lions” or “Sea Lions Present” as shown in the following examples.
Steller Sight saves researchers time, given that approximately a third to a half of the images do not contain any sea lions and researchers should not spend time manually scanning the images for sea lions.
The software has a high accuracy rating of 97% for correctly distinguishing the presence of sea lions. High accuracy means that the software is capable of spotting sea lions present that otherwise the human eye might overlook. The processing speed of one image per second is about 10 times faster than manual review.
Images from Zooniverse
Steller Sight and the Potential of Computer Vision
Steller Sight can be scaled and trained to assist researchers in various conservation efforts. The same Robolow technology can be applied to detect killer whales in support of the “Killer Whale Count” project, whose research team works with NOAA to assess the impact of killer whale predation on sea lion depopulation.