![What is Instance Segmentation? A Guide. [2025]](/content/images/size/w1200/2023/08/what-is-instance-seg.jpg)
Image segmentation is a type of computer vision project that aims to identify the exact location of an object in an image. With a well-trained image segmentation model, you can calculate "masks" that correspond to the exact contours around an object.
In this guide, we are going to discuss:
- What instance segmentation is
- What output from an instance segmentation model looks like
- How instance segmentation compares to other algorithms
What Is Instance Segmentation?
Instance segmentation, also known as image segmentation, is the computer vision task of recognizing objects in images along with their associated shape. It is an extension of object detection where each prediction also includes a shape as opposed to just a bounding box defined by a center point, width, and height.
With instance segmentation, your application can determine the number of objects in an image, the classifications, and their outline.
It's useful in cases where you need to measure the size of detected objects (eg a tomato leaf), cut them out of their background (eg for background removal), or more accurately detect oblong rotated objects (eg a bridge in a satellite image).
There are many models used for instance segmentation. You can fine-tune a model like YOLOv8 or YOLO11 for real-time image segmentation. If you are interested in learning about fine-tuning segmentation models, we have a few guides for you:
- How to Train YOLOv11 Instance Segmentation on a Custom Dataset
- How to Train YOLOv8 Instance Segmentation on a Custom Dataset
Instance Segmentation Inference Output
After you have trained a model for instance segmentation, your model will predict both bounding boxes and instance segmentation masks around the detected object.
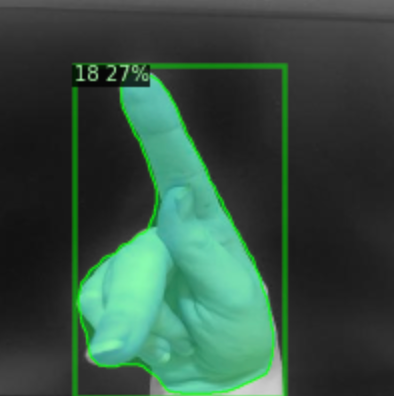
Comparing instance segmentation to other computer vision algorithms
How does instance segmentation compare to other algorithms useful for solving computer vision problems? That's a great question.
Let's compare instance segmentation to both object detection and semantic segmentation so you can build the knowledge you need to better evaluate what model type is best for your use case.
Instance Segmentation vs Object Detection
Both instance segmentation and object detection models identify the position of an object in an image. But, object detection only predicts the object's bounding box, not the outline.
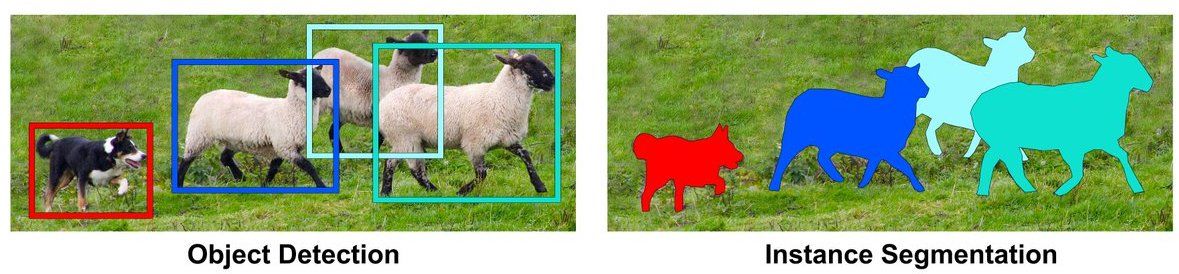
Here are a few things to know about instance segmentation and object detection:
- Instance segmentation labeling is more laborious.
- It is harder to train accurate instance segmentation models.
- Instance segmentation models are typically larger, slower, and less optimized for edge deployment.
- Instance segmentation models may need bigger datasets to obtain the same accuracy as object detection models.
You should only use instance segmentation if the specificity of the object's outline is required by your application. It's often better to try object detection first because it's easier and cheaper to test, and faster and more accurate to run.
Let's consider an example of when these model types are useful. Instance segmentation is better measuring the area of a lawn from satellite imagery. But, if you needed to identify all of the trees on a lawn, object detection would be a more prudent model to use.
Instance Segmentation vs Semantic Segmentation
Semantic segmentation labels each pixel in an image with a class label. Similarly to instance segmentation, you can see the contours of objects in an image, but unlike instance segmentation, you can not count or differentiate between separate objects if the objects are overlapping.
Semantic Segmentation | Instance Segmentation |
---|---|
1. For each pixel in the given image, it detects the object category it belongs to, where all object categories/ labels are known to the model. | 1. For each pixel in the given image, it identifies the object instance it belongs to. It dives deeper than semantic segmentation and differentiates two objects with the same labels. |
2. Example: Semantic segmentation cannot distinguish between different instances in the same category, i.e. all chairs are marked blue. | 2. Example: Instance segmentation can distinguish between different instances of the same categories, i.e. different chairs are distinguished by different colours. |
3. Firstly, target detection takes place, and then each pixel is labelled. | 3. It is a hybrid of annotation of target detection and semantic segmentation. |
4. List of awesome open-source datasets is Stanford Background Dataset, Microsoft COCO Dataset, MSRC Dataset, KITTI Dataset, and Microsoft AirSim Dataset. | 4. List of awesome open-source datasets is LiDAR Bonnetal Dataset, HRSID (High-Dimension SAR Images Dataset), SSDD (SAR Ship Detection Dataset), Pascal SBD Dataset, and iSAID (A Large Scale Aerial Images Dataset). |
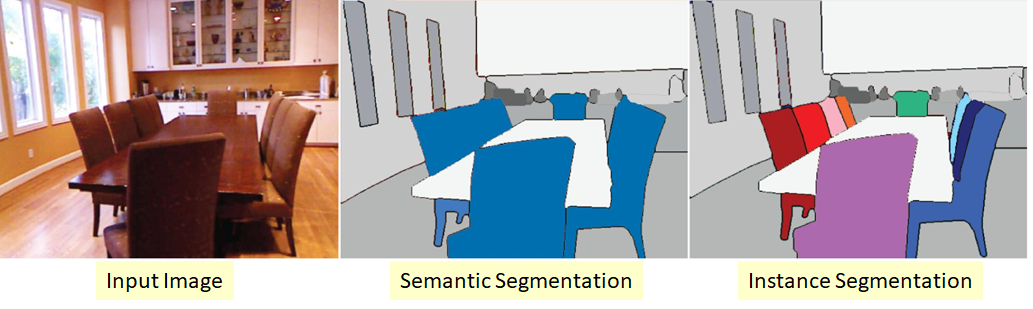
How to Label Images for Instance Segmentation
Images for instance segmentation problems have to be annotated as precisely as possible in an annotation tool. You should draw an outline around the object that you want to annotate and then assign that object a class.
How can you do this annotation? Good news, you can now label images for instance segmentation by drawing polygons within Roboflow Annotate.
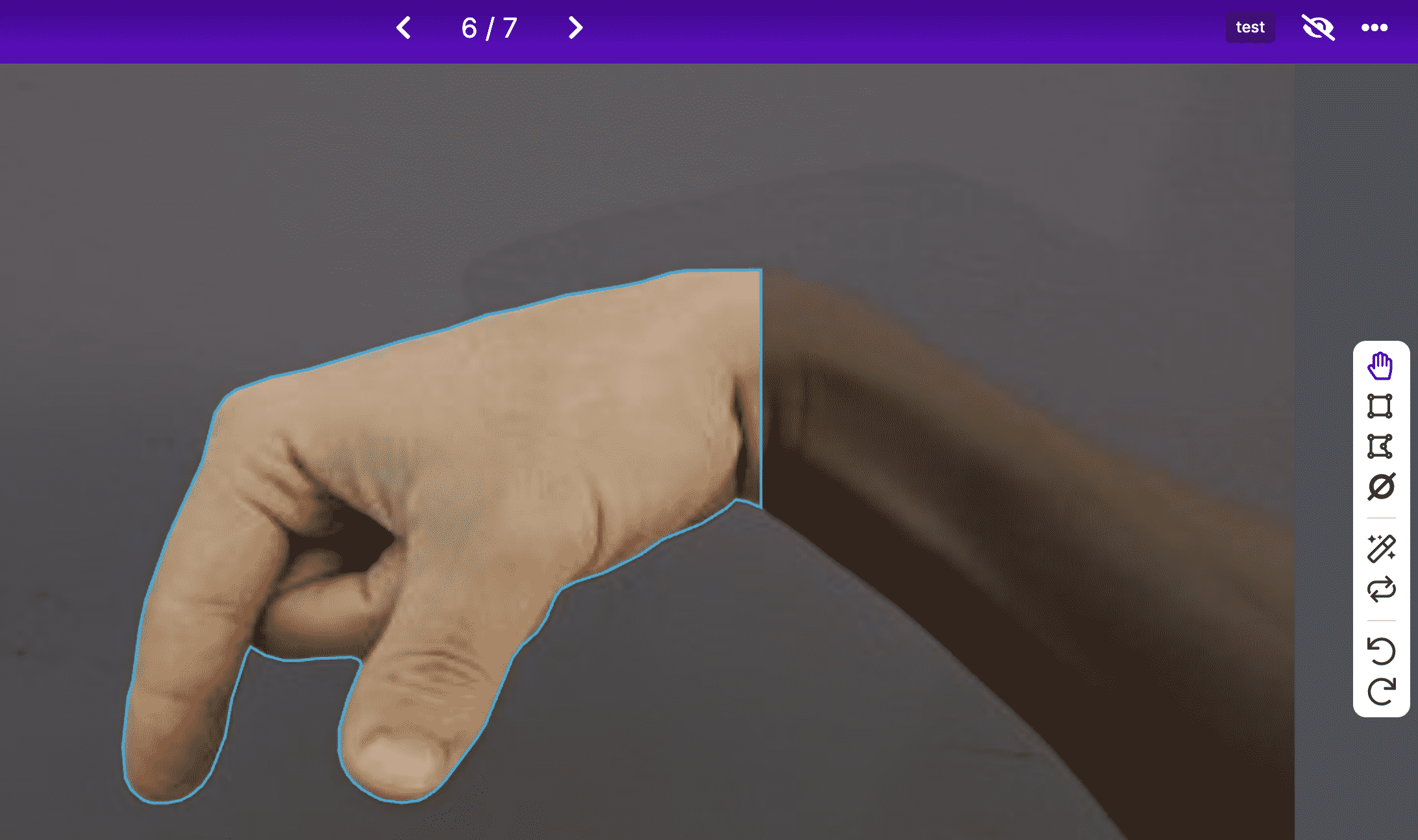
How to Train an Instance Segmentation Model
Once you have labeled your instance segmentation dataset, it is time to train a model to make predictions on images you have not labeled.
Roboflow offers an automated machine learning solution via Roboflow Train for training and deploying instance segmentation models with a single click. Follow that link to see how you can easily train and deploy instance segmentation into production.
If you place to train a model outside of Roboflow, check out our tutorial: How to Train Detectron2 for Instance Segmentation. You'll see how training works when not using an autoML solution.
Instance Segmentation Dataset Format
The most common format for instance segmentation datasets is COCO. The COCO format records the points of the segmentation in a series of x,y pairs.
{
"id": 4,
"image_id": 1,
"category_id": 2,
"bbox": [
273,
451,
98.81642999999997,
119.86870800000003
],
"area": 11844.99779327244,
"segmentation": [
[
294.526515,
451.00638,
273.495847,
518.689734,
372.312277,
570.875088,
295.413657,
452.786753,
294.526515,
451.00638
]
],
"iscrowd": 0
}
You can now import and export your instance segmentation data from Roboflow in the COCO format.
Try Instance Segmentation for Free
We are excited to announce the launch of instance segmentation projects in Roboflow today. The new project type extends Roboflow Annotate's capabilities to polygons so you can detect objects with greater specificity 🚀. Instance segmentation and polygon labeling is included in all of our pricing plans.
To get started, log in to your Roboflow account, create a new project, and choose the Instance Segmentation
project type.
Happy labeling, happy training, and of course, happy inferencing.
Cite this Post
Use the following entry to cite this post in your research:
Jacob Solawetz. (Nov 26, 2024). What is Instance Segmentation? A Guide. [2025]. Roboflow Blog: https://blog.roboflow.com/instance-segmentation/