The computer vision research community benchmarks new models and enhancements to existing models to test model performance. Benchmarking happens using standard datasets which can be used across models. With this approach, the efficacy of various models can be compared, in general, to show how one model is more or less performant than another.
Common Objects in Context (COCO) is one such example of a benchmarking dataset, used widely throughout the computer vision research community. It even has applications for general practitioners in the field, too.
In this post, we will dive into the COCO dataset, explaining the motivation for the dataset and exploring dataset facts and metrics. Let's start by talking about what the COCO dataset is.
What is the Microsoft COCO Dataset?
The Microsoft Common Objects in Context (COCO) dataset is the gold standard benchmark for evaluating the performance of state of the art computer vision models. COCO contains over 330,000 images, of which more than 200,000 are labelled, across dozens of categories of objects. COCO is a collaborative project maintained by computer vision professionals from numerous prestigious institutions, including Google, Caltech, and Georgia Tech.
Why should I use the COCO Dataset?
The COCO dataset is designed to represent a vast array of things that we regularly encounter in everyday life, from vehicles like bikes to animals like dogs to people.
The COCO dataset contains images from over 80 "object" and 91 generic "stuff" categories, which means the dataset can be used for benchmarking general-purpose models more effectively than small-scale datasets.
In addition, the COCO dataset contains:
- 121,408 images
- 883,331 object annotations
- 80 classes of data
- The median image ratio is 640 x 480
You can use semantic search to query inside COCO to better understand the data or take a look at the mix of classes. We found some weird images in COCO, which served as a good reminder that you should always have an in-depth understanding of your training data.
The COCO dataset is labeled, providing data to train supervised computer vision models that are able to identify the common objects in the dataset.
Label and Annotate Data with Roboflow for free
Use Roboflow to manage datasets, label data, and convert to 26+ formats for using different models. Roboflow is free up to 10,000 images, cloud-based, and easy for teams.
Of course, no computer vision model is perfect by all metrics. The COCO dataset provides a benchmark for evaluating the periodic improvement of these models through computer vision research. Practitioners and researchers can benchmark models to see how they have evolved with changes, allowing the community to chart the growth of specific models over time. Entirely different models can be benchmarked on COCO, too.
For a snapshot of how different real-world computer vision models perform on object identification tasks with COCO, check out the Papers with Code COCO Dataset leaderboard.
A Checkpoint for Transfer Learning
The COCO dataset also provides a base dataset to train computer vision models in a supervised training method. Once the model is trained on the COCO dataset, it can be fine-tuned to learn other tasks, with a custom dataset. Thus, you can think of COCO like a springboard: it will help you build a generic model, and you can customize it with your own data to improve performance for specific tasks.
In the video below, we discuss how to get started with transfer learning from the COCO dataset. This video dives deep into what objects are in the COCO dataset and how well different objects are represented.
What is the COCO dataset used for?
The COCO dataset can be used for multiple computer vision tasks. COCO is commonly used for object detection, semantic segmentation, and keypoint detection. Let's discuss each of these problem types in more detail.
Object Detection with COCO
Objects are annotated with a bounding box and class label. This annotation can be used to identify what is in an image. In the example below, giraffes and cows are identified in a photo of the outdoors.
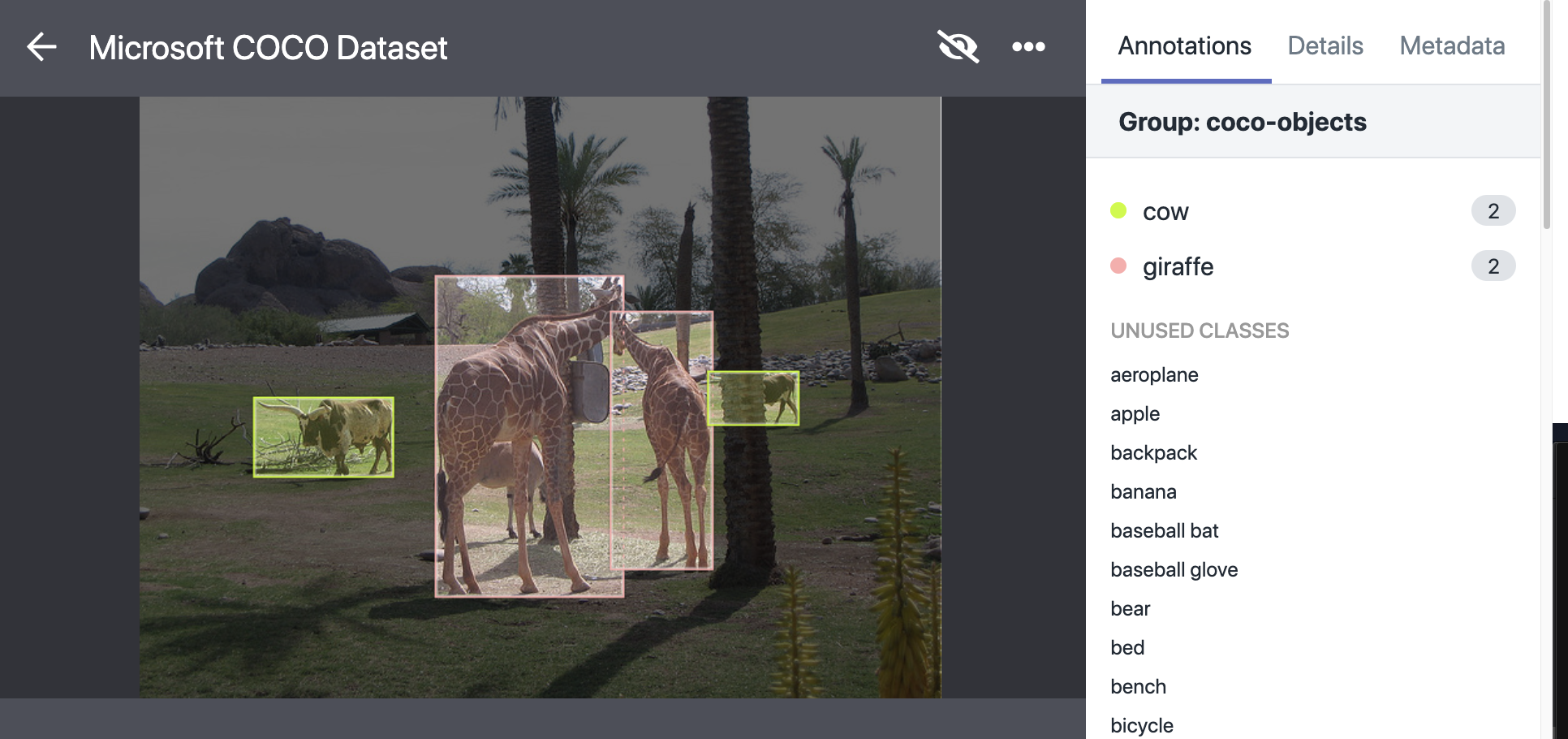
Semantic Segmentation with COCO
In semantic segmentation, the boundary of objects are labeled with a mask and object classes are labeled with a class label. You can use this to identify more exactly where different objects are in a photo or video.
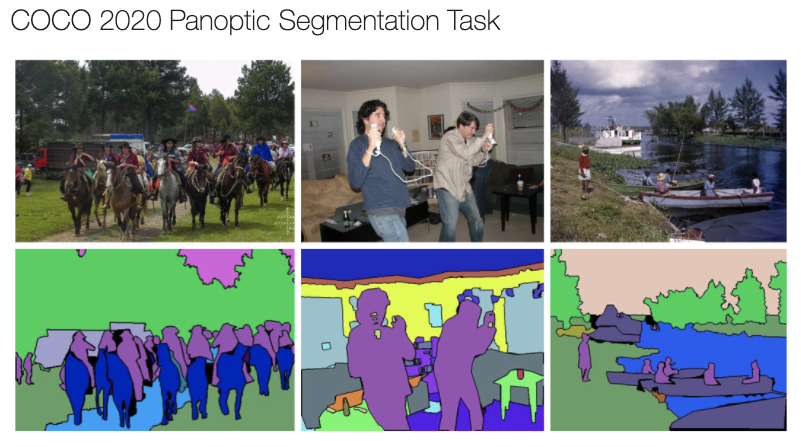
Keypoint Detection with COCO
In keypoint detection, humans are labeled with key points of interest (elbow, knee, etc.). You can then use this to track specific movements such as whether a person is standing or sitting down. COCO contains over 250,000 people with keypoints labelled.
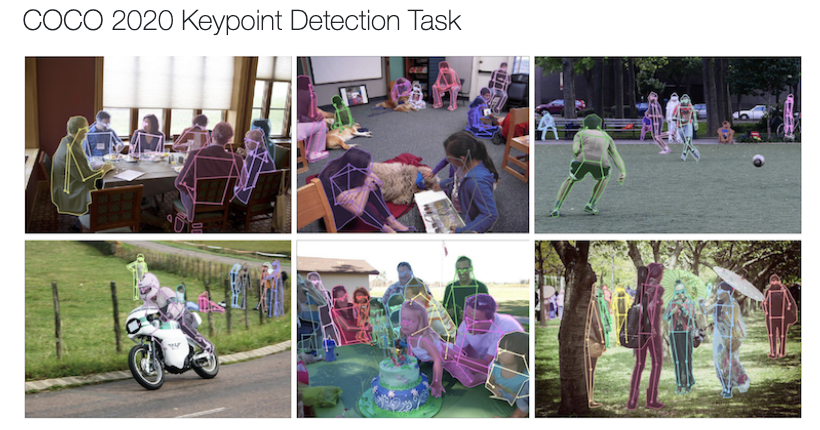
COCO Dataset Class List
Are you curious about what exactly is in the dataset? Here's a breakdown of all of the class labels provided in annotations in the COCO dataset:
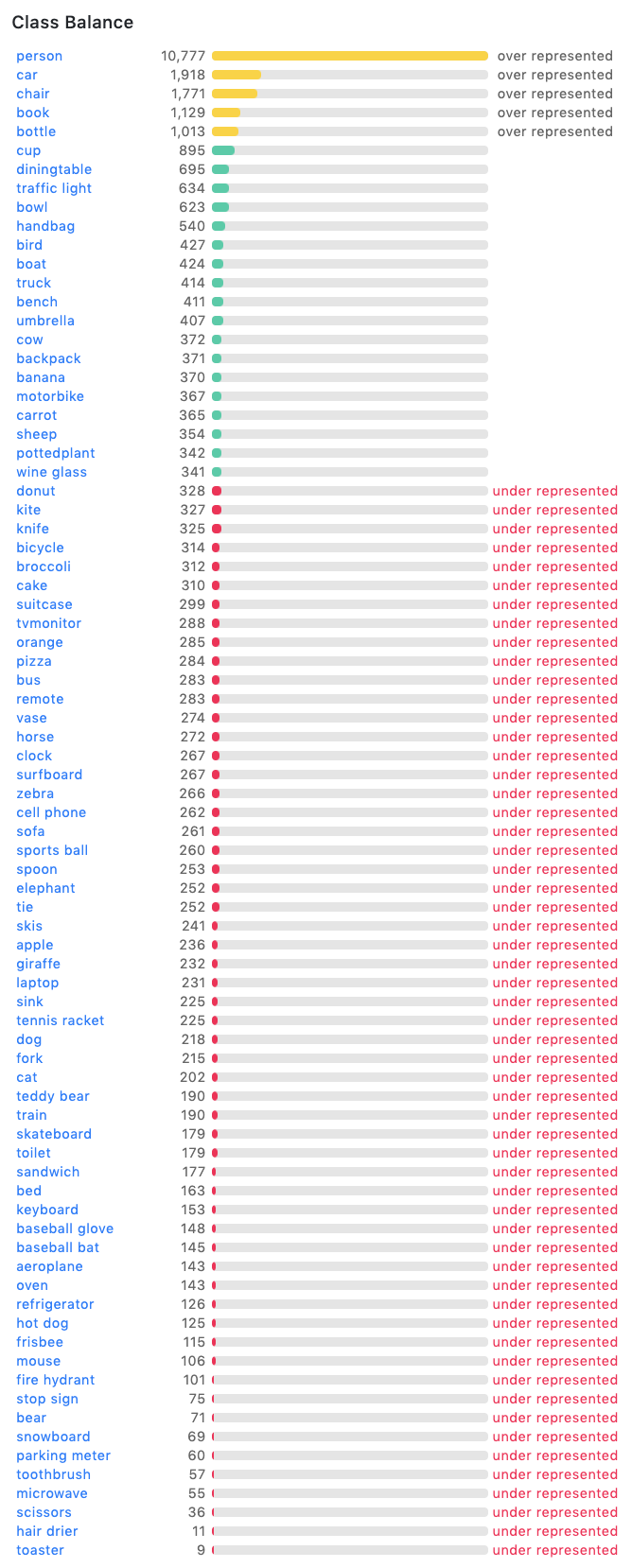
In the COCO dataset class list, we can see that the COCO dataset is heavily biased towards major class categories - such as person, and lightly populated with minor class categories - such as toaster. There are many classes of objects that are annotated hundreds of times in the dataset, from dogs to skateboards to laptops. Some objects are not well represented, such as toasters. There are only 9 annotations corresponding to toasters in the dataset.
It is hard to train models on the COCO dataset to recognize classes that are under exposed. See our balancing classes in object detection video for more information on why this is the case. Thus, COCO is not a one-stop, complete dataset for all computer vision model needs. If an object you want to identify is not in COCO, you will need to add your own data to improve representation of the object.
COCO Dataset Explorer
You can explore the COCO dataset by using the COCO dataset explorer. To use the explore simply visit, the COCO dataset explorer page
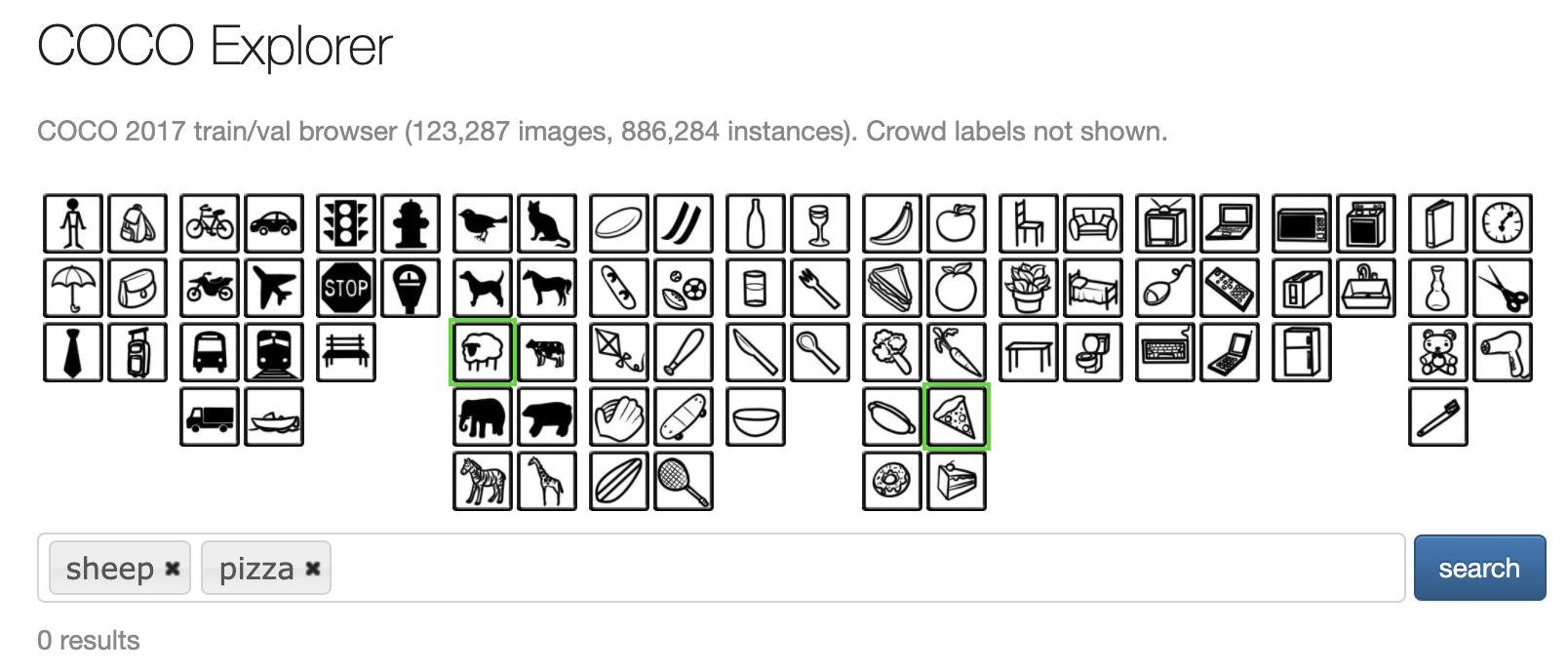
Downloading the COCO Dataset
To download the COCO dataset you can visit the download link on the COCO dataset page. The following Python script downloads the object detection portion of the COCO dataset to your local drive. The script also unzips all of the data so you'll have everything set up for your exploration and training needs.
#!/bin/bash
# COCO 2017 dataset http://cocodataset.org
# Download command: bash data/scripts/get_coco.sh
# Train command: python train.py --data coco.yaml
# Default dataset location is next to /yolov5:
# /parent_folder
# /coco
# /yolov5
# Download/unzip labels
d='../' # unzip directory
url=https://github.com/ultralytics/yolov5/releases/download/v1.0/
f='coco2017labels.zip' # 68 MB
echo 'Downloading' $url$f ' ...' && curl -L $url$f -o $f && unzip -q $f -d $d && rm $f # download, unzip, remove
# Download/unzip images
d='../coco/images' # unzip directory
url=http://images.cocodataset.org/zips/
f1='train2017.zip' # 19G, 118k images
f2='val2017.zip' # 1G, 5k images
f3='test2017.zip' # 7G, 41k images (optional)
for f in $f1 $f2; do
echo 'Downloading' $url$f ' ...' && curl -L $url$f -o $f && unzip -q $f -d $d && rm $f # download, unzip, remove
done
The COCO Dataset Format
The COCO dataset comes down in a special format called COCO JSON. COCO JSON is not widely used outside of the COCO dataset. As a result, if you want to add data to extend COCO in your copy of the dataset, you may need to convert your existing annotations to COCO. Equally, you can also convert COCO data to any one of many formats (i.e. YOLO Darknet TXT) if this is appropriate for your use case.
Roboflow is here to help with this task. Check out our COCO JSON Data Conversion Guide to find out how to convert data to and from the COCO format.
Conclusion
In this article, we have discussed:
- How COCO is a widely-used dataset for benchmarking.
- The amount of data in COCO and the scope of the dataset.
- Common tasks you can do with the dataset.
With this knowledge in mind, you now have all the information you need to start exploring COCO for yourself and making your own projects. If you decide to build your own project with COCO, let us know! As always, happy building.
Frequently Asked Questions
What is the main differentiator of COCO?
The COCO dataset contains many annotated images of different objects in their natural context. For example, a television in a room, or a person playing tennis on a tennis court. This is in contrast to many other datasets where images are focused in specific contexts.
Because COCO contains images in many different contexts, well-trained models trained on the dataset can perform better at identifying images in various environments.
Is COCO used for benchmarking?
Yes, the COCO dataset is used for benchmarking. In fact, there is even a collection of leaderboards on the COCO website where you can see how various computer vision models perform on tasks that use the COCO dataset.