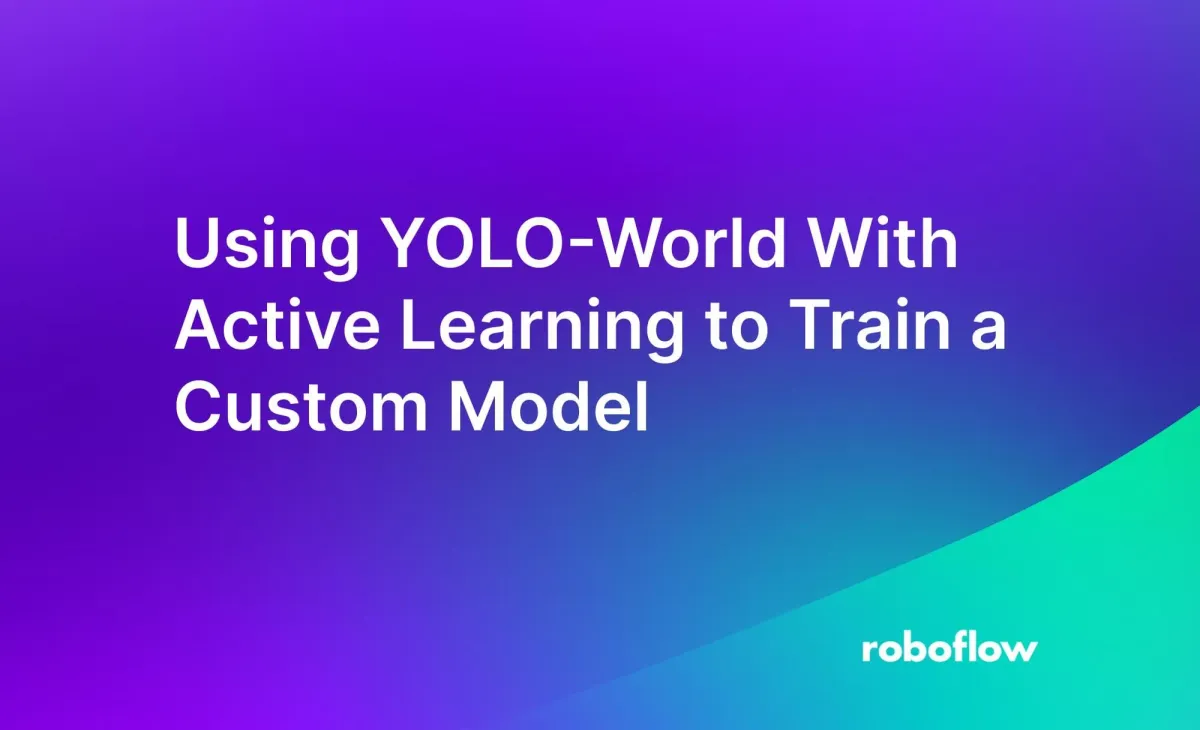
Large vision models like YOLO-World, a zero-shot open-vocabulary object detection model by Tencent’s AI Lab, have shown impressive performance. While zero-shot detection models can detect objects with increasing accuracy, smaller custom models have proven to be faster and more compute-efficient than large models while being more accurate in specific domains and use cases.
Despite those tradeoffs, there’s an important benefit to large, zero-shot models: they require no data labeling or model training to use in production.
In this guide, we demonstrate an approach to applying the benefits of YOLO-World while using active learning for data collection to train a custom model. To do this, we will:
- Deploy YOLO-World using Inference
- Integrate active learning with our deployment
- Train a custom model using our automatically labeled data
Step 1: Deploy YOLO-World
In a few steps, we can set up YOLO-World to run on Roboflow Inference. First, we will install our required packages:
pip install -q inference-gpu[yolo-world]==0.9.12rc1
Then, we will import YOLO-World from Roboflow Inference, where we will set up the classes that we want to detect:
from inference.models.yolo_world.yolo_world import YOLOWorld
model = YOLOWorld(model_id="yolo_world/l") # There are multiple different sizes: s, m and l.
classes = ["book"] # Change this to whatever you want to detect
model.set_classes(classes)
Next, we can run an inference on a sample image and see the model in action:
import supervision as sv
import cv2
image = cv2.imread(IMAGE_PATH)
results = model.infer(image, confidence=0.2) # Infer several times and play around with this confidence threshold
detections = sv.Detections.from_inference(results)
Using Supervision, we can visualize the predictions:
labels = [
f"{classes[class_id]} {confidence:0.3f}"
for class_id, confidence
in zip(detections.class_id, detections.confidence)
]
annotated_image = image.copy()
annotated_image = sv.BoundingBoxAnnotator().annotate(annotated_image, detections)
annotated_image = sv.LabelAnnotator().annotate(annotated_image, detections, labels=labels)
sv.plot_image(annotated_image)
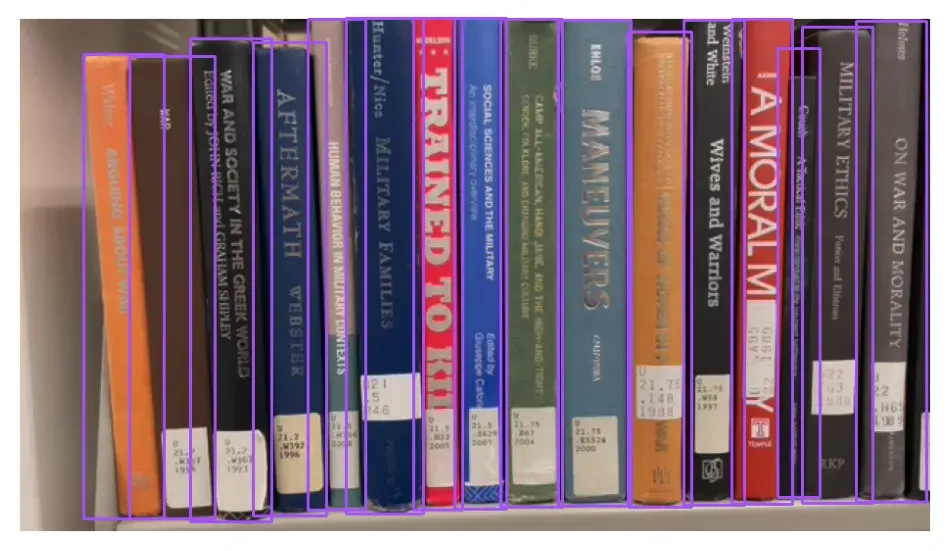
Now we can start running predictions with YOLO-World, and with a bit of extra code, we can start to create a model that’s faster, more efficient, and more accurate at the same time.
Step 2: Active Learning
First, to have a place to store our data, we will create a Roboflow project for our book detection model.
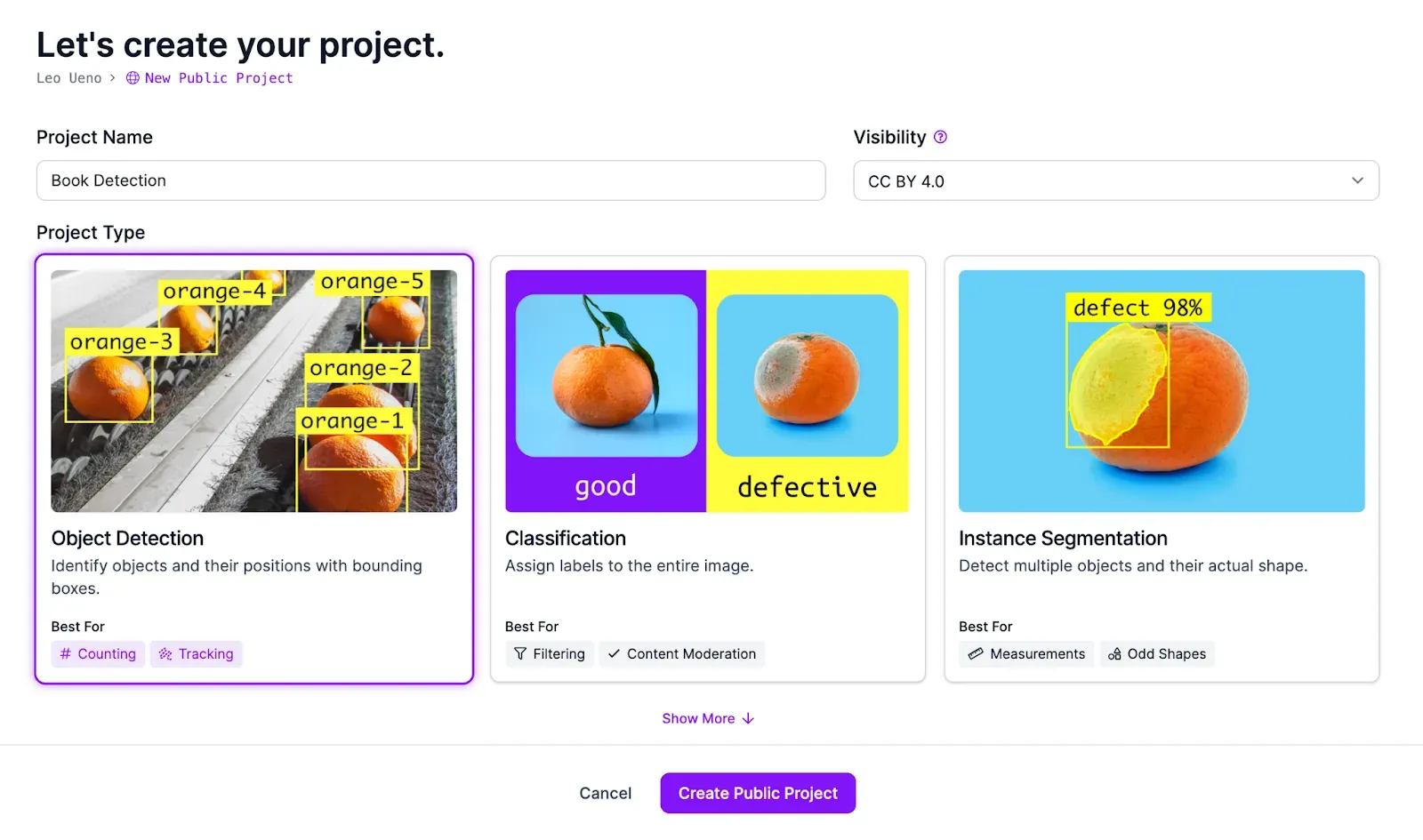
Once that’s done, we can access our workspace with the Roboflow API using Python. First, let’s install the Roboflow Python SDK:
pip install roboflow
Then, we can access our project by using our API key and entering our workspace and project ID.
from roboflow import Roboflow
rf = Roboflow(api_key="YOUR_ROBOFLOW_API_KEY")
project = rf.workspace("*workspace_id*").project("*project_id*")
After that, we have all we need to combine all the previous parts of this guide to create a function that we can use to predict with YOLO-World, while adding those predictions to a dataset so we can train a custom model.
from inference.models.yolo_world.yolo_world import YOLOWorld
import supervision as sv
import cv2
from roboflow import Roboflow
# Project Setup (Copied From Previous Section)
rf = Roboflow(api_key="YOUR_ROBOFLOW_API_KEY")
project = rf.workspace("*workspace_id*").project("*project_id*")
# Model Setup (Copied From Previous Section)
model = YOLOWorld(model_id="yolo_world/l") # There are multiple different sizes: s, m and l.
classes = ["book"] # Change this to whatever you want to detect
model.set_classes(classes)
def infer(image):
results = model.infer(image, confidence=0.1)
detections = sv.Detections.from_inference(results)
image_id = str(uuid.uuid4())
image_path = image_id+".jpg"
dataset = sv.DetectionDataset(classes=classes,images={image_path:image},annotations={image_path:detections})
dataset.as_pascal_voc("dataset_upload/images","dataset_upload/annotations")
project.upload(
image_path=f"dataset_upload/images/{image_path}",
annotation_path=f"dataset_upload/annotations/{image_id}.xml",
batch_name="Bookshelf Active Learning",
is_prediction=True
)
return detections
You can change the infer function to suit your deployment needs. For our example, we plan to run detections on a video, and we don’t want nor need every single frame to be uploaded, so we will modify our infer function to upload randomly 25% of the time:
def infer(image):
results = model.infer(image, confidence=0.1)
detections = sv.Detections.from_inference(results)
if random.random() < 0.25:
print("Adding image to dataset")
image_id = str(uuid.uuid4())
image_path = image_id+".jpg"
dataset = sv.DetectionDataset(classes=classes,images={image_path:image},annotations={image_path:detections})
dataset.as_pascal_voc("dataset_upload/images","dataset_upload/annotations")
project.upload(
image_path=f"dataset_upload/images/{image_path}",
annotation_path=f"dataset_upload/annotations/{image_id}.xml",
batch_name="Bookshelf Active Learning",
is_prediction=True
)
return detections
Using Supervision and the following code, we will run inferences against a video of a library:
def process_frame(frame, i):
print(i)
detections = infer(frame)
annotated_image = frame.copy()
annotated_image = sv.BoundingBoxAnnotator().annotate(annotated_image, detections)
return annotated_image
sv.process_video("library480.mov","library_processed.mp4",process_frame)
A video of visualized predictions from YOLO-World
Then, as you run inferences, you will see images being added to your Roboflow project.
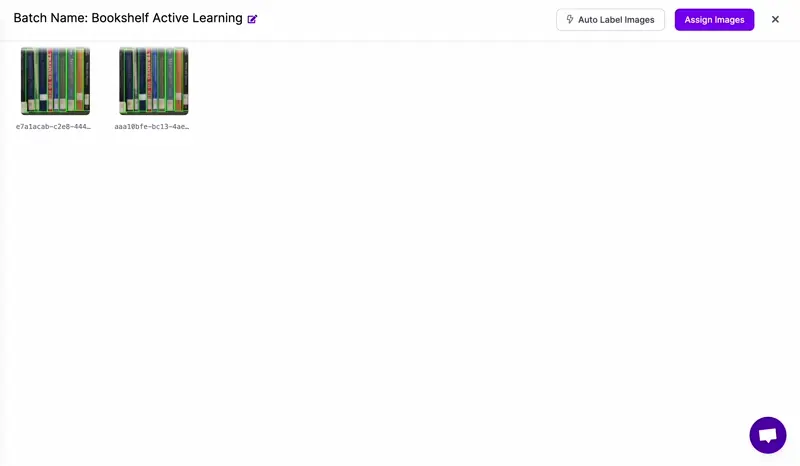
Once uploaded, you can review and correct annotations as necessary, then create a new version to start training your custom model!
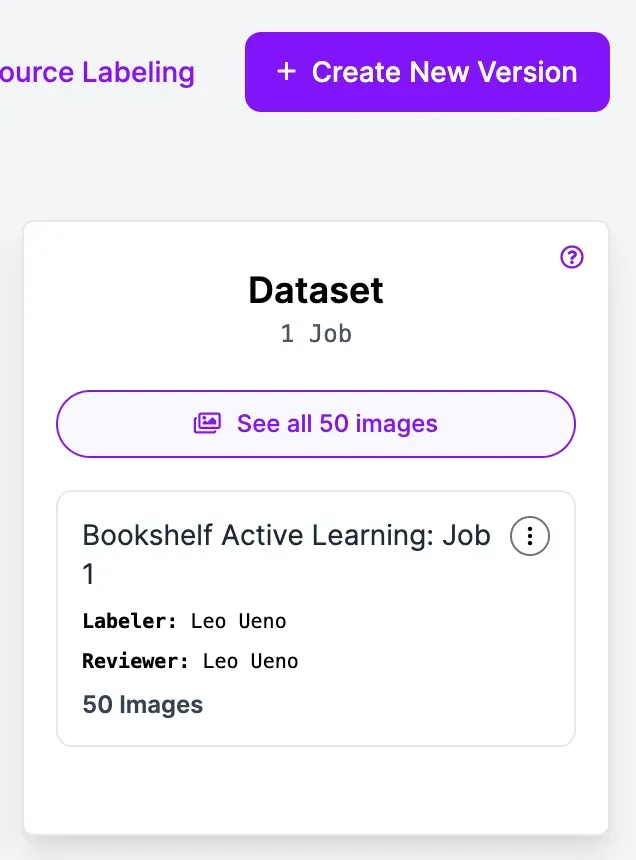
After training, we were able to train a custom model with around 98.3% mean average precision (mAP).

As you continue using your model, whether that be a custom model or a large model like YOLO-World, you can continue collecting data to further improve our models in the future.
Conclusion
In this guide, we were able to combine the best of both worlds: Using a state-of-the-art zero-shot object detection model for immediate use and then using that deployed model to train a custom model with better performance without spending time collecting and labeling a dataset.
After you create your custom model, you can keep improving your models through built-in active learning features in the Roboflow platform.
Cite this Post
Use the following entry to cite this post in your research:
Leo Ueno. (Feb 29, 2024). How to Use YOLO-World With Active Learning to Train a Custom Model. Roboflow Blog: https://blog.roboflow.com/using-yolo-world-with-active-learning-to-train-a-custom-model/