Outsource Labeling is a service offered by Roboflow where customers can work directly with third-party data labelers to annotate their images. Roboflow vets and manages a network of vendors so that customers can seamlessly engage trusted partners to help curate their datasets.
When working with Roboflow’s Outsource Labeling team, providing instructions to labelers is a critical part of the workflow to guarantee the best possible curation of your dataset.
In this article, we have curated best practices to follow which will help ensure that labelers receive a high quality set of instructions to reference throughout their work. Detailed instructions help ensure labelers can meet your expectations given the ontology you have in mind for your project.
Tip #1: Provide Positive Examples
Examples of well annotated images are the most informative way to explain to other labelers how to annotate your data. By providing examples of what the correct outcome should look like, labeling teams have a source of truth that can be referenced at all times.
As a general rule of thumb: the larger that source of truth is, the less confusion or need for communication throughout the labeling process there is.
If you do not have any examples of pre-annotated data, you can label example images in Roboflow Annotate within your project. Here are some examples of well annotated images:
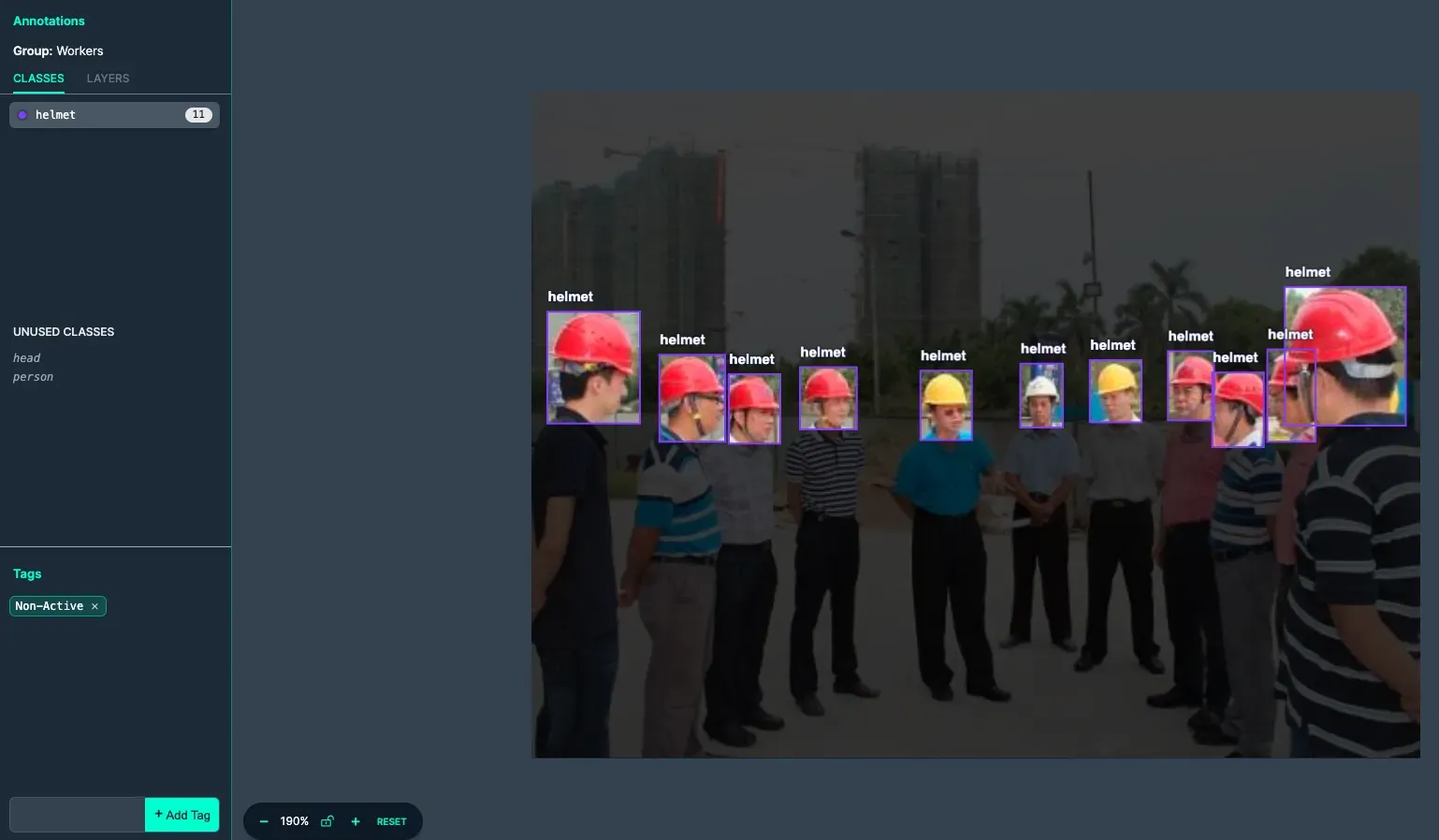
The image above has all its classes labeled properly. The image is also tagged to help with filtering and organization once added to the dataset.
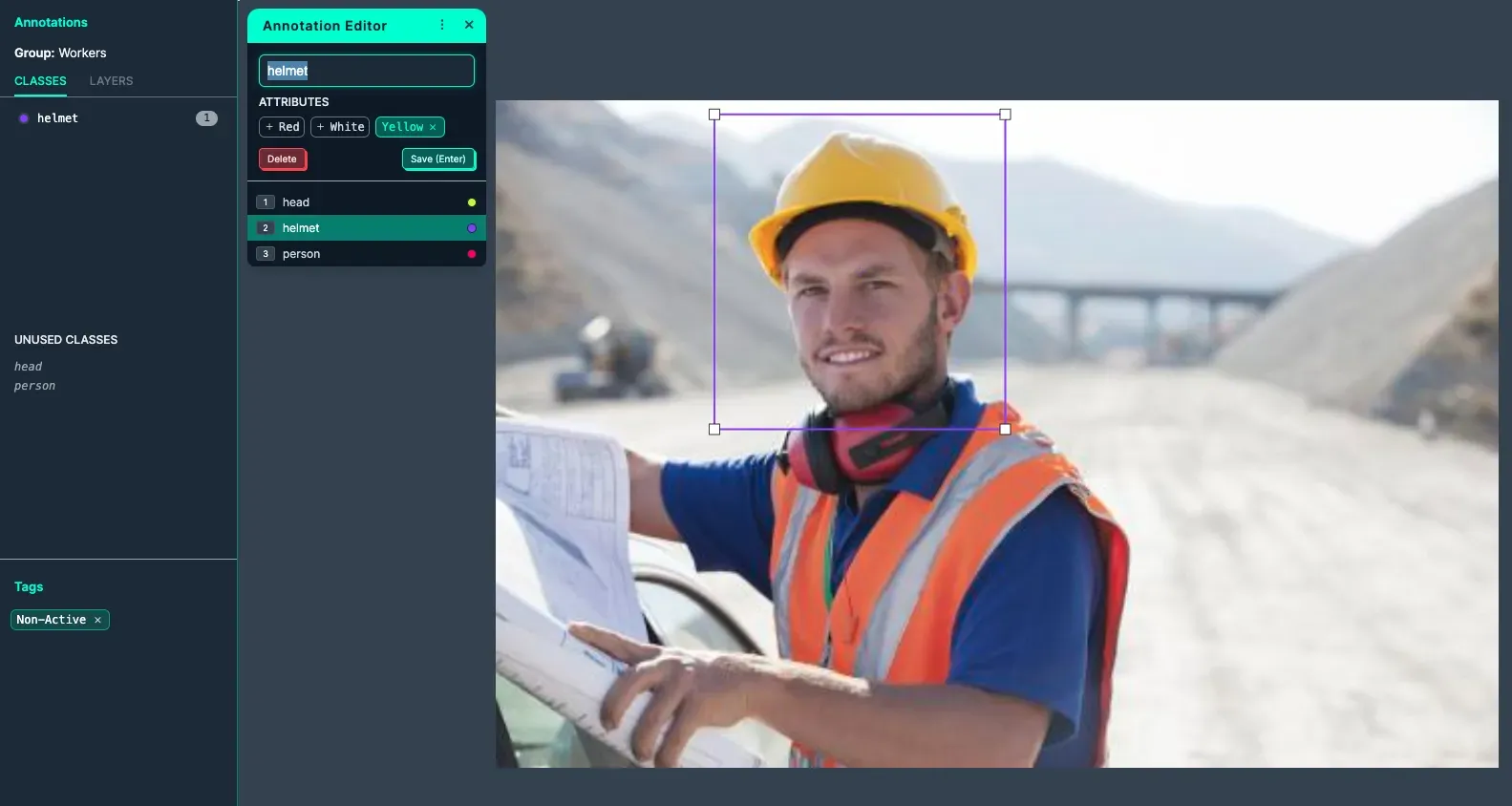
The image above is labeled with an Annotation Attribute to help increase labeling granularity (Yellow) within the class (Helmet). The image is also tagged to help with filtering and organization once added to the dataset.
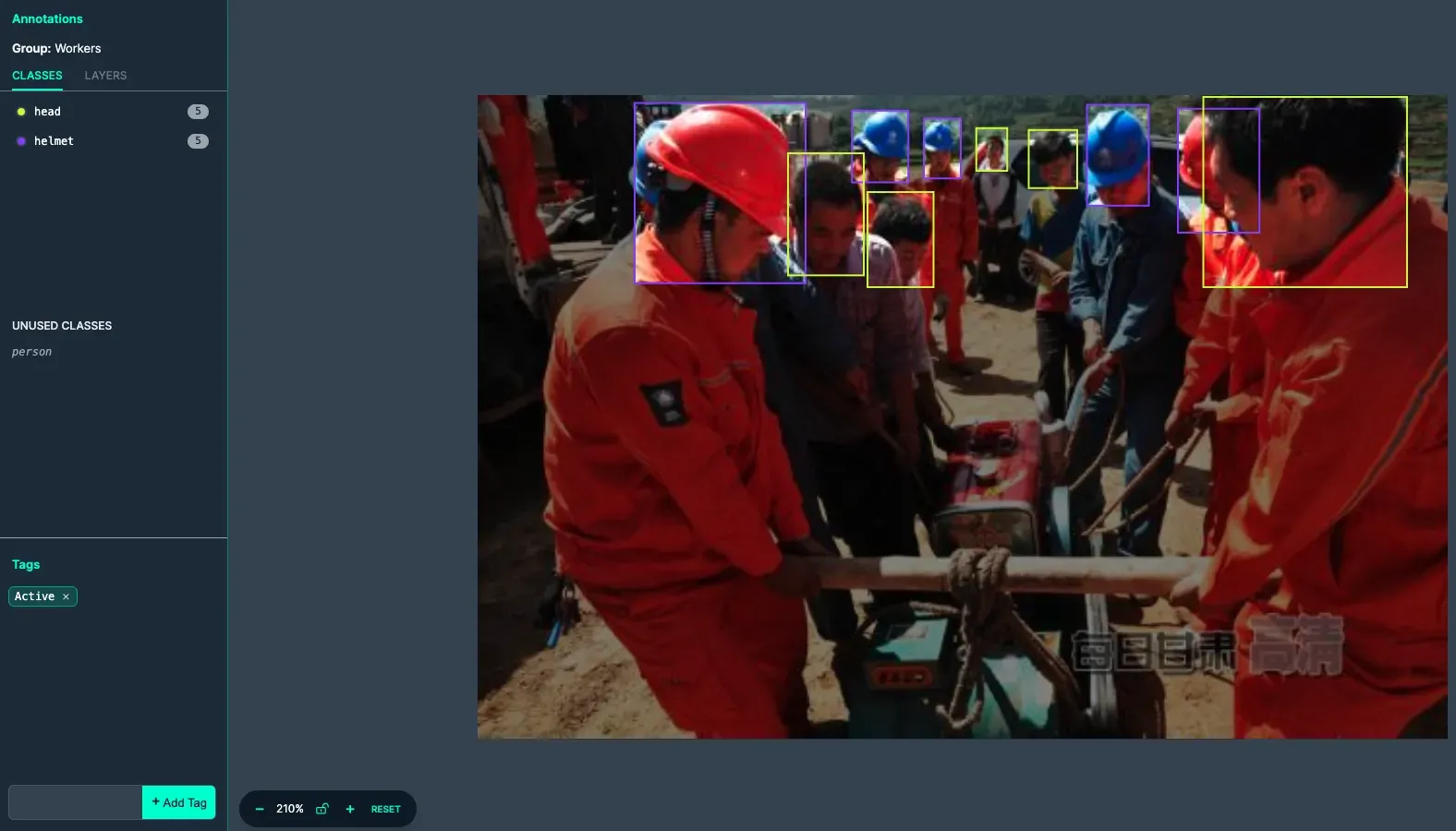
This image includes multiple classes that are all labeled correctly. The image is also tagged to help with filtering and organization once added to the dataset.
Tip #2: Provide Negative Examples
Negative examples, where you feature an image that has been annotated incorrectly, can also help labelers navigate annotation jobs. Negative examples are particularly useful when there is an element of subjectivity to the classes which lends itself to mislabelling. Alternatively, if there are many objects in the image that can get missed, including insufficient examples is also helpful.
Here are some examples of poorly annotated images:
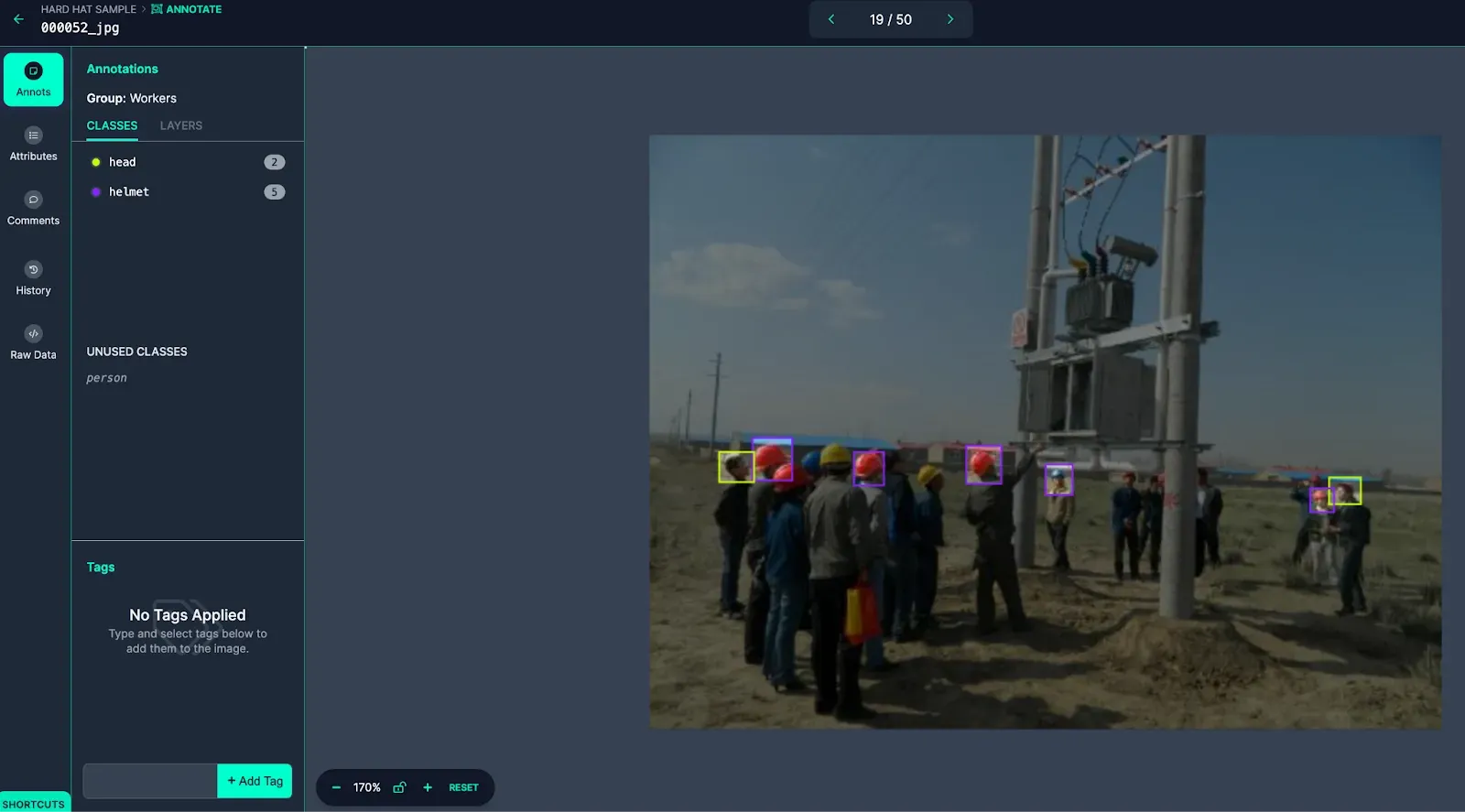
The image above is missing annotations of visible objects, making it an insufficiently labeled image.
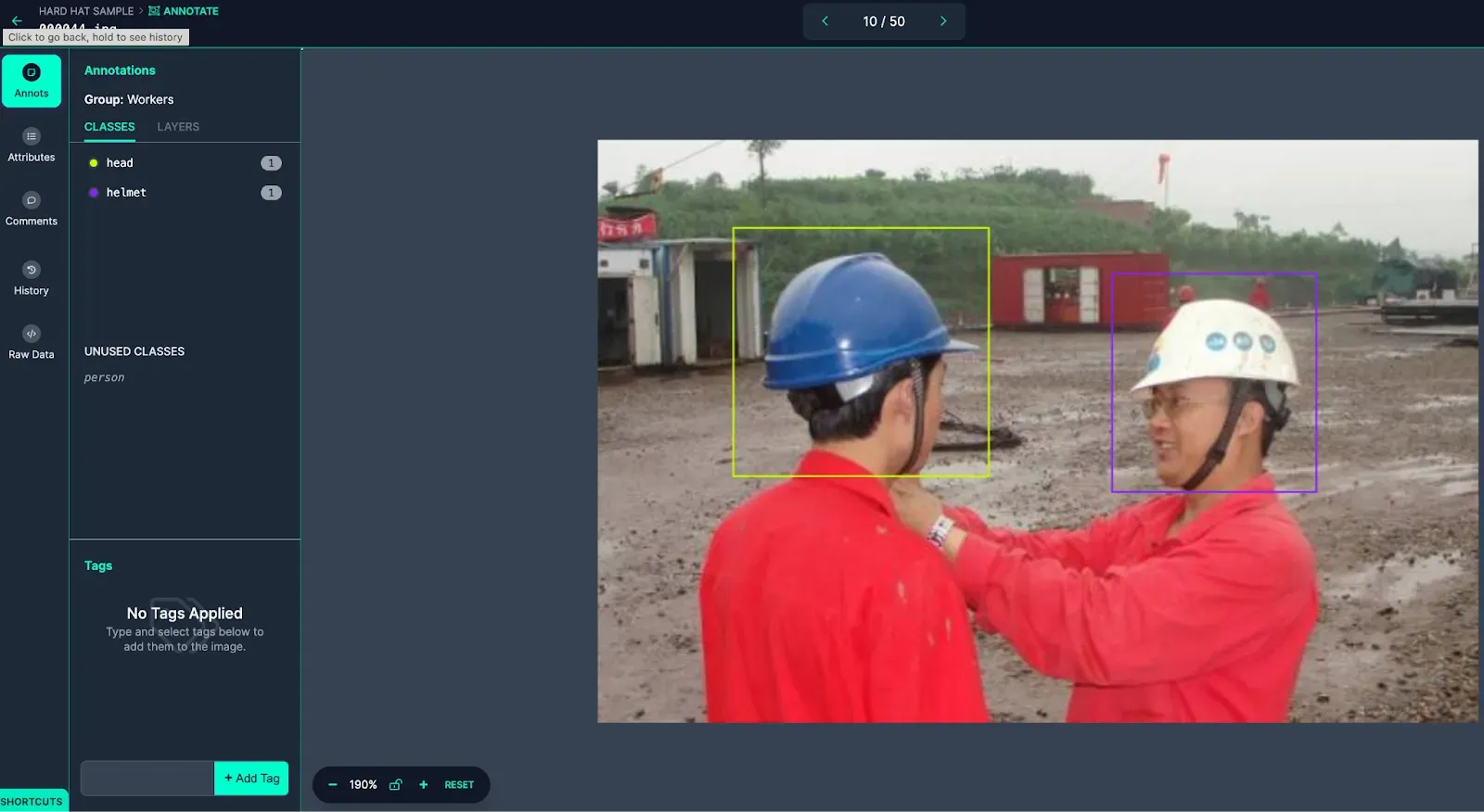
The image above is labeled incorrectly as both people pictured are wearing helmets, despite only one of them being labeled as Helmet. This image would be rejected and not added to the dataset.
Regardless or whether or not the instructions are positive or negative, explaining why they are examples is just as important as the images themselves.
Tip #3: Provide Guidance on Unannotated Images
As you lay the foundation for labeling your data through positive and negative examples, including unannotated images in the instructions can be helpful for labelers to confirm they are properly understanding how the data should be interpreted. If you provide positive and negative examples and labelers still have questions on how to label the unannotated data, that can be a strong signal that the instructions have not been clear enough.
Ultimately, the better a labeler can understand how you view the data, the more successful the labeling process will go. Having unannotated examples can serve as an initial litmus test of the quality of the instructions.
Tip #4: Detail Context for Your Project
By providing labeling instructions, you are teaching labelers how to walk in your shoes when it comes to annotating data. Providing context at a high level regarding the problem you are solving with computer vision can fill in the “why” behind the project.
This allows labelers to not only look at annotations through the lens of what is being annotated but also why it is being annotated. This may spark more informed questions throughout the labeling process or make sure details do not get missed.
Adding project context can be as simple as explaining “we are labeling whether or not workers at construction sites are wearing hard hats to help improve worker safety and reduce workplace injury”.
When tying together all of these pieces of information, the most helpful medium is to compile everything into a shared or downloadable document. Our intake form allows you to share the necessary information with the labelers, whether as a compiled document or as separate pieces of information. When you are ready to begin working with Roboflow’s Outsource Labeling team, fill out the form and we will get back to you within 24 hours.