Roboflow provides everything you need to label, train, and deploy computer vision solutions.
You can use the information you learn in this guide to build a computer vision model for your own use case.
As you get started, reference our Community Forum if you have feedback, suggestions, or questions.
We have two examples:
- A quickstart video you can follow in six minutes where we show identifying several objects with a vision model, and;
- Our written example which walks through training a model to identify screws, showing how vision could be used as part of a quality assurance system (i.e. ensuring the number of screws is correct in an assembled kit).
You can follow whatever tutorial you want, but the fundamentals are the same.
Quickstart Tutorial (6 Minutes)
Adding Data
To get started, create a free Roboflow account.
After reviewing and accepting the terms of service, you will be asked to choose between one of two plans: the Public Plan and the Starter Plan.
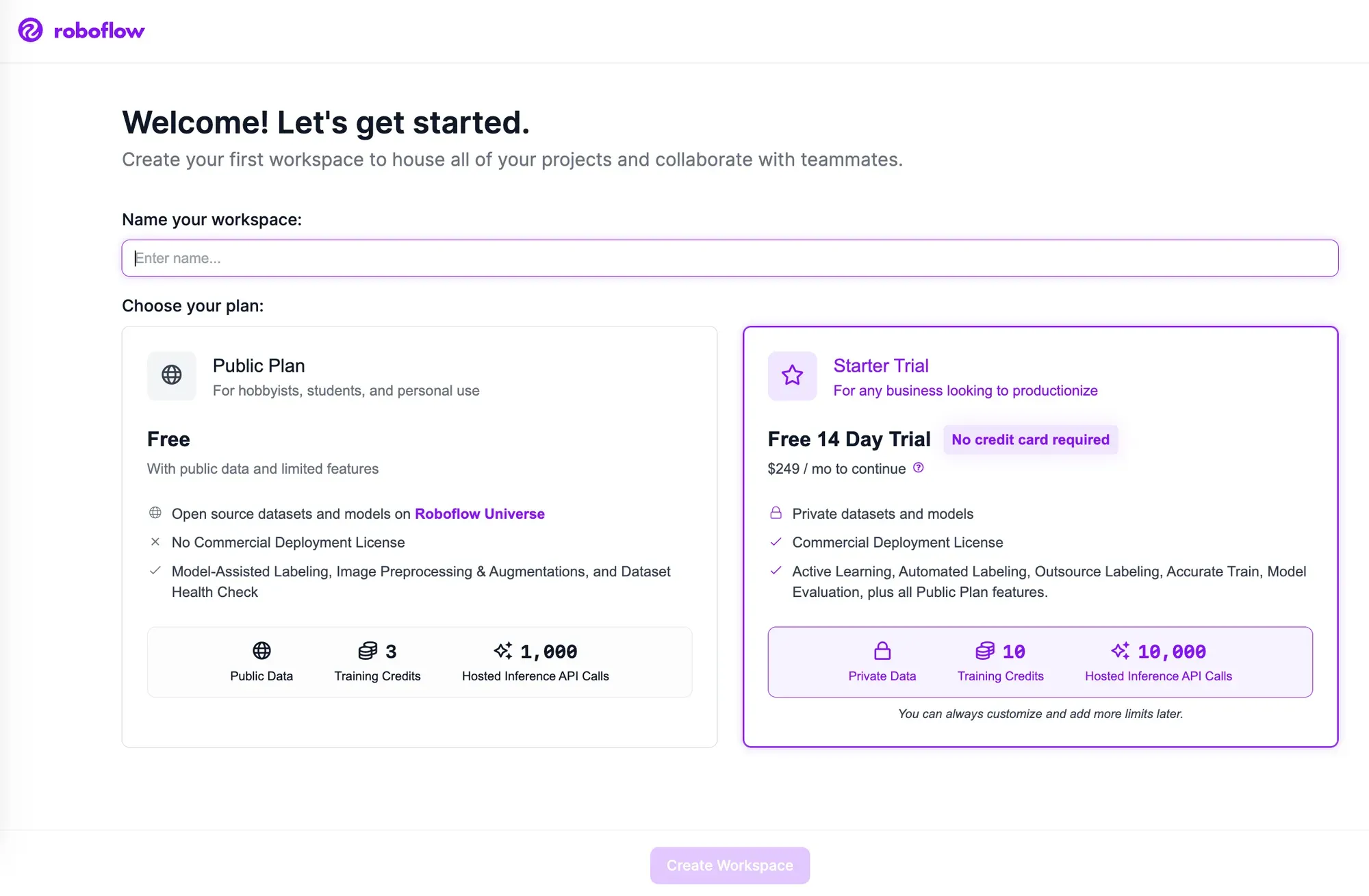
Then, you will be asked to invite collaborators to your workspace. These collaborators can help you annotate images or manage the vision projects in your workspace.
Once you have invited people to your workspace (if you want to), you will be able to create a project.
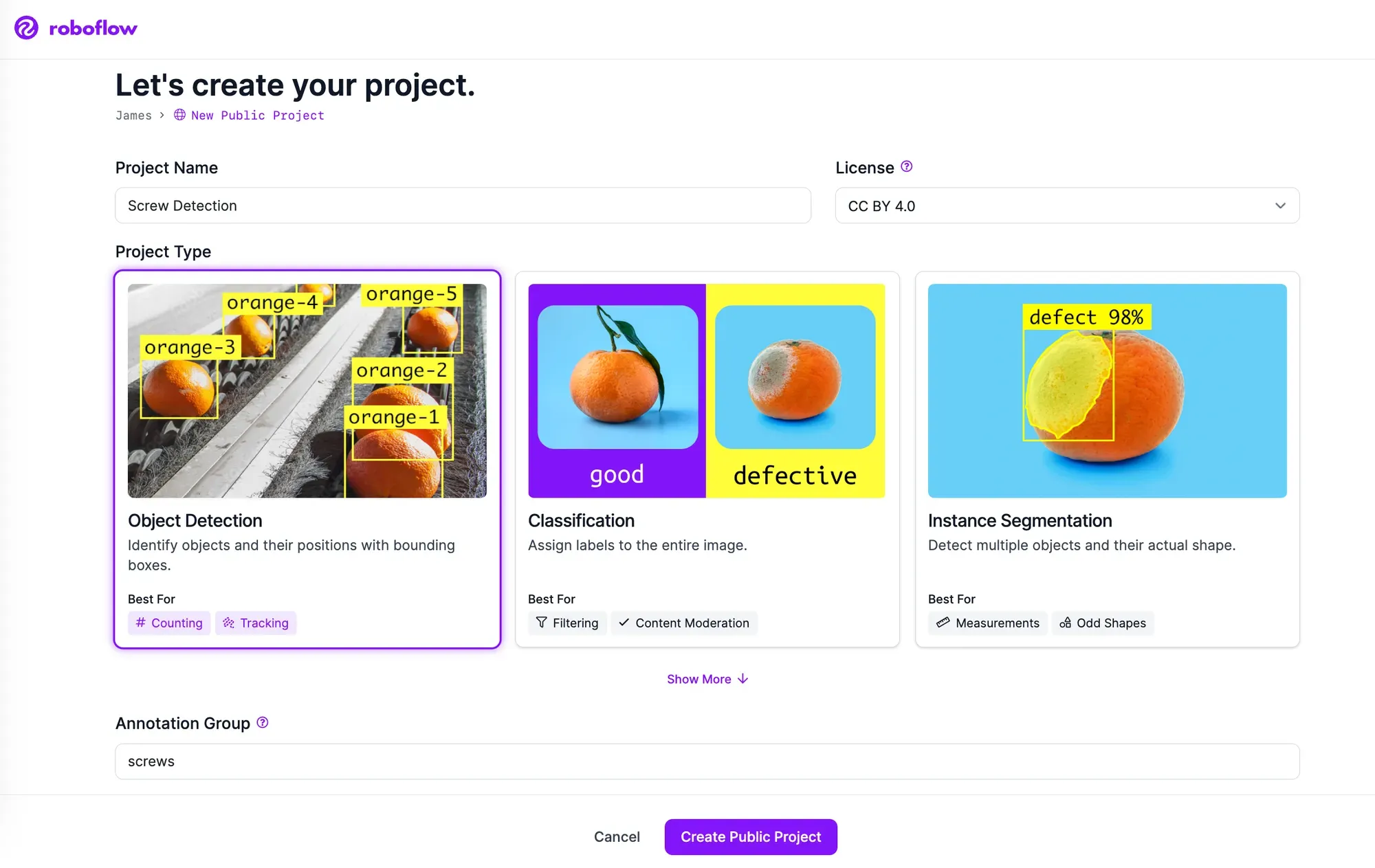
For this example, we will be using a dataset of screws to train a model that can identify screws in a kit. This model could be used for quality assurance in a manufacturing facility. With that said, you can use any images you want to train a model.
Leave the project type as the default "Object Detection" option since our model will be identifying specific objects and we want to know their location within the image.
Click “Create Project.” to continue.
For this walkthrough, we’ll use a Roboflow provided sample screws dataset. Download the screw dataset.
Once you have downloaded the dataset, unzip the file. Click and drag the folder called screw-dataset
from your local machine onto the highlighted upload area. This dataset is structured in the COCO JSON format, one of 40+ computer vision formats Roboflow supports.
We recommend starting with at least 5-10 images that contain a balance of classes you want to identify. For example, if you want to identify two different types of an object – a screw and a nut – you would want to have at least 5-10 images that show both of those objects.
Once you drop the screw-dataset
folder into Roboflow, the images and annotations are processed for you to see them overlayed.
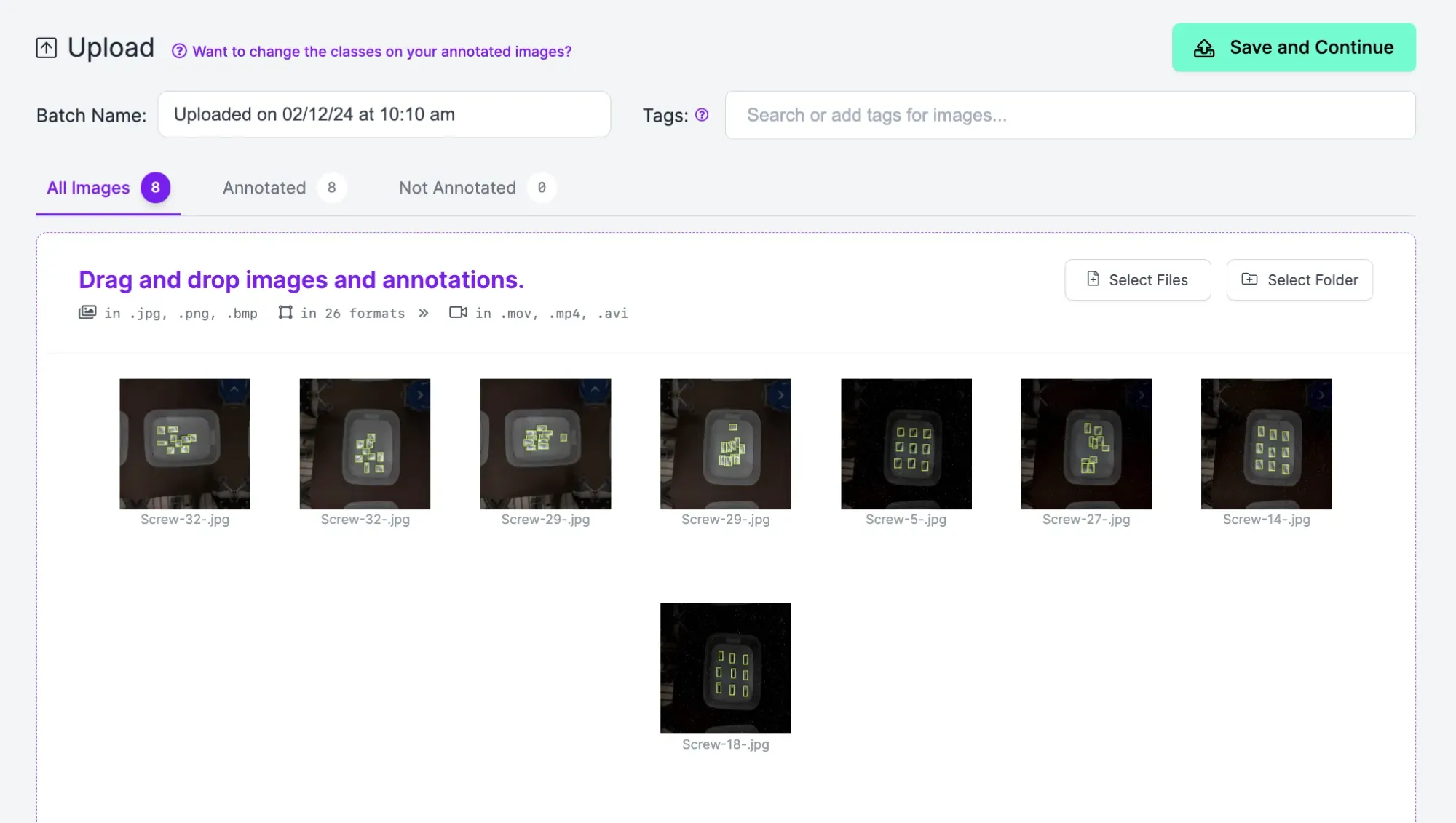
If any of your annotations have errors, Roboflow alerts you. For example, if some of the annotations improperly extended beyond the frame of an image, Roboflow intelligently crops the edge of the annotation to line up with the edge of the image and drops erroneous annotations that lie fully outside the image frame.
At this point, our images have not yet been uploaded to Roboflow. We can verify that all the images are, indeed, the ones we want to include in our dataset and that our annotations are being parsed properly. Any image can be deleted upon mousing over it and selecting the trash icon.
Note that one of our images is marked as "Not Annotated" on the dashboard. We'll annotate this image in the next section.
Everything now looks good. Click “Save and Continue” in the upper right-hand corner to upload your data.
You will be asked to choose a dataset split. This refers to how images will be split between three sets: Train, Test, and Valid.
Your train set contains the images that will be used to train your model. Your valid set will be used during training to validate performance of your model. Your test set contains images you can use to manually test the performance of your model. Learn more about image dataset splits and why to use them.
You can set your own custom splits with Roboflow or, if one is available, use an existing split in a dataset. Our dataset has already been split with Roboflow, so we can choose the "Use existing values" option when asked how to split our images:
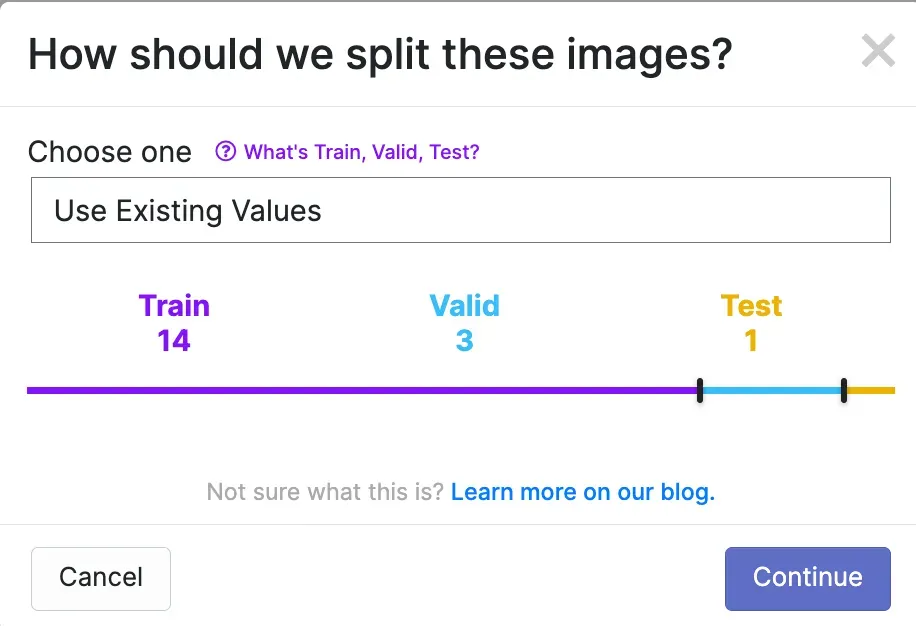
You can upload videos as well as images. We already have enough images for our project, but we'll talk through this process in case you want to add video data to your projects in the future.
To upload a video, go to the Upload tab in the Roboflow sidebar and drag in a video. You can also paste in the URL of a YouTube video you want to upload.

When you upload a video, you will be asked how many images should be captured per second. If you choose "1 frame / second", an image will be taken every second from the video and saved to Roboflow.
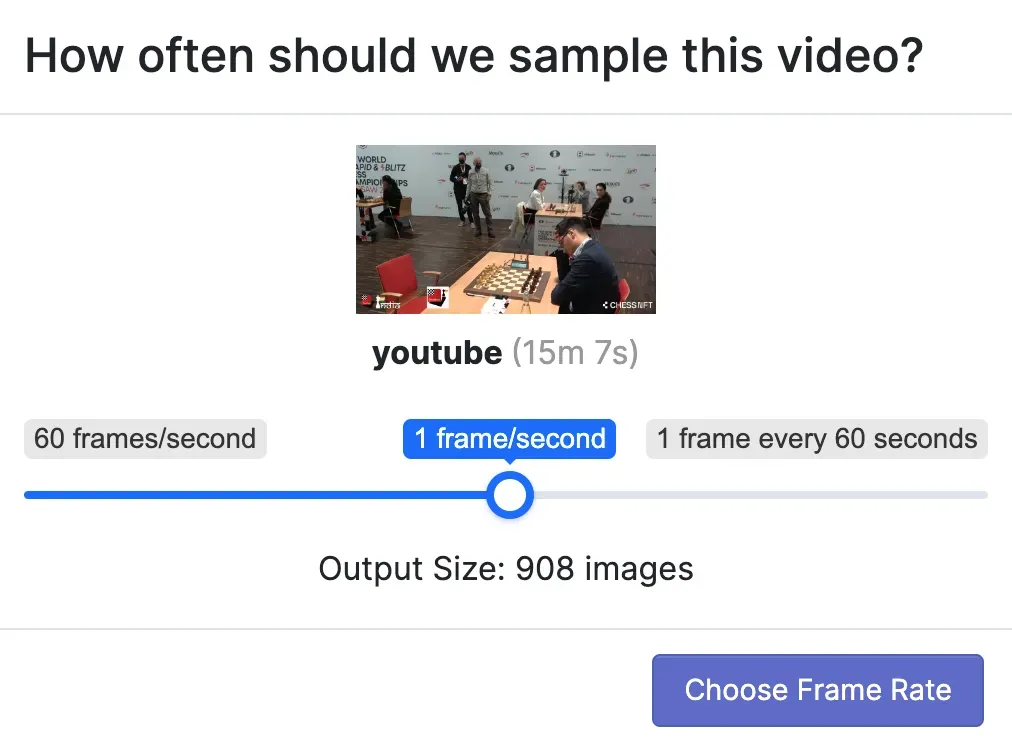
When you have selected an option, click "Choose Frame Rate". This will begin the process of collecting images from the video.
Annotate Images
One of the images in the sample dataset is not yet annotated. You will use Roboflow Annotate to add a box around the unlabeled screws in the image.
Annotations are the answer key from which your model learns. The more annotated images we add, the more information our model has to learn what each class is (in our case, more images will help our model identify what is a screw).
Use your cursor to drag a box around the area on the chess board you want to annotate. A box will appear in which you can enter the label to add. In the example below, we will add a box around a screw, and assign the corresponding class:
We have just added a "bounding box" annotation to our image. This means we have drawn a box around the object of interest. Bounding boxes are a common means of annotation for computer vision projects.
You can use publicly available models hosted on Roboflow Universe, our dataset community, for label assist, too.
You can also label object detection datasets with polygons, which are shapes with multiple points drawn around an object. Using polygons to label for object detection may result in a small boost in model performance.
Polygons are essential for instance segmentation projects where you want to identify the exact location, to the pixel, of an object in an image. Roboflow offers a few tools to help with labeling with polygons. You can manually label polygons using the Polygon annotation tool or you can use Smart Polygon to label objects with one click.
To enable Smart Polygon, click the cursor with sparkles icon in the left sidebar, above the magic wand icon. A window will pop up that asks what version of Smart Polygon you want to enable. Click "Enhanced".
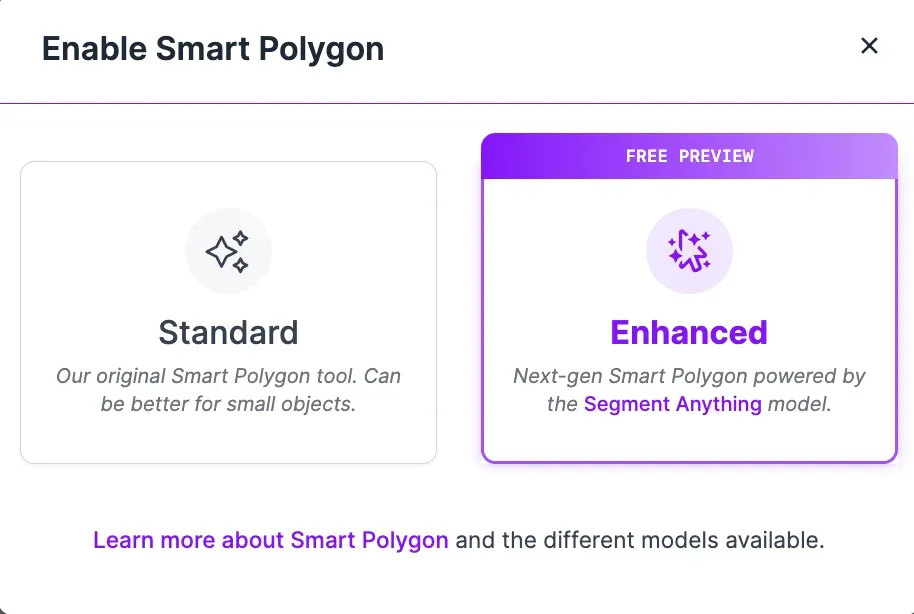
When Smart Polygon has loaded, you can point and click anywhere on an image to create a label. When you hover over an object, a red mask will appear that lets you see what region of the object Smart Polygon will label if you click.
Annotation Comments and History
Need help from a team member on an annotation? Want to leave yourself a note for later on a particular image? We have you covered. Click the speech bubble icon on the sidebar of the annotation tool. Then, click the place on the image you want to leave a comment.
If you have multiple people working with you on a project, you can tag them by using the @
sign, followed by their name. They will get a notification that you have commented and requested their assistance.
We can see the history of our annotated image in the sidebar:
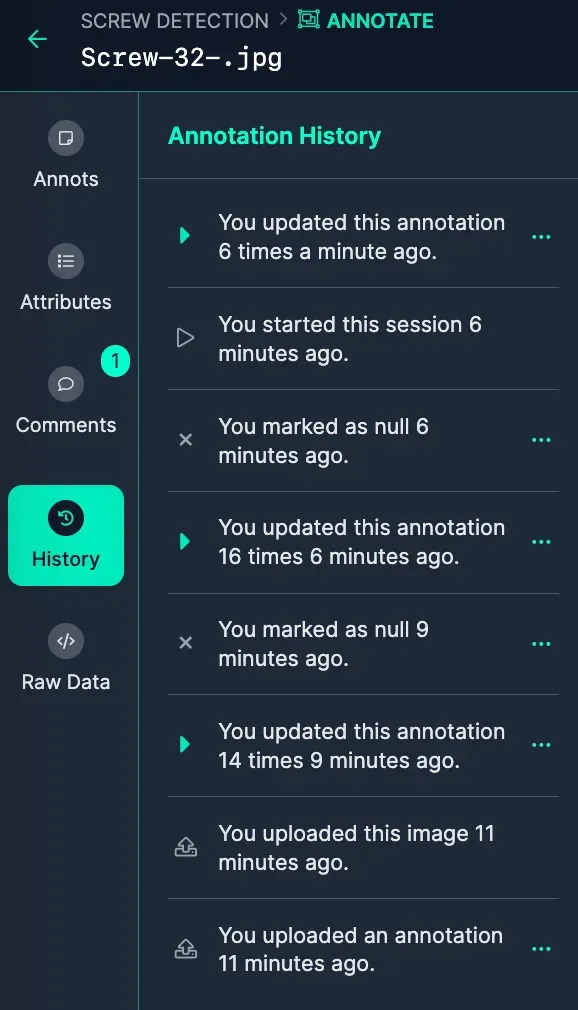
To view a project at a previous point in the history, hover over the state in history that you want to preview.
Add Images to Dataset
Once you have annotated your image, you need to add it into your dataset for use in training a model. To do so, exit out of the annotation tool and click "Annotate" in the sidebar. Then, click on the card in the "Annotating" section that shows there is one annotated image for review:
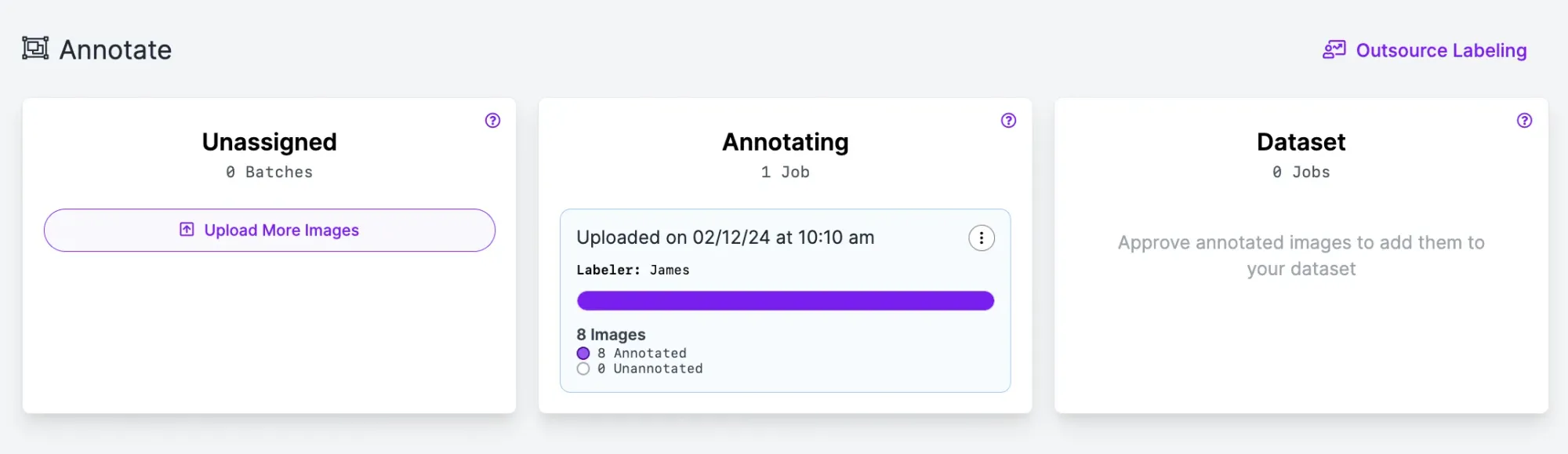
Next, click "Add Images to Dataset" to add your image to the dataset:
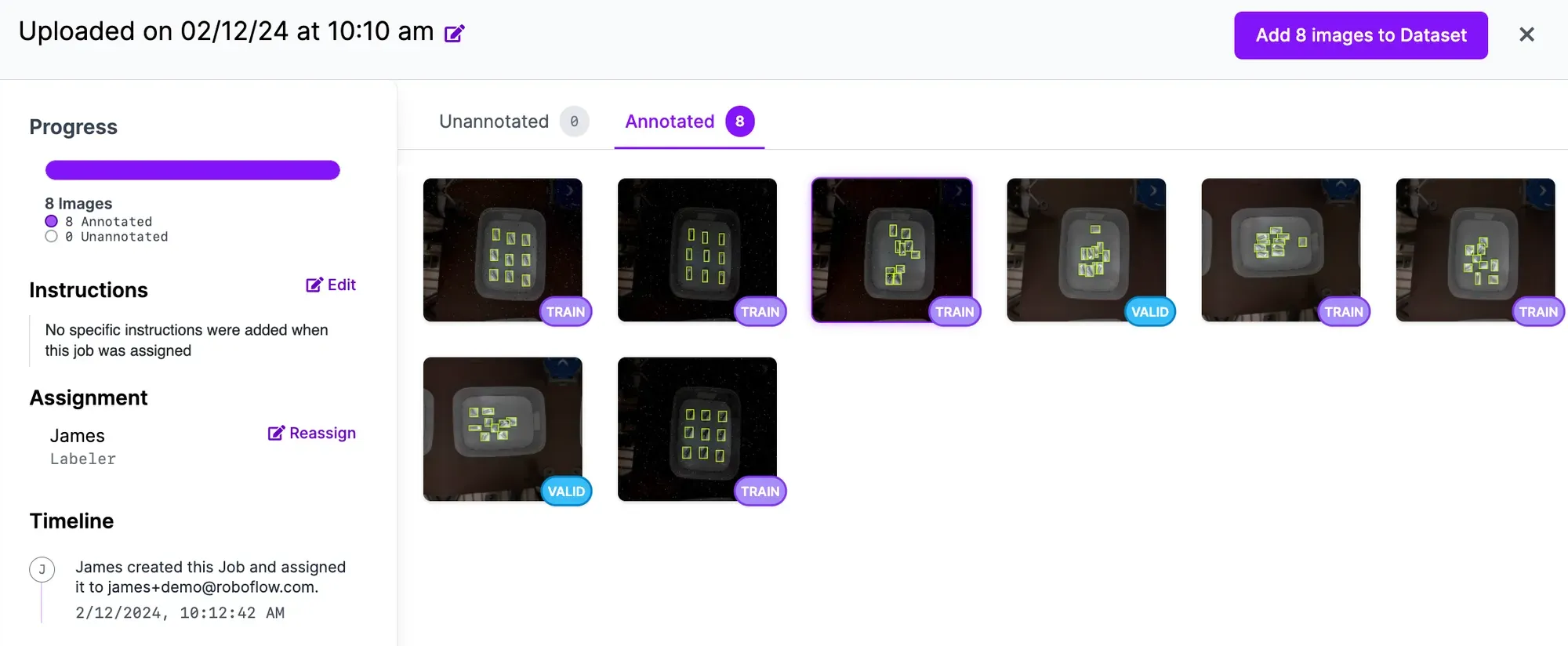
You can search the images in your dataset by clicking "Dataset" in the sidebar. The search bar runs a semantic search on your dataset to find images related to your query. For example, if we had images with nuts, bolts, screws, and plastic assembly parts, we could search through them with ease.
Preprocessing and Augmentations
After you're done annotating and have added your annotated image to your dataset, continue to generate a new version of your dataset. This creates a point-in-time snapshot of your images processed in a particular way (think of it a bit like version control for data).
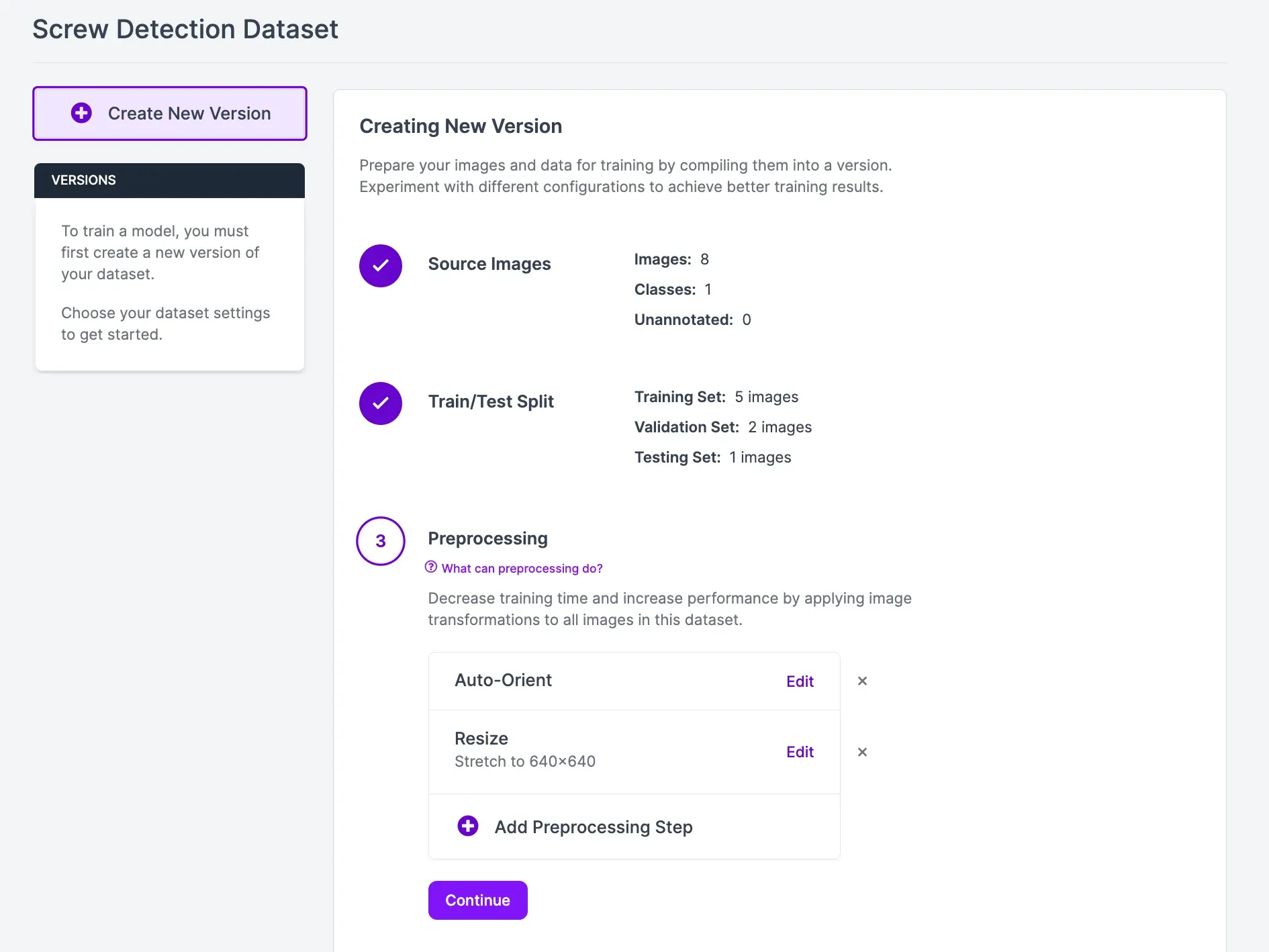
From here, we can apply any preprocessing and augmentation steps that we want to our images. Roboflow seamlessly makes sure all of your annotations correctly bound each of your labeled objects -- even if you resize, rotate, or crop.
You can also choose to augment your images which generates multiple variations of each source image to help your model generalize better.
Roboflow supports auto-orient corrections, resizing, grayscaling, contrast adjustments, random flips, random 90-degree rotations, random 0 to N-degree rotations, random brightness modifications, Gaussian blurring, random shearing, random cropping, random noise, and much more. To better understand these options, refer to our documentation.
We usually recommend applying no augmentations on your first model training job. This is so that you can understand how your model performs without augmentations. If your model performs poorly without augmentations, it is likely you need to revisit your dataset to ask questions like: Are all my images labeled? Are my images consistently labeled? Do my images contain a balance of the classes I want to identify?
With that said, we have done some experimentation so you can achieve the best performance without tinkering with augmentation for this screw dataset.
For this project, we are going to apply two augmentations: Flip and Rotate.
We recommend these augmentations for our screw dataset since screws can appear at any angle and we want to identify a screw no matter how it is positioned.
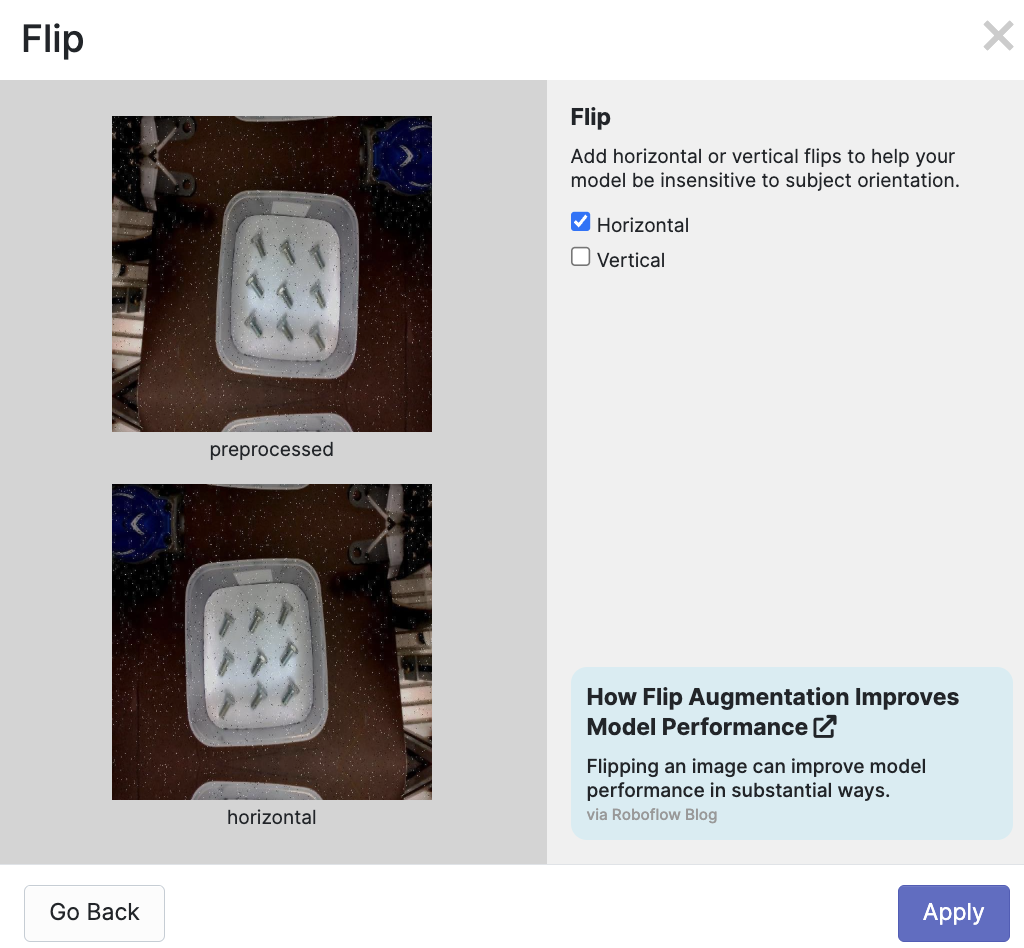
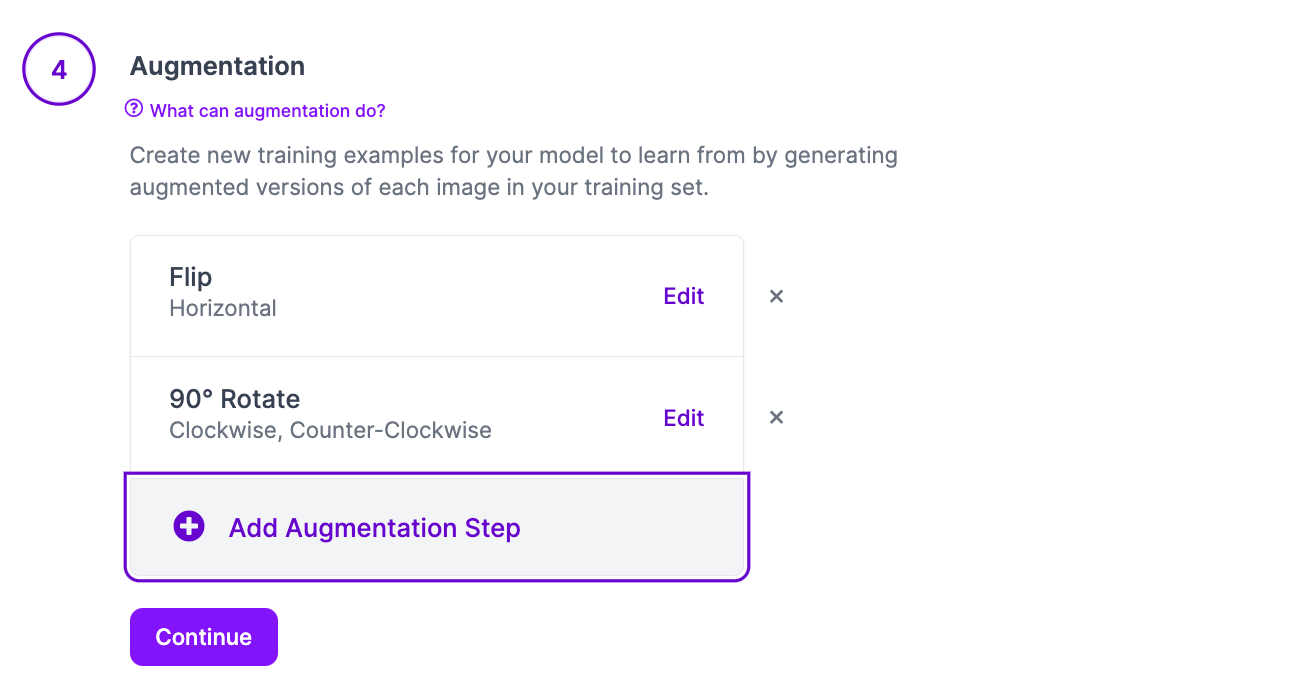
Applying augmentations to our dataset.
With that said, these augmentations may not work for your data. If you are identifying an object that will only appear at one orientation (such as might be the case on an assembly line), a flip augmentation will not help as much as others.
To learn more about applying augmentations, refer to our image augmentation and preprocessing guide.
To generate your dataset, click the "Create" button at the bottom of the page.
It will take a few moments for your dataset to be ready. Then, you can use it to start training a model.
Prepare Data for Training
Once you have generated your dataset, you can use it to train a model on the Roboflow platform.
Roboflow Train offers model types that you can train and host using Roboflow. We handle the GPU costs and also give you access to out-of-the-box optimized deployment options which we will cover later in this guide.
Your trained model can now be used in a few powerful ways:
- Model-assisted labeling speeds up labeling and annotation for adding more data into your dataset
- Rapid prototyping or testing your model on real-world data to test model performance (explained in the next section)
- Deploying to production with out-of-the-box options that are optimized for your model to run on multiple different devices (explained in the next section)
You can also export your dataset for use in training a model on your own hardware. To export your data, click "Export Dataset" and select your desired export format.
For this guide, let's train a model on the Roboflow platform.
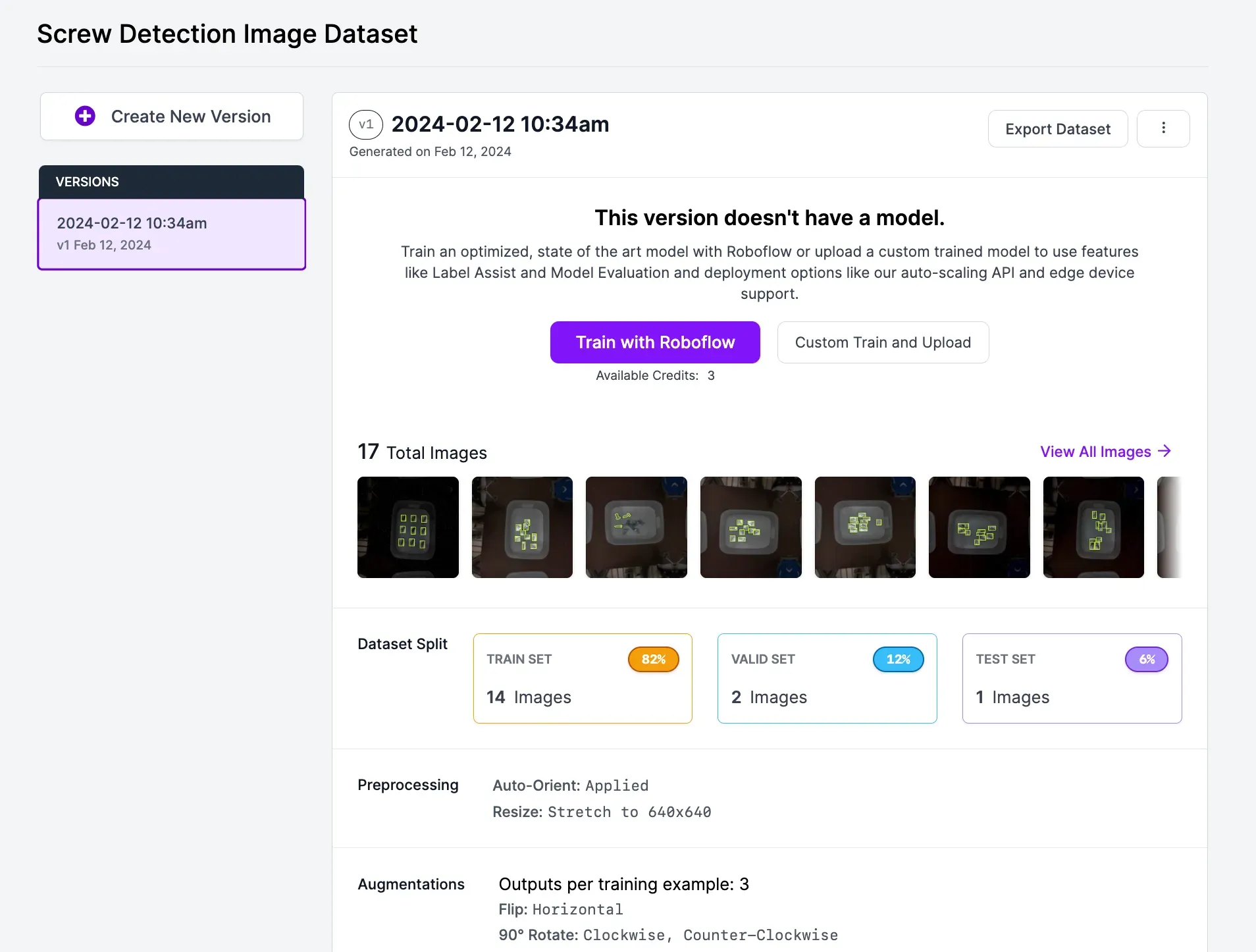
To get started, click the "Train with Roboflow" button. A window will appear where you can configure your model training job.
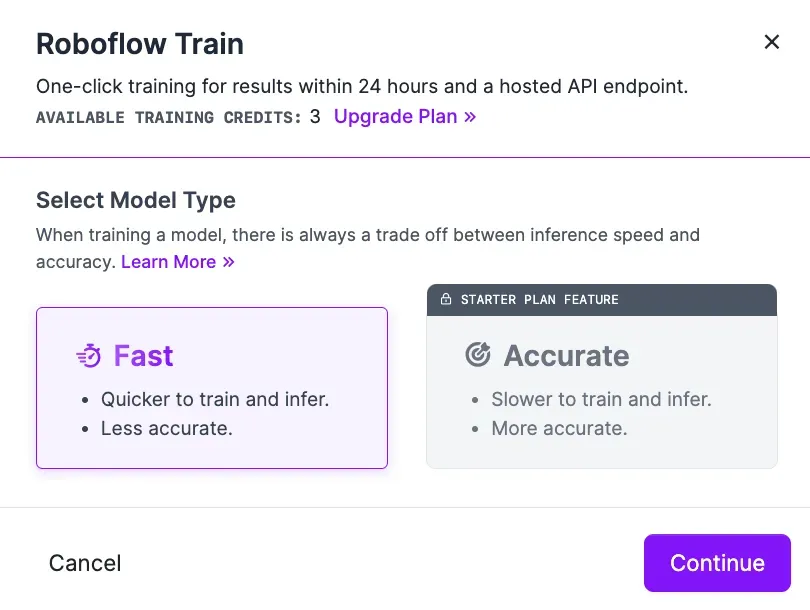
First, you will be asked to choose which model to train. Each model has performance tradeoffs that you'll want to test for your unique use case.
For this guide, choose "Fast". You will then be asked to choose from what checkpoint you want to train.
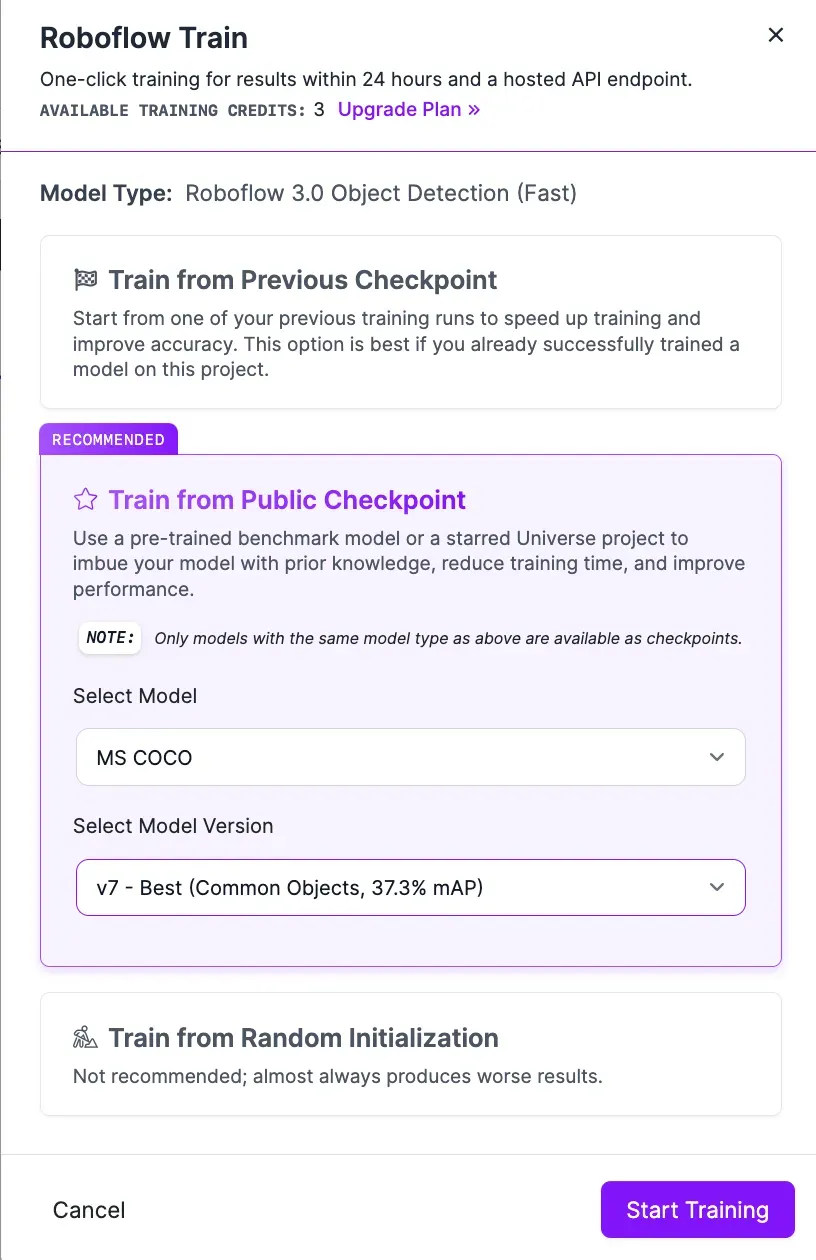
For the first version of a new model, we recommend training from the MS COCO checkpoint. This uses a model trained on the Microsoft COCO dataset as a "checkpoint" from which your model will start training. This should lead to the best performance you can achieve in your first model training job.
When you have trained a version of a model, you can train using your last model as a checkpoint. This is ideal if you have trained a model that performs well that you are looking to improve. You can also use models you have starred from Universe as a checkpoint.
Click "Start Training" to start training your model.
This will take between a few minutes and a day depending on how many images are in your dataset. Because our screw dataset contains less than a dozen images, we can expect the training process will not take too long.
When you start training your model, an estimate will appear that shows roughly how long we think it will take for your model to train. You will see the message "Training machine starting..." while your model training job is allocated to a server. This may take a few moments.
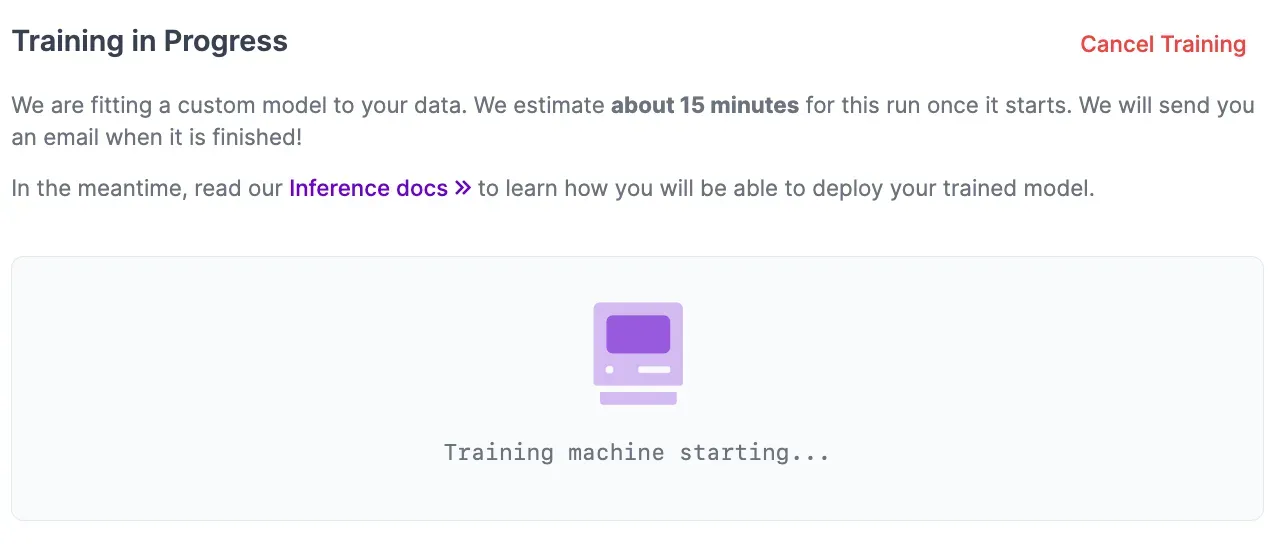
When a machine has been assigned your dataset from which to train a model, a graph will appear on the page. This graph shows how your model is learning in real-time. As your model trains, the numbers may jump up and down. Over the long term, the lines should reach higher points on the chart.
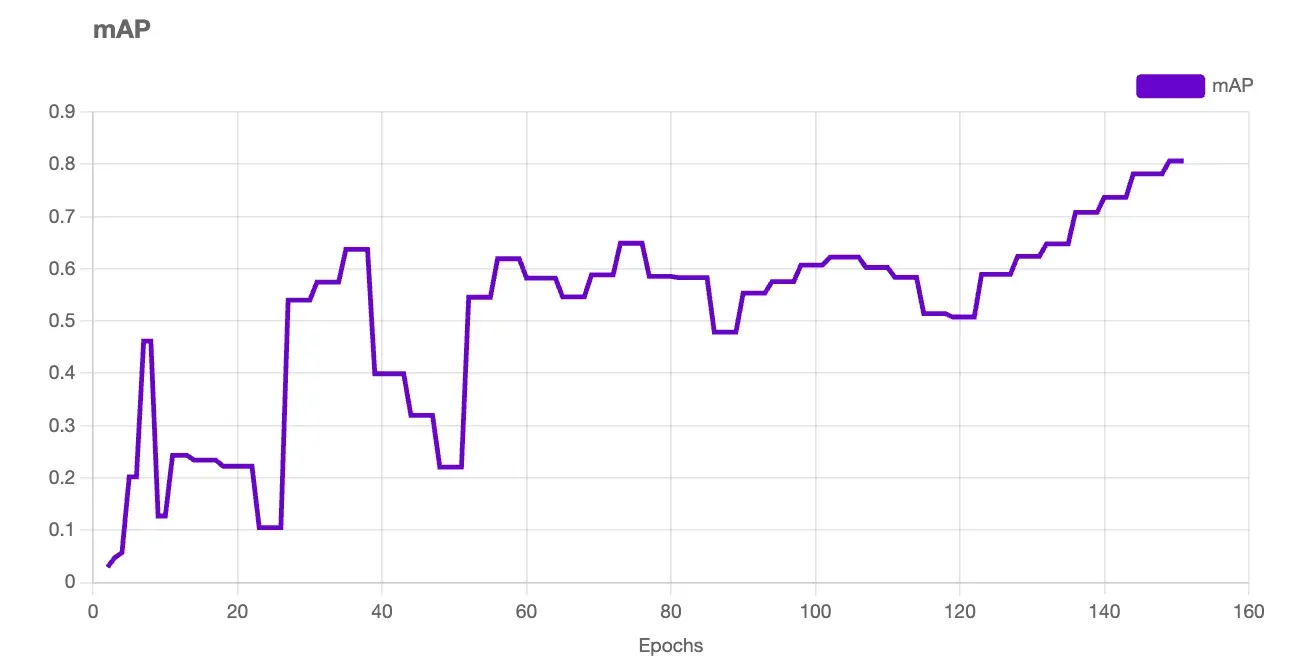
The higher the mean average precision (mAP) is, the better.
The mAP, precision, and recall statistics tell us about the performance of our model. You can learn more about what these statistics tell you in our guide to mAP, precision, and recall.
You'll receive an email once your model has finished training. The email contains the training results for you to see how the model performed. If you need to improve performance, we have recommendations on how to do that.
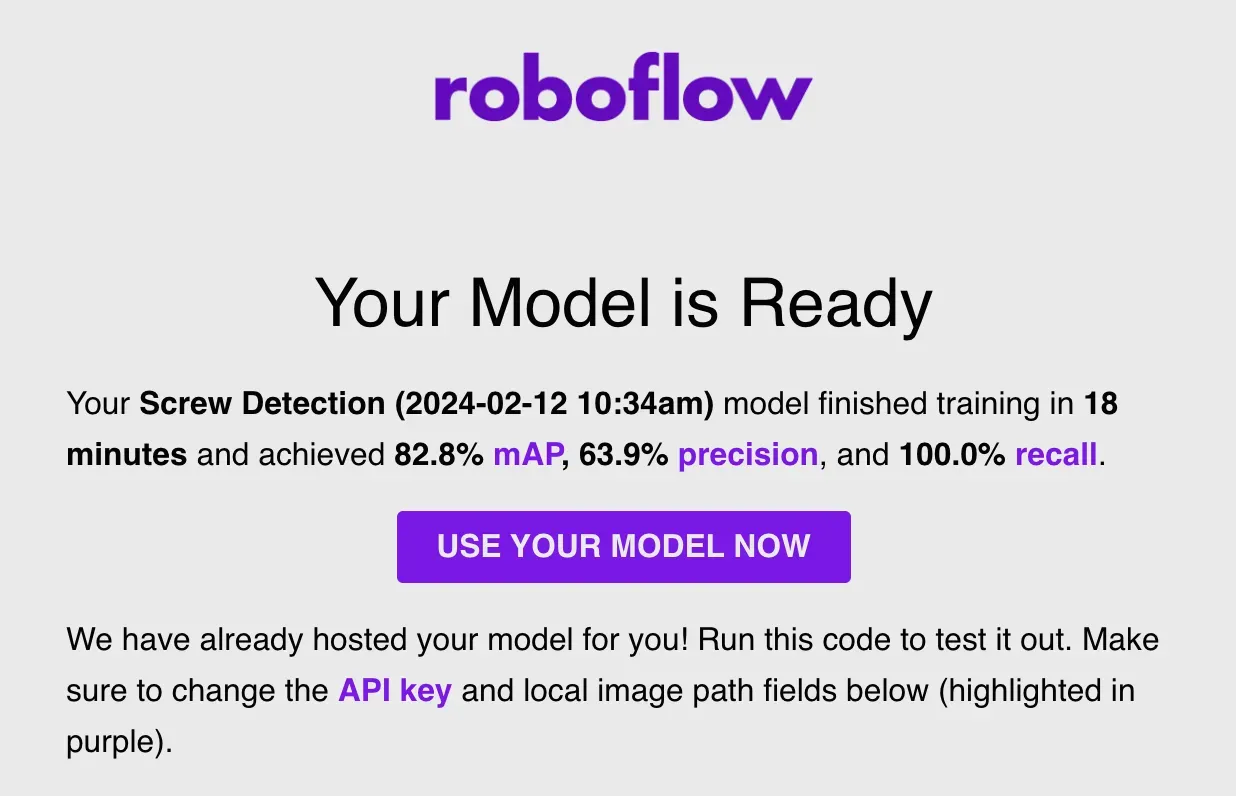
View your training graphs for a more detailed view of model performance.
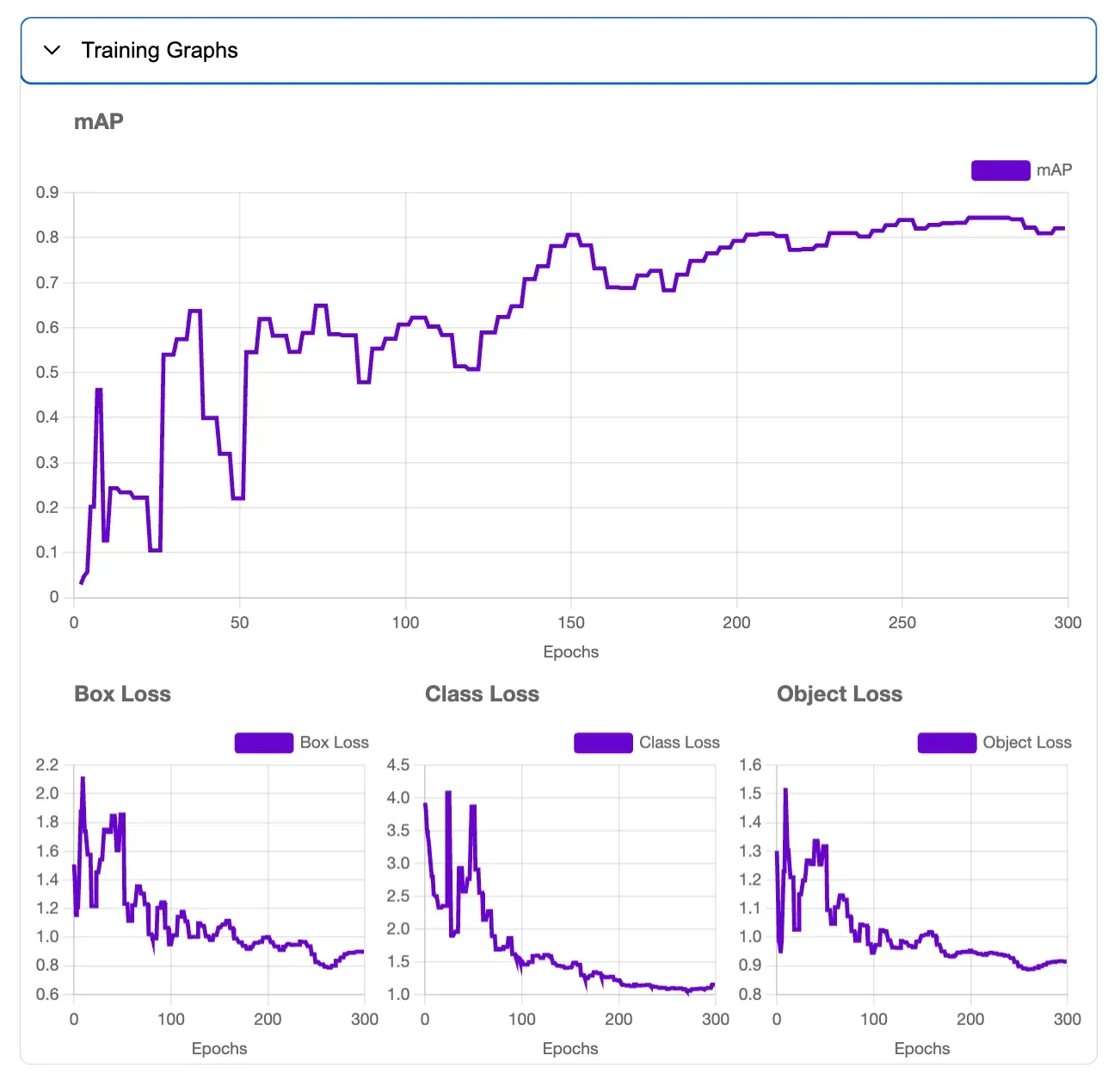
Deploying a Computer Vision Model
Your trained model, hosted by Roboflow, is optimized and ready to be used across multiple deployment options. If you're unsure of where might be best to deploy your model, we have a guide that lists a range of deployment methods and when they are useful that can be used to navigate that decision.
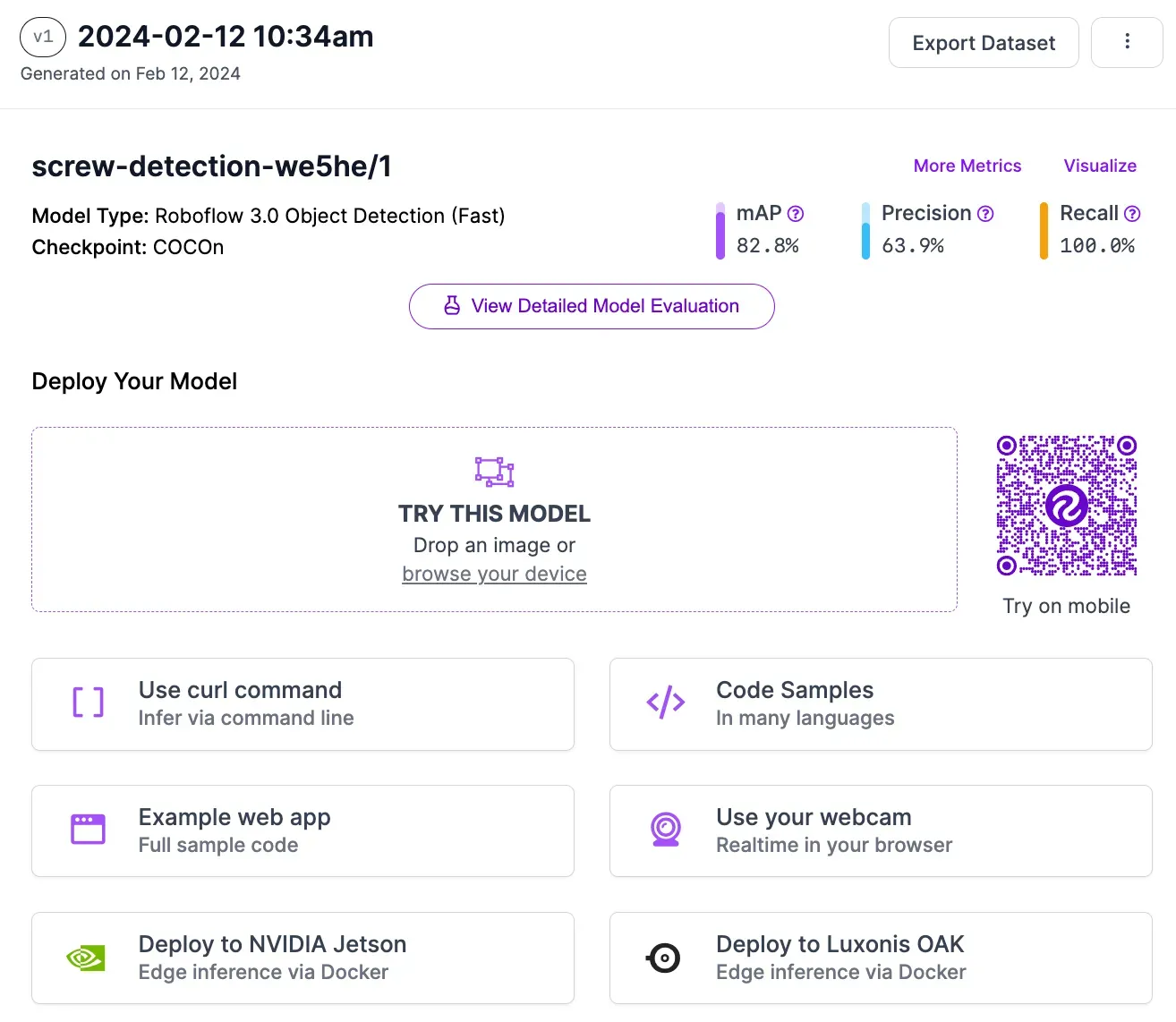
The fastest ways to test your new model and see the power of computer vision are:
- Drag-and-drop an image onto the screen to see immediate inference results.
- Use your webcam to see real-time inference results with our sample app and then dive deeper into how to use a webcam in production.
- Try the example web app to try an image URL.
From this screen, you can also deploy using options that make it easy to use your model in a production application whether via API or on an edge device. With the Roboflow Hosted API (Remote Server) method, your model is run in the cloud so you don't need to worry about the hardware capabilities of the device on which you are running your model.
Click "Visualize" in the sidebar or drag-and-drop an image into the Roboflow dashboard to test your model.
Here is an example of our screw model running on an image:
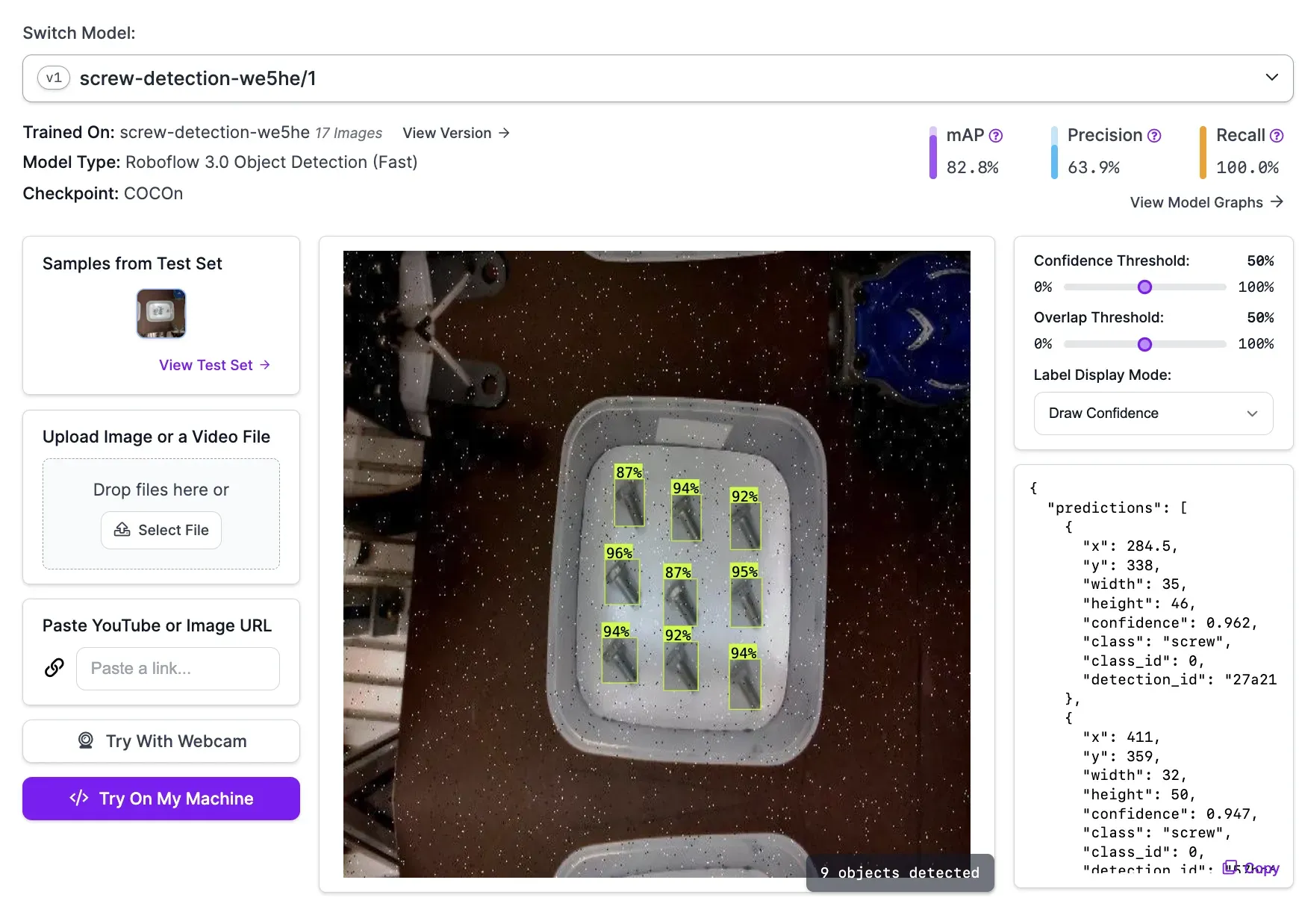
Our model successfully identified nine screws.
We could then connect this model with business logic. For example, we could have a system that counts the number of screws in a container. If there are not ten screws in a container on an assembly line, the container could be rejected and sent back for further review by a human.
We will build this logic in the next step.
On-Device Inference
You can deploy your model on your own hardware with Roboflow Inference. Roboflow Inference is a high-performance open source computer vision inference server. Roboflow Inference runs offline, enabling you to run models in an environment without an internet connection.
You can run models trained on or uploaded to Roboflow with Inference. This includes models you have trained as well as any of the 50,000+ pre-trained vision models available on Roboflow Universe. You can also run foundation models like CLIP, SAM, and DocTR.
Roboflow Inference can be run either through a pip package or in Docker. Inference works on a range of devices and architectures, including:
- x86 CPU (i.e. a PC)
- ARM CPU (i.e. Raspberry Pi)
- NVIDIA GPUs (i.e. an NVIDIA Jetson, NVIDIA T4)
To learn how to deploy a model with Inference, refer to the Roboflow Inference Deployment Quickstart widget. This widget provides tailored guidance on how to deploy your model based on the hardware you are using.
Let's run our screw detection model on our own hardware using Inference.
To get started, install Inference with pip:
pip install inference
We also need to install Supervision, a Python package with utilities for use in working with vision models:
pip install supervision
We are now ready to write logic to count screws.
Create a new Python file and add the following code:
from inference import get_roboflow_model
import supervision as sv
import cv2
image_file = "image.jpeg"
image = cv2.imread(image_file)
model = get_roboflow_model(model_id="counting-screws/1")
results = model.infer(image)
detections = sv.Detections.from_roboflow(results[0].dict(by_alias=True, exclude_none=True))
if len(detections) == 10:
print("10 screws counted. Package ready to move on.")
else:
print(len(detections), "screws counted. Package is not ready.")
This code will print a message that tells us if there are ten screws in a box.
Above, replace:
image.jpeg
with the name of the image you want to use with your model, and;counting-screws/1
with your Roboflow model ID. Learn how to retrieve your model ID.
Let's run the code with the following image:
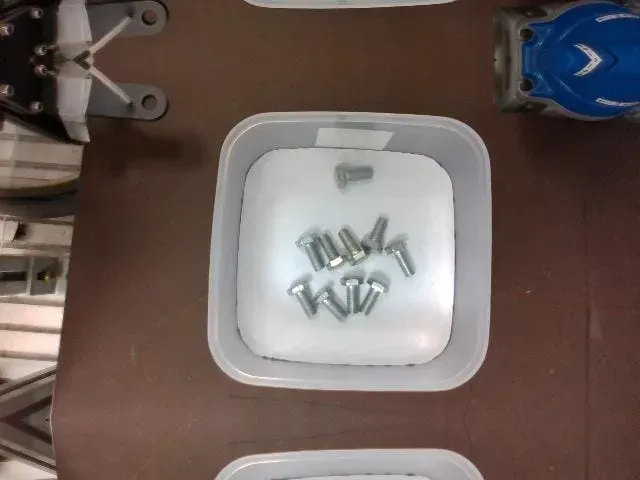
Our script returns:
10 screws counted. Package ready to move on.
We have successfully verified the number of screws in the container.
To learn more about using Inference to deploy models, refer to the Inference documentation.
For example, here is an example of a football player detection model where frames were processed on-device with Inference:
Regardless of your deployment method, we suggest taking a data-centric approach to improving your model over time by using active learning.
The benefit of using Roboflow Train and Roboflow Deploy is that we make it easy for you to test deployment options, change deployment options, or use multiple deployment options in your application.
As always, reference our Documentation or Community Forum if you have feedback, suggestions, or questions.
We’re excited to see what you build with Roboflow!