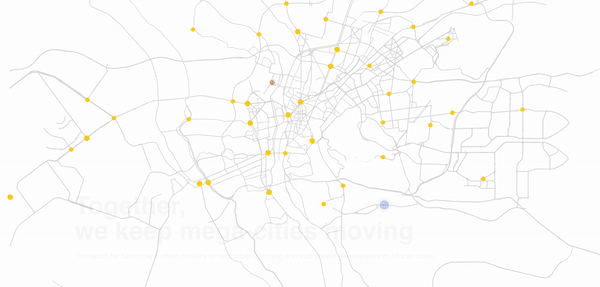
How Transport for Cairo is Improving Commuting for Millions with Computer Vision
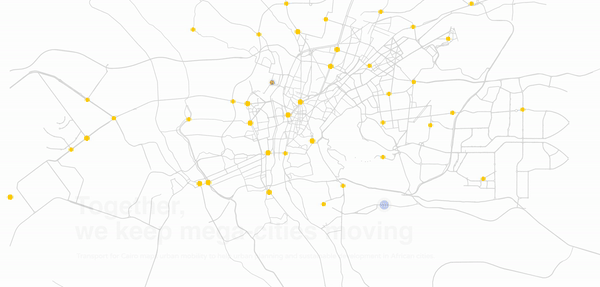
Reducing traffic in well-planned cities where bus routes are well-mapped, subways are running on a predictable cadence, and lanes are well-marked is a significant undertaking.
Now consider improving traffic without predictable routes, maps, and regular modes of transit. It’s a chaotic scene that CityLab paints well:
Come rush hour, the streets of Cairo, Egypt, descend into mayhem. Cars sit in gridlock traffic, horns blaring all around. Some commuters wait at bus stops, unsure when the next one will come. Others escape into Cairo’s subway stations, only to find themselves lost in a sea of people. Then there are the informal microbuses, packed past capacity with passengers—and with more hanging dangerously from the sides.
These are the types of the challenges Transport for Cairo (TfC), a data-driven consultancy that serves primarily African cities, faces in their work everyday. The group exists to help cities reduce congestion while adapting to rapid urbanization -- challenges that they note are at the crux of helping emerging cities grow while adapting to climate change.
Project Overview
A recent TfC project called on the organization to improve infrastructure in a Ugandan city where data is sparse on how traffic flows, where the greatest bottlenecks are, and what types of vehicles commuters use. A mix of taxis, tuk tuks, microbuses, and bikes zip through the city at a dizzying rate. Bus routes are influx and largely to be determined. Even city officials have little data on what patterns look like, further limiting informed decision making.
Without any traffic data to start, TfC’s client suggested the organization turn to hiring human volunteers to stand at various intersections and take tally of how many vehicles they saw in hour segments. Recruiting hundreds of workers to stand at intersections would be expensive, time-consuming, and inexact. Where TfC pursued this manual approach yielded spotty results: it’s challenging to know the validity of the data and staffers could only be paid for specific points in time. A more precise solution was required.
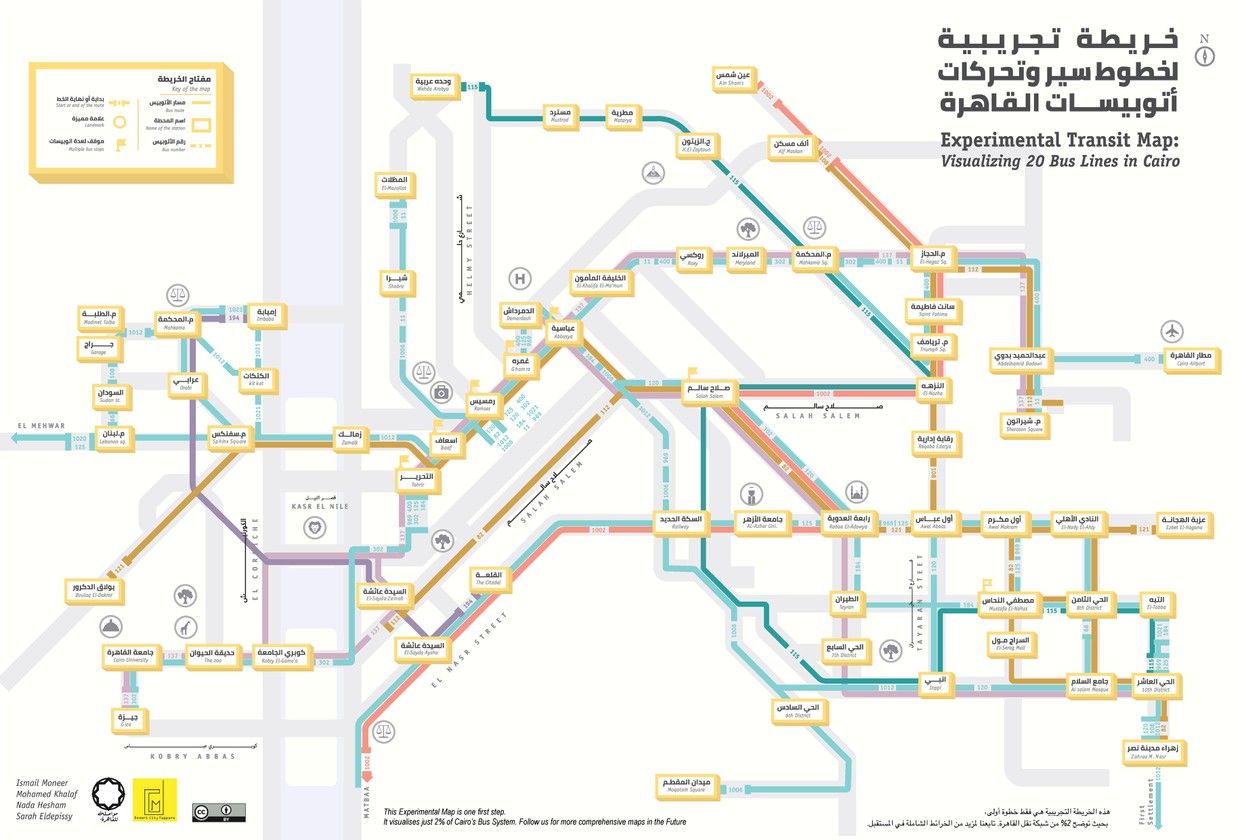
Computer Vision Informed Traffic Flows
Thus, the TfC team set out to use computer vision to more cheaply, reliably, and accurately count commuters in transit. The Ugandan city officials already had closed circuit TV at many intersections of interest. Thus, the TfC team could apply their efforts to processing the data and training a reliable model to count all forms of mobility.
TfC quickly recognized they needed to train a custom model for their problem. Even though ImageNet (and other models) have classes that identify vehicles like cars and trucks, the unique forms of micromobility zipping across the streets require label nuances not captured in pre-trained solutions. What’s more: the model may need to run inference on-device and parse video feeds, requiring fast inference times in a small compute environment.
TfC data scientist Beshoi Maher turned to Roboflow to process labeled data unique to his team’s challenges. Beshoi noted that having access to Roboflow’s dataset health check informed the team’s data collection practices to quickly get ahead of class imbalances that emerged in the data. Moreover, one-click specialized augmentations allowed his team to increase their dataset size, especially of underrepresented classes seamlessly. When it came time to training, the ease with which Beshoi could export his data to any architecture’s required format made testing various models straightforward.
When it came to needing to prep data for custom computer vision models, Roboflow saved the team what could have been another teammate or twice the time for a single model. As TfC continues to incorporate automated vehicle detection for future cities, Roboflow will be a key tool in their arsenal to process images and train more accurate models, faster.
About Transport for Cairo
Transport for Cairo (TfC) provides data, tools and research to improve urban mobility in emerging cities, primarily in Africa. Rapid Urbanization, economic and population growth in times of the climate crisis force us to tackle the complexity and ever-changing urban mobility scene in developing cities. TfC is a disruptive transport consultancy that optimizes existing transport systems and develops flexible and sustainable mobility solutions for our future. https://transportforcairo.com/
Cite this Post
Use the following entry to cite this post in your research:
Joseph Nelson. (Aug 4, 2020). Reducing Traffic with Computer Vision. Roboflow Blog: https://blog.roboflow.com/reducing-traffic-with-computer-vision/
Discuss this Post
If you have any questions about this blog post, start a discussion on the Roboflow Forum.