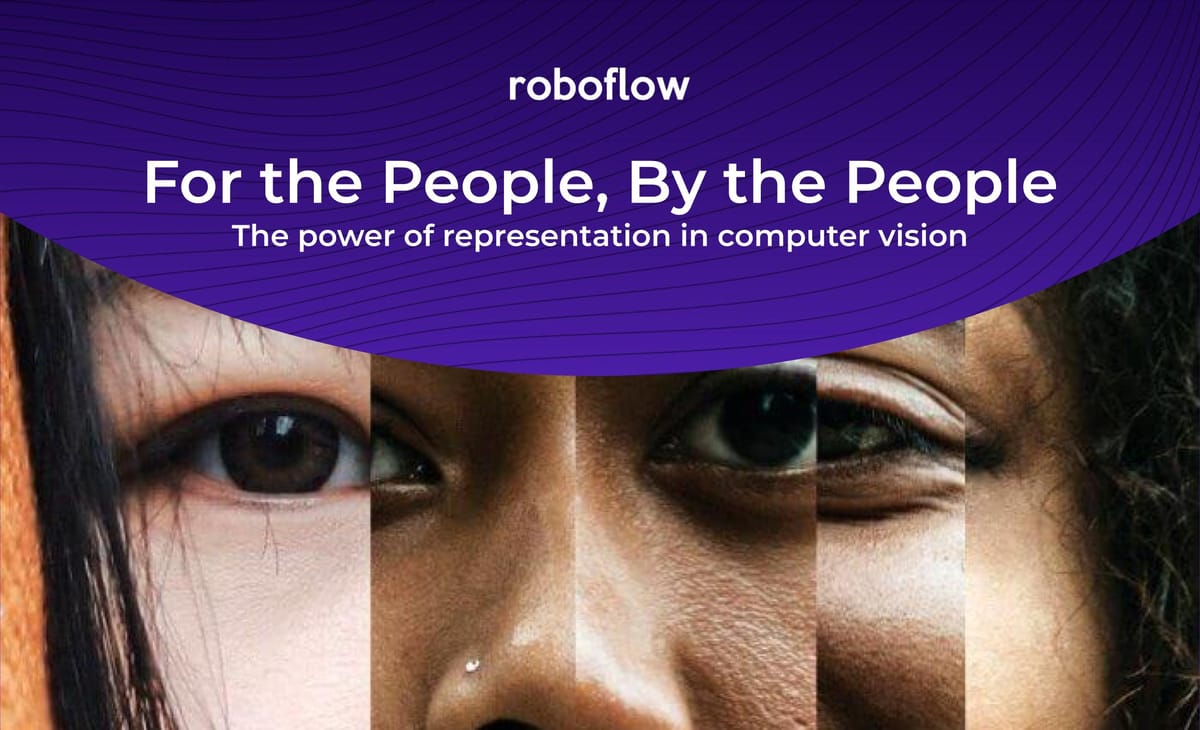
Computer vision, on the whole, is an ambitious undertaking.
We are developing technology that can see the world as we see it - to recognize simple objects like trees and croissants, and more complex occurrences like oil and methane leaks. Today, models can even read license plates and receipts. Computer vision is already changing our world, its applications both expansive and breathtaking.
When we talk about the importance of representative data, we usually mean it in the context of the environment around the object(s) we are teaching a model to detect. For example, if we’re training a model to recognize lug nuts on a tire, we’d want our dataset to include images of lug nuts in both bright and dim lighting, on different types of hubcaps, and at any angle or rotation of the tire.
These same tenants hold true, of course, when we encounter significant diversity in the objects themselves - for example, in human detection. Consider the soap dispenser that is equipped with a tiny camera to detect the presence of hands; if you only train that model on images of white/Caucasian hands, soap won’t be dispensed to anyone with dark skin. Extrapolate this to pedestrian detection for self-driving cars, and the importance of representative data in the training set becomes both apparent and urgent.
In many ways, these models reflect our own cultural biases back at us, highlighting the gaps in the diversity of not only our source dataset, but perhaps (more broadly) our worldview.
We teach our models in much the same way you might teach a child - but in the real world, forces outside our control can influence childhood development. This is not true when training a computer vision model, a fact that is both an advantage and a drawback for the vision architect. Machine learning is isolated from outside influence, and only if the engineer has employed active learning - or the process by which we collect inference data to feed back into our training set - will the model improve as it encounters true diversity in the wild.
As a result, disparities in model performance can almost always be attributed back to human error and/or oversight – so in many ways, these models reflect our own cultural biases back at us, highlighting the gaps in the diversity of not only our source dataset, but perhaps (more broadly) our worldview.
How should we define diversity?
Certainly, skin pigmentation isn’t the only variable we must consider when we set out to build a model that can detect humans in any context or capacity. Depending on the unique use-case and purpose of the model itself, there are many variables we can anticipate it might encounter in deployment.
We’ve outlined a few below, and while this list is nowhere near exhaustive, it is (at the very least) intended to start the dialogue, to get our customers’ wheels turning, and to make the case that inclusivity is an essential component in the field of computer vision.
Accessibility
Believe it or not, places of public accommodation and buildings constructed by state or local governments weren’t required to be fully accessible to people with disabilities until 1992. Since then, we’ve come a long way towards ensuring that not just our government buildings, but our communities at-large, are accessible to people of all abilities - and yet there is still work to be done. As different industries explore and adopt computer vision into existing workflows and for new products and services, keeping accessibility at the forefront is paramount; we ignore this consideration at the risk of undoing the progress our society has made in the last three decades.
Applications of relevance are everywhere. Consider the model that counts individuals as they enter public spaces (to keep capacity at-or-below the fire code), or that can evaluate the wait-time by determining how many people are in-line for the best rides at an amusement park - do these models know to recognize people sitting and standing? Using crutches or a cane?
Religious Diversity
By definition, computer vision relies solely on imagery to function. It is a technology that is trained on visual data (inputs) and can therefore only generate model predictions (outputs) by way of interpreting video footage or still frames. Computers can’t “see” religion, spirituality or even morality, for that matter - and it would naturally follow that this technology can’t therefore be more or less inclusive of human subjects based on their intrinsic beliefs.
This is true, of course, but models can see variation in attire - and for some of us, our clothes reflect not only who we are, but what we believe. If you’re training a model to detect human ears, as an example, how will your model respond when it encounters ears partially occluded by head dresses, hair coverings, wraps or ornamental jewelry?
We can ensure our models are responsive to these differing presentations by proactively addressing them as known gaps in our dataset. This could mean incorporating images of people wearing burkas, yamakas, bonnets and hijabs - an awareness that will improve model performance for all people, regardless of their religious beliefs.
Gender Fluidity
June is Pride Month, and we’d be remiss if we left out the many vibrant and interwoven complexities of gender identity and sexual orientation from this topic. Some computer vision applications might require the model to understand and differentiate people based on their preferred gender expression. Herein lies an opportunity to develop vision technology that exists outside the heteronormative framework - a framework that, until today, has constrained innovation across industries in ways we perhaps haven’t yet fully realized or appreciated.
One good example can be found in air travel. The TSA body scanner at most airports requires an agent to press a “female” or “male” icon before the passenger steps into the machine to be scanned. These scanners do not offer the agents a gender neutral option, a consequential omission that leads to regular and unnecessary pat downs. This technology wasn’t developed to account for the inevitable fluidity of its subjects; as a result, it’s a less effective (and less efficient) machine.
We know now that technology plays a key role in shaping our cultural understanding (and acceptance) of many marginalized groups. Computer vision is no exception. In fact, it could be said that we are building the models that will lay the foundation for the future of our world. What should that future look like? Who is “seen” by the machines, and who is left behind?
Democratizing Computer Vision
Creating representative datasets is not only important for ethical reasons, it’s congruent with good business. The process of considering and identifying variance (in the form of diversity) that our models may encounter in the wild will always result in better performance.
The question we posit today touches a vulnerability that is shared across all democratic systems: can computer vision escape the intrinsic biases of the human condition? After all, models are only as equitable as the people who create them. This technology has no concept of racism or ableism, no preference for any particular religion or set of beliefs, and it is not constrained within the gender binary - these are uniquely human traits, and they are taught to us both directly and implicitly throughout our lifetimes.
As we set out to create a world both vastly changed and significantly improved by computer vision, it is our responsibility to ensure those impacts are felt across borders and beliefs - to build machines that represent the best of us, to improve our lives and safeguard our planet, that never learn to automate the flaws of humans.
Cite this Post
Use the following entry to cite this post in your research:
Kaylee Williams. (Jun 20, 2021). For the People, By the People. Roboflow Blog: https://blog.roboflow.com/for-the-people-by-the-people/