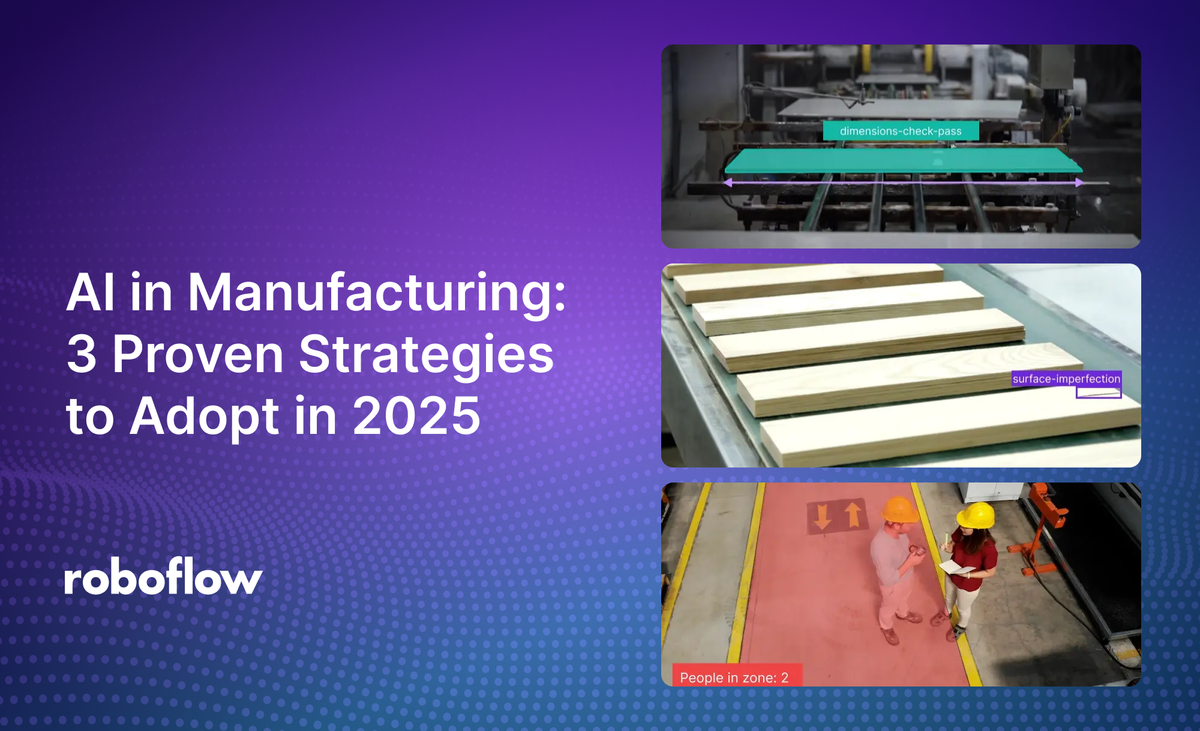
Over the past year, we've seen manufacturers enthusiastically integrating vision AI into their production processes – whether they're making industrial machinery, commercial vehicles, or materials used in construction and infrastructure projects.
The buzz around machine learning and artificial intelligence has fueled widespread experimentation across industries. That said, experimenting with AI is simple; the hard part is getting into production and scaling results effectively.
“Achieving positive results using AI in a lab environment is easy, but the real challenge comes when scaling the solution across a network like ours without disrupting day-to-day operations. Our partnership with Roboflow is allowing us to do just that.”
- Asim Ghanchi, AVP of Technology, BNSF Railway,
It’s an exciting time for AI in manufacturing; past investments in the technology are yielding impressive results. For example, a maker of agricultural equipment saved at least $8 million at each facility where they adopted vision AI. Another company reduced time spent updating inventory by 90%. Yet another manufacturer cut the number of customer returns in half after implementing AI-assisted quality inspections.
Proven strategies for visual AI in manufacturing
If you’re just getting started with AI, where is the immediate ROI? Based on successful AI deployments among manufacturers using Roboflow, here are the highest value places to consider implementing AI immediately:
- Detecting jams, pileups, or bunching along assembly lines
- Identifying quality issues and defects in products, packaging, labels
- Alerting facility staff to safety and health hazards
Now let's dig into these common challenges and see real-world stories from manufacturers that solved them with visual AI.
Solution 1: Avoiding jams and pileups
Our first story comes from a company that produces building and construction materials. In the past, as products moved along their production line, if one item was angled or sized incorrectly, it would get snagged and jam machinery. This resulted in unplanned downtime, damaged products, and time lost clearing the line.
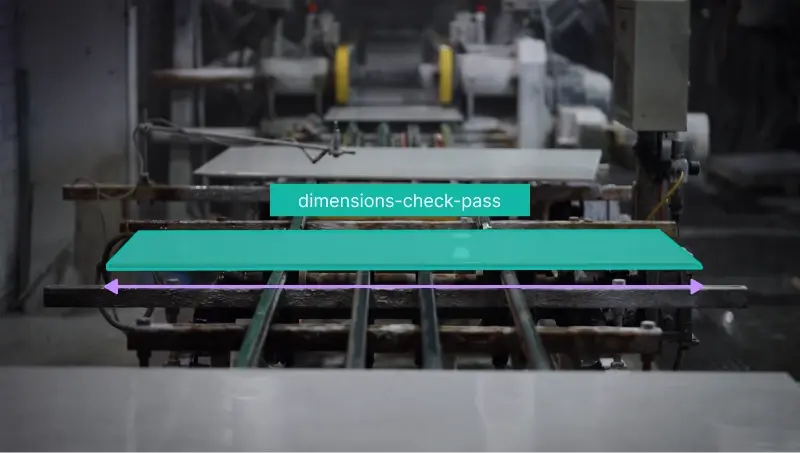
To avoid these costly pileups, the company rolled out an AI-assisted monitoring system that continuously tracks goods moving along the production line. It automatically identifies when a component is the wrong size or angled incorrectly. When a problem is detected, it triggers an alarm for production crews to resolve the issue proactively.
During the implementation process, the system identified an average of nine daily issues, each of which could have caused an hour of downtime. That translates to around 3,000 hours of unplanned downtime prevented annually, thanks to computer vision.
Solution 2. Identifying defects in products, packaging, and labels
Our next story comes from a manufacturer of wood, fiberglass, and vinyl building products. They wanted to improve quality control and reduce downstream issues like reworking products and processing return requests.
To accomplish that, the company deployed a vision AI system that automates quality inspections. Compared to traditional machine vision solutions, their modern AI system can identify nuanced variations in product specifications like color, texture, inaccurate labels, and other slight imperfections.
By automatically detecting these slight quality issues earlier in the production process, this manufacturer minimized the number of defects going out the factory door. As a result, they project at least 60% less return and exchange requests annually.
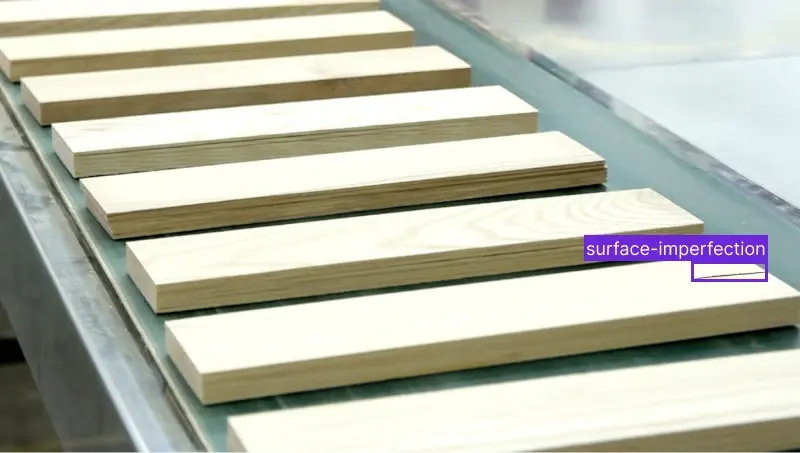
Solution 3. Alerting staff to safety and health hazards
When monitoring the safety of employees, it's crucial to detect hazards and dangerous behavior before an incident occurs. One story that exemplifies this comes from a global manufacturer of industrial equipment.
The company is introducing an AI-powered monitoring system that detects near-collisions between vehicles and humans. In addition to triggering a real-time warning, this kind of visual AI system can identify the exact vehicle and record incidents of near-collisions to a database.
With this AI-powered safety monitoring system, staff can review trends and take proactive steps — like scheduling additional training sessions or altering facility layout — to reduce the possibility of collisions in the future.
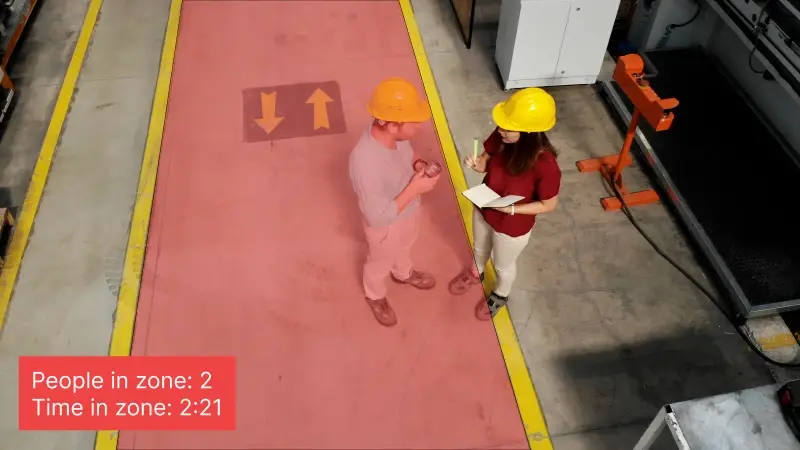
Yielding results with vision AI – now is the time
From minimizing unplanned downtime to enhancing product quality and bolstering workplace safety, the above case studies demonstrate the tangible benefits that manufacturers are realizing through the use of visual AI.
With AI advancing quickly, we expect manufacturers to rethink processes from the ground up. We’re excited to see how it enables entirely new methods and ways to solve complex business challenges in 2025.
Learn more
Check out more ways Roboflow helps you automate and optimize manufacturing processes. Or check out one of our technical guides about developing a defect detection system with vision AI, measuring product dimensions, identifying thermal hotspots during a manufacturing process.
Do you have an AI success story? Let us know.
Would you like to share your manufacturing use case or success story? Send an email to stories@roboflow.com and let us know how you’re measuring the return on investment or seeing success with computer vision.
Cite this Post
Use the following entry to cite this post in your research:
Patrick Deschere. (Jan 31, 2025). AI in Manufacturing: 3 Proven Strategies to Adopt in 2025. Roboflow Blog: https://blog.roboflow.com/ai-in-manufacturing/