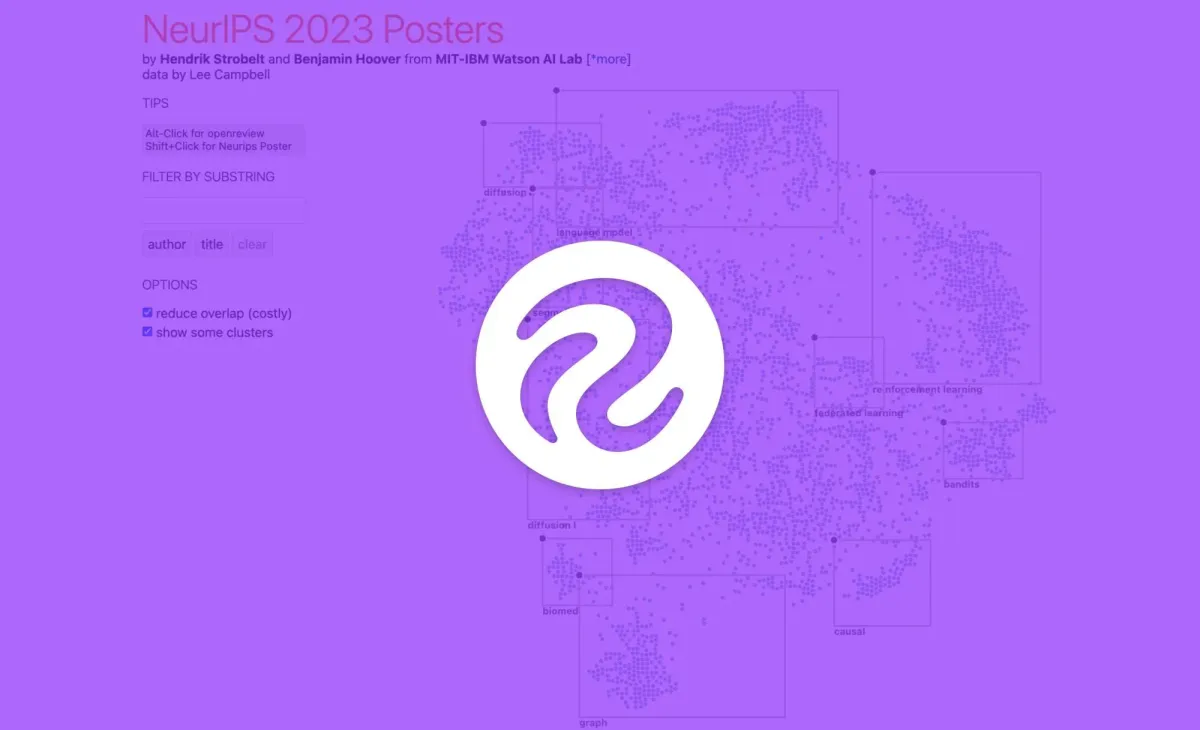
NeurIPS 2023, the conference and workshop on Neural Information Processing Systems, took place December 10th through 16th. The conference showcased the latest in machine learning and artificial intelligence. This year’s conference featured 3,584 papers that advance machine learning across many domains. NeurIPS announced the NeurIPS 2023 award-winning papers to help highlight their view of top research papers.
With the explosion of groundbreaking papers, our team utilized a powerful NeurIPS 2023 papers visualization tool to find the latest advances in computer vision and multimodality from the conference. From there, we read through to find the most impactful papers to share with you.
In this blog post, we highlight 11 important papers from NeurIPS 2023 and share general trends that look to be setting the stage for 2024 and beyond. Let’s begin!
11 Vision and Multimodal Highlights from NeurIPS 2023
Segment Everything Everywhere All at Once
SEEM is a promptable and interactive model for segmenting everything everywhere all at once in an image using a novel decoding mechanism. This mechanism enables diverse prompting for all types of segmentation tasks. The aim is to create a universal segmentation interface that behaves like large language models (LLMs).
Improving Multimodal Datasets with Image Captioning
Massive web datasets play a key role in the success of large vision-language models like CLIP and Flamingo. This study shows how generated captions can increase the utility of web-scraped data points with nondescript text. Through exploring different mixing strategies for raw and generated captions, they outperform the best filtering methods by reducing noisy data without sacrificing data diversity.
What’s Left? Concept Grounding with Logic-Enhanced Foundation Models
Recent works have composed foundation models for visual reasoning—using large language models (LLMs) to produce programs that can be executed by pre-trained vision-language models. However, abstract concepts like “left” can also be grounded in 3D, temporal, and action data, as in moving to your left. This paper proposes Logic-Enhanced FoundaTion Model (LEFT), a unified framework that learns to ground and reason with concepts across domains.
Fine-Grained Visual Prompting
Vision-Language Models (VLMs) have demonstrated impressive zero-shot transfer capabilities in image-level visual perception. However, these models have shown limited performance in instance-level tasks that demand precise localization and recognition.
This paper introduces a new zero-shot framework that leverages pixel-level annotations acquired from a generalist segmentation model for fine-grained visual prompting. Through this research, they reveal an application of blurring outside a target mask that exhibits exceptional effectiveness, called Fine-Grained Visual Prompting (FGVP). This technique demonstrates superior performance in zero-shot comprehension of referring expressions on the RefCOCO, RefCOCO+, and RefCOCOg benchmarks.
Learning to Taste : A Multimodal Wine Dataset
A large multimodal wine dataset for studying the relations between visual perception, language, and flavor.
The paper proposes a low-dimensional concept embedding algorithm that combines human experience with automatic machine similarity kernels. The authors demonstrate that this shared concept embedding space improves upon separate embedding spaces for coarse flavor classification (alcohol percentage, country, grape, price, rating) and aligns with the intricate human perception of flavor.
VisionLLM: Large Language Model is also an Open-Ended Decoder for Vision-Centric Tasks
This team presents an LLM-based framework for vision-centric tasks, termed VisionLLM. This framework provides a unified perspective for vision and language tasks by treating images as a foreign language and aligning vision-centric tasks with language tasks that can be flexibly defined and managed using language instructions. The model can achieve over 60% mAP on COCO, on par with detection-specific models.
Multi-modal Queried Object Detection in the Wild
Multi-modal Queried Object Detection, MQ-Det, is an efficient architecture and pre-training strategy designed to utilize both textual description with open-set generalization and visual exemplars with rich description granularity as category queries, namely, for real-world detection with both open-vocabulary categories and various granularity.
MQ-Det incorporates vision queries into existing well established language-queried-only detectors. MQ-Det significantly improves the state-of-the-art open-set detector GLIP by +7.8% AP on the LVIS benchmark via multimodal queries without any downstream finetuning, and averagely +6.3% AP on 13 few-shot downstream tasks.
LoRA: A Logical Reasoning Augmented Dataset for Visual Question Answering
LoRA is a novel Logical Reasoning Augmented VQA dataset that requires formal and complex description logic reasoning based on a food-and-kitchen knowledge base. 200,000 diverse description logic reasoning questions based on the SROIQ Description Logic were created, along with realistic kitchen scenes and ground truth answers. Zero-shot performance of state-of-the-art large vision-and-language models are then performed on LoRA.
Vocabulary-free Image Classification
Vocabulary-free Image Classification (VIC), aims to assign to an input image a class that resides in an unconstrained language-induced semantic space, without the prerequisite of a known vocabulary. VIC is a challenging task as the semantic space is extremely large, containing millions of concepts, with fine-grained categories.
Category Search from External Databases (CaSED), a method that exploits a pre-trained vision-language model and an external vision language database to address VIC in a training-free manner. Experiments on benchmark datasets validate that CaSED outperforms other complex vision language frameworks, while being efficient with much fewer parameters, paving the way for future research in this direction.
CLIP4HOI: Towards Adapting CLIP for Practical Zero-Shot HOI Detection
Zero-shot Human-Object Interaction (HOI) detection aims to identify both seen and unseen HOI categories. CLIP4HOI is developed on the vision-language model CLIP and avoids the model from overfitting to seen human-object pairs. Humans and objects are independently identified and all feasible human-object pairs are processed by Human-Object interactor for pairwise proposal generation.
Experiments on prevalent benchmarks show that CLIP4HOI outperforms previous approaches on both rare and unseen categories, and sets a series of state-of-the-art records under a variety of zero-shot settings.
Towards In-context Scene Understanding
In-context learning is the ability to configure a model’s behavior with different prompts. This paper provides a mechanism for in-context learning of scene understanding tasks: nearest neighbor retrieval from a prompt of annotated features.
The resulting model, Hummingbird, performs various scene understanding tasks without modification while approaching the performance of specialists that have been fine-tuned for each task. Hummingbird can be configured to perform new tasks much more efficiently than fine-tuned models, raising the possibility of scene understanding in the interactive assistant regime.
Conclusion and Trends
NeurIPS is one of the premier conferences for the fields of machine learning and artificial intelligence. With over 3,000 papers included in the NeurIPS 2023 corpus, there is an overwhelming amount of advancements and breakthroughs which will shape the future of AI in 2024 and beyond.
A few exciting trends emerged this year which we think will continue to grow in importance and enable new computer vision use cases:
- Multimodal model performance: GPT-4 with Vision, Gemini, and many open source multimodal models are pushing model performance into a realm where real enterprise applications can be built using multimodal models. With more teams focusing on improving performance, new benchmarks for understanding performance, and new datasets being created, it looks like 2024 could be the year we have multimodal models as useful as today’s widely adopted language models.
- Prompting for multimodality: LLMs took off in 2023 thanks to the relatively straightforward way of interacting with them using text and it looks like multimodal models are next. Visual data is complex and finding ways to engage with that data in a way to get the results you want is a new frontier being explored. As more interactions are developed, more real world use cases will be unlocked. You can use the open source repo, Maestro, to test out prompting methods.
- Visual logic and reasoning: 2023 had many breakthroughs in segmenting and understanding objects within an image. With those problems fairly solved, moving on to understanding the relationship between objects and how their interactions communicate information is the next step. Much like how LLMs can reason and improve outputs by understanding context, multimodal models will become more useful when they better understand the context of a given scene.
We are thankful to everyone who worked to submit papers for NeurIPS 2023 and excited to help developers turn this research into real-world applications in 2024!
Cite this Post
Use the following entry to cite this post in your research:
Trevor Lynn. (Dec 21, 2023). NeurIPS 2023 Papers Highlights. Roboflow Blog: https://blog.roboflow.com/neurips-2023-papers-highlights/