We are excited to release support for zero-shot segmentation labeling in Roboflow Annotate using Meta AI’s Segment Anything Model (SAM).
Using the Smart Polygon feature, you’re accessing a cloud-hosted Segment Anything model enabling you to apply polygon annotations faster, easier, and more accurately than ever before, right inside the Roboflow UI. No setup. No servers. No integrations. Create annotations with one click.
Starting today, all users can access the new SAM powered Smart Polygon feature for free. Log in to give it a try.
Decreasing the time and cost to label data while increasing the accuracy of labels means more accurate models running in production.
This post will show you how to access the new feature and best practices for using it to the fullest extent for your labeling needs. Let’s get started!
Adding Data
You can add data to your Roboflow account in multiple ways. Common methods are drag-and-drop in the Roboflow UI, via API or CLI, and Youtube video upload. Additionally, you can utilize any of the 200k+ open source datasets in Roboflow Universe to clone images (with or without labels) into your account.
Using Smart Polygon with SAM
Once you’re in the labeling interface, click the cursor icon in the right toolbar to enable Smart Polygon and select the free preview of Enhanced.
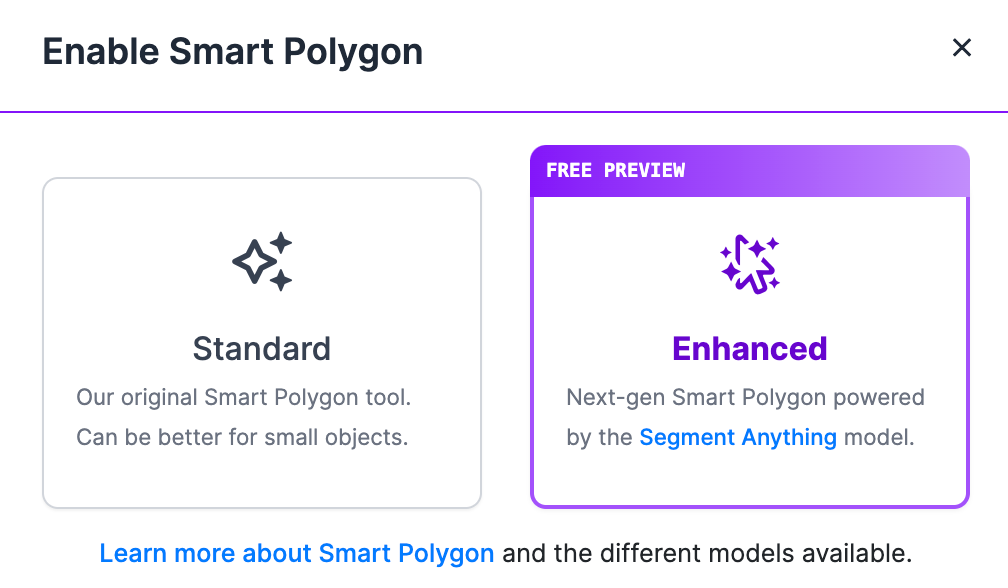
Smart Polygon is now running SAM in the browser. You’ll notice you can hover over objects and see a preview of the mask that will be generated with your initial click. These previews help save time because you can see masks before you apply them and navigate the image to find the best initial mask to create.
When you create an initial mask, you'll be able to select the complexity of your polygon (toggle between them to see the difference!) and then accept the initial mask by pressing Enter.
You can interactively edit the initial mask by clicking outside of the mask to expand the mask, or click inside the mask where it may have included more than your desired object.
For larger objects or objects where masks aren’t created properly in one click, you can click and drag to draw a box around the full object. The method you use depends on your data so experiment with what works best for you.
Convert Bounding Boxes to Polygons
With Smart Polygon, you’re able to convert bounding box annotations into polygon annotations. Right click any bounding box then select Convert to Smart Polygon.
Speed up conversion of bounding boxes to polygons by letting SAM convert all bounding boxes to polygons. This is an option you can use when you know SAM performs well with your custom data.
You can also convert polygons to well fitting bounding boxes using the same right click method.
Tips for Labeling with SAM
We recommend using real world data from your production environment to understand how SAM performs with objects for your specific use case. The interactive Meta AI browser demo has high quality photos which may have objects you’re interested in labeling but your image size or quality could impact how well the model creates annotations in practice.
As we’ve explored labeling with SAM, there are areas to keep in mind when labeling. Fine edges, corners, and small spaces can require more effort to capture. These edge cases are impacted by image size and image quality.
In the below example, the top left image is the full original image. In the top right image, when segmenting the carpet, there are many carpet pixels not captured by SAM.
In the bottom left, you can see SAM struggles to segment the area between fingers. If we take the same image, crop the problematic area, and upload, SAM handles the smaller spaces much better. Take this into consideration when labeling and uploading your data.
For additional speed, you can create multiple individual masks of objects when selecting areas to segment.
Conclusion
SAM is a breakthrough foundation model for computer vision and has broad applicability across domains. Read more about the SAM architecture or see our tutorial on how to use SAM for exploring other ways you can leverage this new model in your next computer vision project.
A great way to familiarize yourself with SAM and ideate on how you can use the model is to experience how it interacts with your data. All Roboflow accounts have access until April 20th so give it a try today for free.
We have more in the works and plan to release many new features to help you build with Roboflow and SAM!