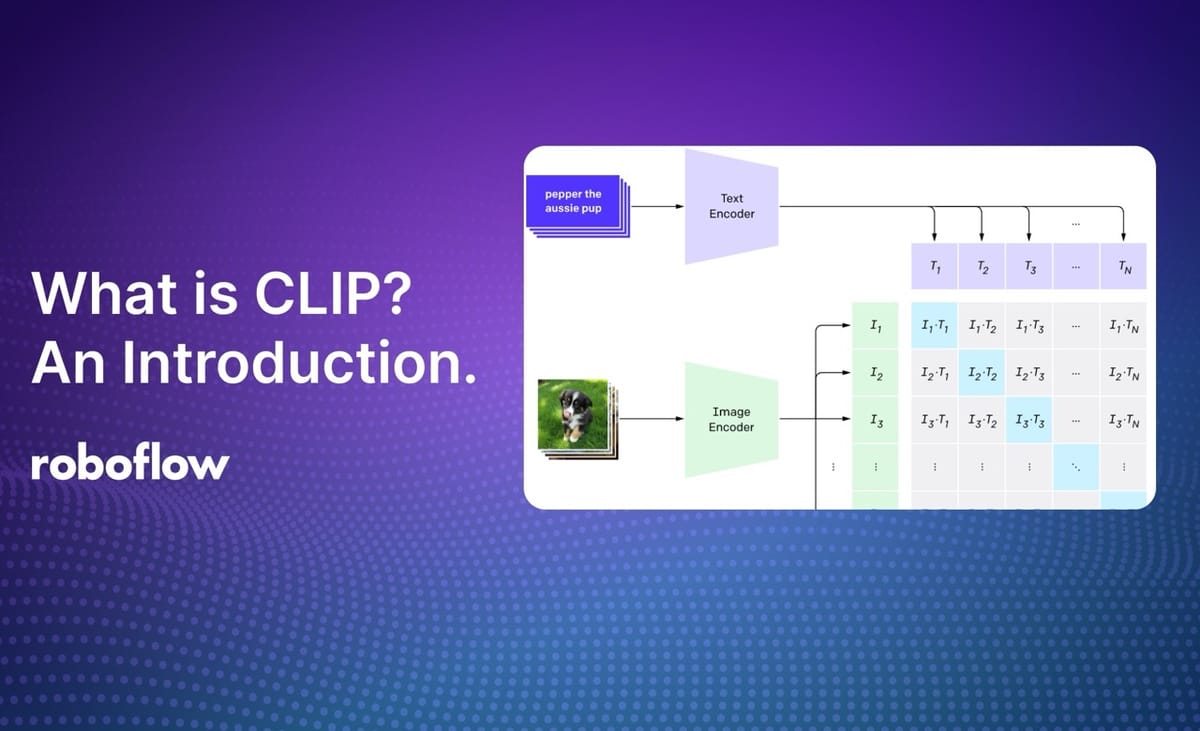
CLIP is a gigantic leap forward, bringing many of the recent developments from the realm of natural language processing into the mainstream of computer vision: unsupervised learning, transformers, and multimodality to name a few. The burst of innovation it has inspired shows its versatility.
And this is likely just the beginning. There has been scuttlebutt recently about the coming age of "foundation models" in artificial intelligence that will underpin the state of the art across many different problems in AI; I think CLIP is going to turn out to be the bedrock model for computer vision.
In this post, we aim to catalog the continually expanding use cases for CLIP; we will update it periodically.
Prefer video content? Subscribe to our YouTube channel.
What is CLIP?
In a nutshell, CLIP is a multimodal model that combines knowledge of English-language concepts with semantic knowledge of images.
From the OpenAI CLIP repository, "CLIP (Contrastive Language-Image Pre-Training) is a neural network trained on a variety of (image, text) pairs. It can be instructed in natural language to predict the most relevant text snippet, given an image, without directly optimizing for the task, similarly to the zero-shot capabilities of GPT-2 and 3."
Depending on your background, this may make sense -- but there's a lot in here that may be unfamiliar to you. Let's unpack it.
- CLIP is a neural network model.
- It is trained on 400,000,000 (image, text) pairs. An (image, text) pair might be a picture and its caption. So this means that there are 400,000,000 pictures and their captions that are matched up, and this is the data that is used in training the CLIP model.
- "It can predict the most relevant text snippet, given an image." You can input an image into the CLIP model, and it will return for you the likeliest caption or summary of that image.
- "without directly optimizing for the task, similarly to the zero-shot capabilities of GPT-2 and 3." Most machine learning models learn a specific task. For example, an image classifier trained on classifying dogs and cats is expected to do well on the task we've given it: classifying dogs and cats. We generally would not expect a machine learning model trained on dogs and cats to be very good at detecting raccoons. However, some models -- including CLIP, GPT-2, and GPT-3 -- tend to perform well on tasks they aren't directly trained to do, which is called "zero-shot learning."
- "Zero-shot learning" is when a model attempts to predict a class it saw zero times in the training data. So, using a model trained on exclusively cats and dogs to then detect raccoons. A model like CLIP, because of how it uses the text information in the (image, text) pairs, tends to do really well with zero-shot learning – even if the image you're looking at is really different from the training images, your CLIP model will likely be able to give a good guess for the caption for that image.
To put this all together, the CLIP model is:
- a neural network model built on hundreds of millions of images and captions,
- can return the best caption given an image, and
- has impressive "zero-shot" capabilities, making it able to accurately predict entire classes it's never seen before!
CLIP can just as easily distinguish between an image of a "cat" and a "dog" as it can between "an illustration of Deadpool pretending to be a bunny rabbit" and "an underwater scene in the style of Vincent Van Gogh" (even though it has definitely never seen those things in its training data). This is because of its generalized knowledge of what those English phrases mean and what those pixels represent.
This is in contrast to traditional computer vision models which disregard the context of their labels (in other words, a "normal" image classifier works just as well if your labels are "cat" and "dog" or "foo" and "bar"; behind the scenes it just converts them into a numeric identifier with no particular meaning).
Imagine you're given a filing cabinet and 100,000 documents. Your job is to put them each into the correct folder out of the 1,000 folders in the cabinet. At the end of the day your boss will judge your work.
Unfortunately, you're illiterate. You start off doing no better than random chance. But, one day you realize that some of the documents are crisp and white and some are tattered and yellowed. You decide to sort the documents by color and split them evenly between the folders. Your boss is pleased and gives you slightly better marks that day. Day by day you try to discover new things that are different about the files: some are long and some are short. Some have photos and some do not. Some are paper-clipped and some are stapled.
Then, one day, after years and years of tirelessly deciphering this enigma, trying different combinations of folders and ways of dividing the documents, improving your performance bit by bit, your boss introduces you to your new coworker. You furrow your brow trying to figure out how you're going to train her to execute on your delicate and complicated system.
But, to your surprise, on her very first day, her performance exceeds yours! It turns out your new coworker is CLIP and she knows how to read. Instead of having to guess what the folders should contain she simply looks at their labels. And instead of discovering clues about the documents bit by bit, she already has prior knowledge of what those indecipherable glyphs represent.
In real world tasks, the "glyphs" are actually patterns of pixels (features) representing abstractions like colors, shapes, textures, and patterns (and even concepts like people and locations).
How does CLIP work?
In order for images and text to be connected to one another, they must both be embedded. You've worked with embeddings before, even if you haven't thought of it that way. Let's go through an example. Suppose you have one cat and two dogs. You could represent that as a dot on a graph, like below:
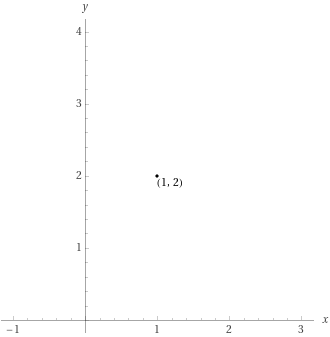
It may not seem very exciting, but we just embedded that information on the X-Y grid that you probably learned about in middle school (formally called Euclidean space). You could also embed your friends' pet information on the same graph and/or you could have chosen plenty of different ways to represent that information (e.g. put dogs before cats, or add a third dimension for raccoons).
I like to think of embedding as a way to smash information into mathematical space. We just took information about dogs and cats and smashed it into mathematical space. We can do the same thing with text and with images!
The CLIP model consists of two sub-models called encoders:
- a text encoder that will embed (smash) text into mathematical space.
- an image encoder that will embed (smash) images into mathematical space.
Whenever you fit a supervised learning model, you have to find some way to measure the "goodness" or the "badness" of that model – the goal is to fit a model that is as "most good" and "least bad" as possible.
The CLIP model is no different: the text encoder and image encoder are fit to maximize goodness and minimize badness.
So, how do we measure "goodness" and "badness?"
In the image below, you'll see a set of purple text cards going into the text encoder. The output for each card would be a series of numbers. For example, the top card, pepper the aussie pup
would enter the text encoder – the thing smashing it into mathematical space – and come out as a series of numbers like (0, 0.2, 0.8).
The exact same thing will happen for the images: each image will go into the image encoder and the output for each image will also be a series of numbers. The picture of, presumably Pepper the Aussie pup, will come out like (0.05, 0.25, 0.7).
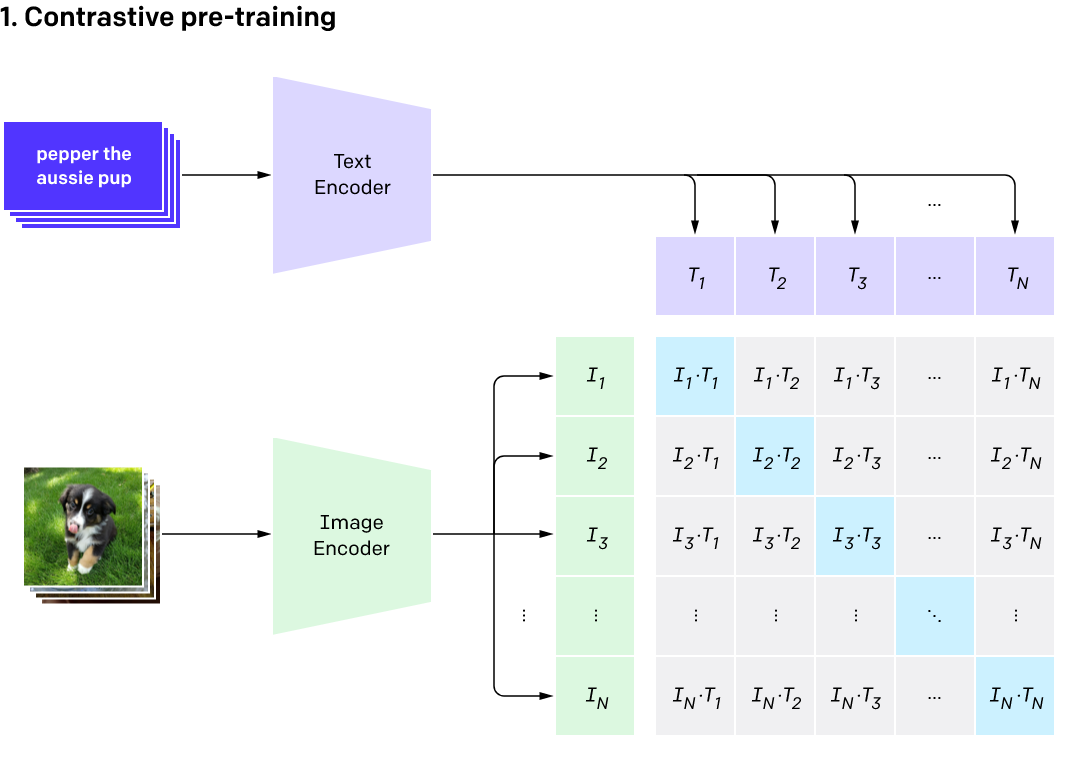
"Goodness" of our model
In an ideal world, the series of numbers for the text "pepper the aussie pup" will be very close (identical) to the series of numbers for the corresponding image. In fact, this should be the case everywhere: the series of numbers for the text should be very close to the series of numbers for the corresponding image. One way for us to measure "goodness" of our model is how close the embedded representation (series of numbers) for each text is to the embedded representation for each image.
There is a convenient way to calculate the similarity between two series of numbers: the cosine similarity. We won't get into the inner workings of that formula here, but rest assured that it's a tried and true method of seeing how similar two vectors, or series of numbers, are. It's how we score submissions to our paint.wtf game, shown below.
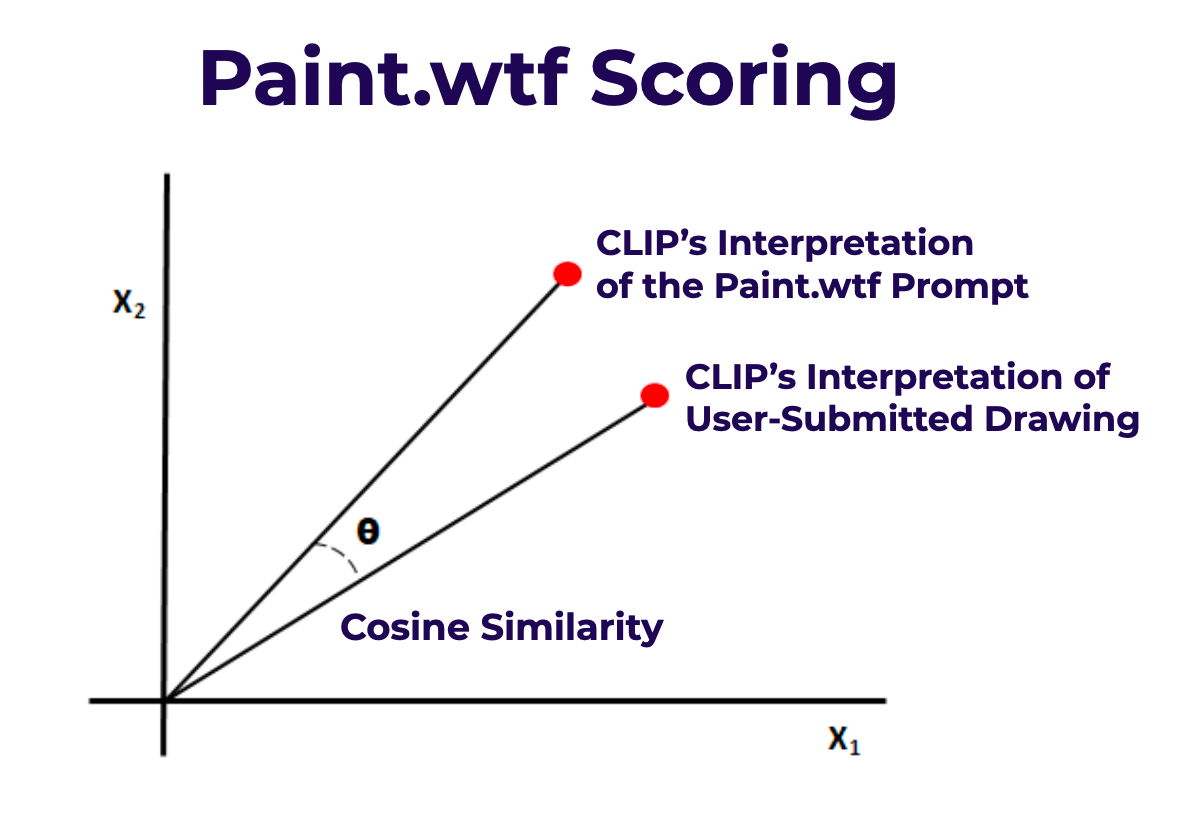
In the pre-training phase image above, the light blue squares represent where the text and image coincide. For example, T1 is the embedded representation of the first text; I1 is the embedded representation of the first image. We want the cosine similarity for I1 and T1 to be as high as possible. We want the same for I2 and T2, and so on for all of the light blue squares. The higher these cosine similarities are, the more "goodness" our model has!
"Badness" of our model
At the same time as wanting to maximize the cosine similarity for each of those blue squares, there are a lot of grey squares that indicate where the text and image don't align. For example, T1 is the text "pepper the aussie pup" but perhaps I2 is an image of a raccoon.
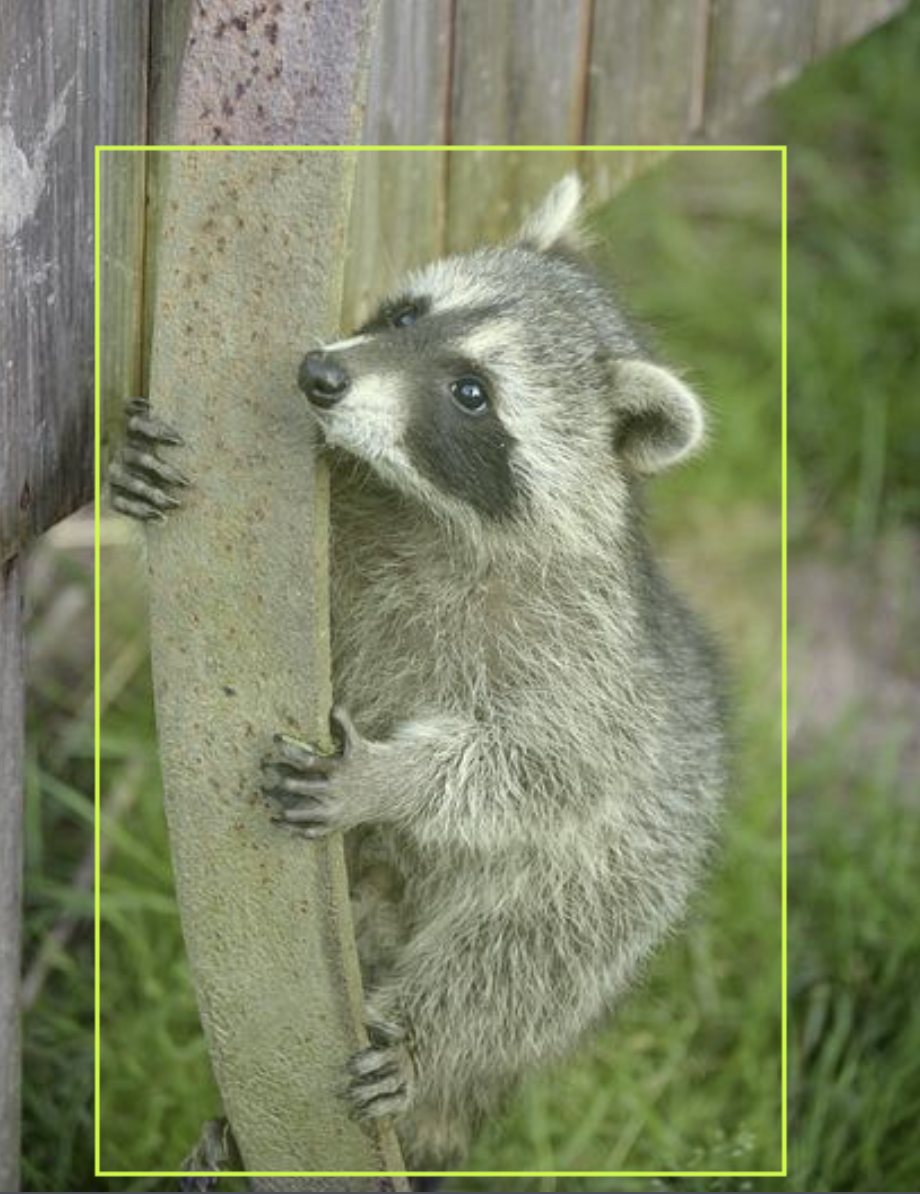
Cute though this raccoon is, we want the cosine similarity between this image (I2) and the text pepper the aussie pup
to be pretty small, because this isn't Pepper the Aussie pup!
While we wanted the blue squares to all have high cosine similarities (as that measured "goodness"), we want all of the grey squares to have low cosine similarities, because that measures "badness."
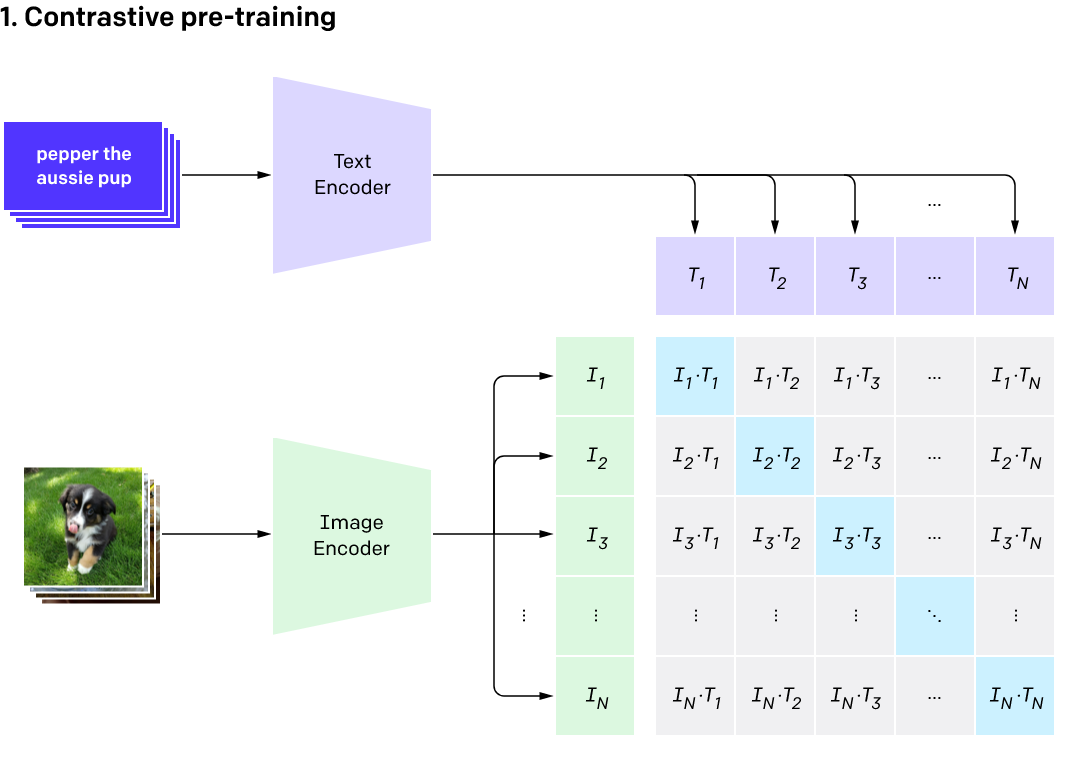
How do the text and image encoders get fit?
The text encoder and image encoder get fit at the same time by simultaneously maximizing the cosine similarity of those blue squares and minimizing the cosine similarity of the grey squares, across all of our text+image pairs.
Note: this can take a very long time depending on the size of your data. The CLIP model trained on 400,000,000 labeled images. The training process took 30 days across 592 V100 GPUs. This would have cost $1,000,000 to train on AWS on-demand instances!
Once the model is fit, you can pass an image into the image encoder to retrieve the text description that best fits the image – or, vice versa, you can pass a text description into the model to retrieve an image, as you'll see in some of the applications below!
CLIP is a bridge between computer vision and natural language processing.
Why is CLIP cool?
With this bridge between computer vision and natural language processing, CLIP has a ton of advantages and cool applications. We'll focus on the applications, but a few advantages to call out:
- Generalizability: Models are usually super brittle, capable of knowing only the very specific thing you trained them to do. CLIP expands knowledge of classification models to a wider array of things by leveraging semantic information in text. Standard classification models completely discard the semantic meaning of the class labels and simply enumerated numeric classes behind the scenes; CLIP works by understanding the meaning of the classes.
- Connecting text / images better than ever before: CLIP may quite literally be the "world's best caption writer" when considering speed and accuracy together.
- Already-labeled data: CLIP is built on images and captions that were already created; other state-of-the-art computer vision algorithms required significant additional human time spent labeling.
Why does @OpenAI's CLIP model matter?https://t.co/X7bnSgZ0or
— Joseph Nelson (@josephofiowa) January 6, 2021
CLIP Use Cases
One of the neatest aspects of CLIP is how versatile it is. When introduced by OpenAI they noted two use-cases: image classification and image generation. But in the 9 months since its release it has been used for a far wider variety of tasks.
Some of the uses of CLIP so far:
- CLIP has been used to index photos on sites like Unsplash.
- One Twitter user took celebrities including Elvis Presley, Beyoncé, and Billie Eilish, and used CLIP and StyleGAN to generate portraits in the style of "My Little Pony."
- Have you played Pictionary? Now you can play online at paint.wtf, where you'll be judged by CLIP.
- CLIP could be used to easily improve NSFW filters!
- Find photos matching a mood – for example, via a poetry passage.
- OpenAI has also created DALL-E, which creates images from text.
Let's talk about a few use cases in detail.
Image classification
OpenAI originally evaluated CLIP as a zero-shot image classifier. They compared it against traditional supervised machine learning models and it performed nearly on par with them without having to be trained on any specific dataset.
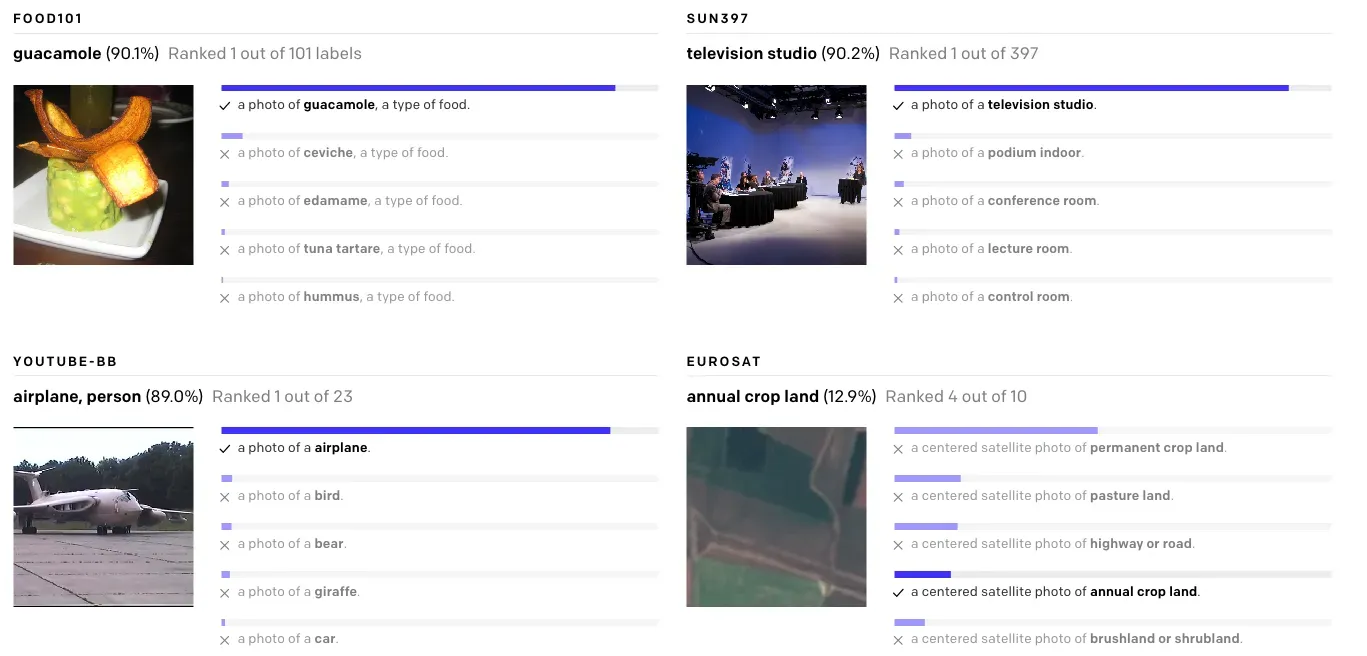
One challenge with traditional approaches to image classification is that you need lots of training examples that closely resemble the distribution of the images it will see in the wild. Because of this, CLIP does better on this task the less training data there is available.
To try CLIP for image classification, follow our CLIP tutorial. If you're having trouble getting good results, read our tips on prompt engineering.
Image Generation
DALL-E was developed by OpenAI in tandem with CLIP. It's a generative model that can produce images based on a textual description; CLIP was used to evaluate its efficacy.
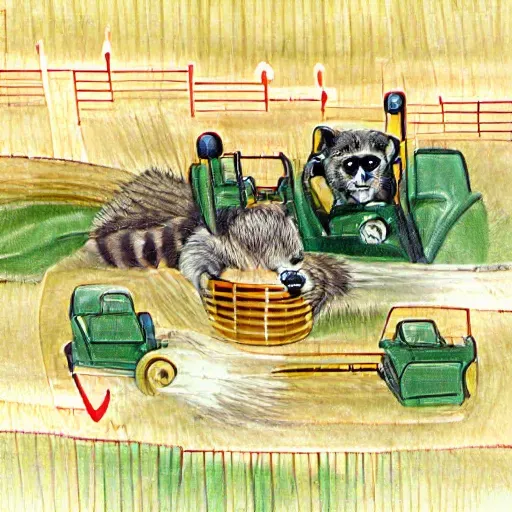
The DALL-E model has still not been released publicly, but CLIP has been behind a burgeoning AI generated art scene. It is used to "steer" a GAN (generative adversarial network) towards a desired output. The most commonly used model is Taming Transformers' CLIP+VQGAN which we dove deep on here.
Content Moderation
One extension of image classification is content moderation. If you ask it in the right way, CLIP can filter out graphic or NSFW images out of the box. We demonstrated content moderation with CLIP in a post here.
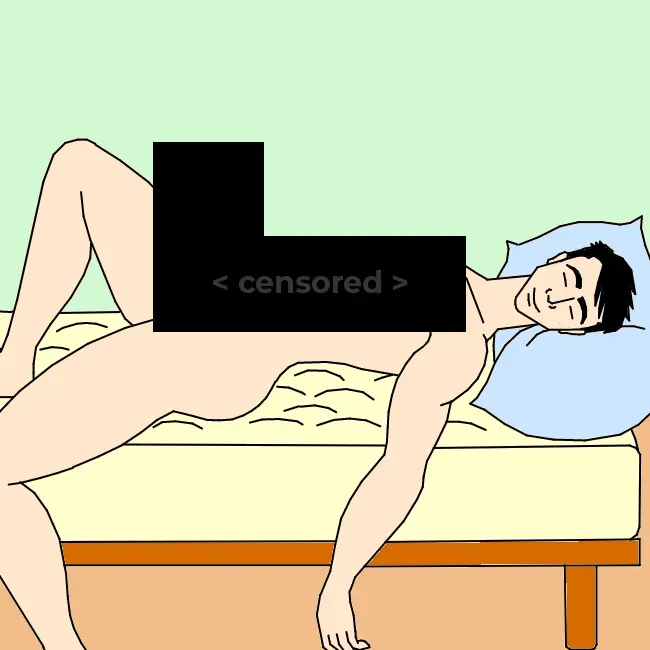
Image Search
Because CLIP doesn't need to be trained on specific phrases, it's perfectly suited for searching large catalogs of images. It doesn't need images to be tagged and can do natural language search.
Yurij Mikhalevich has already created an AI-powered command image line search tool called rclip. It wouldn't surprise me if CLIP spawns a Google Image Search competitor in the near future.
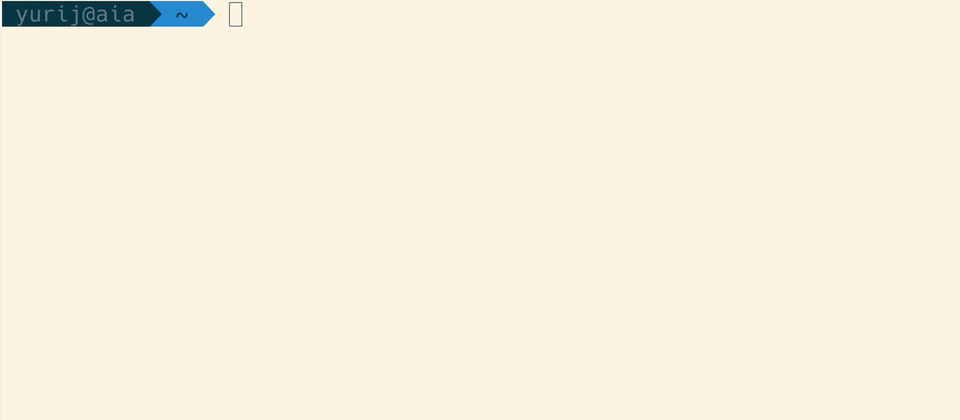
Image Similarity
Apple's Neuralhash semantic image similarity algorithm has been in the news a lot recently for how they're applying it to scanning user devices for CSAM. We showed how you can use CLIP to find similar images in the exact same way Apple's Neuralhash works.
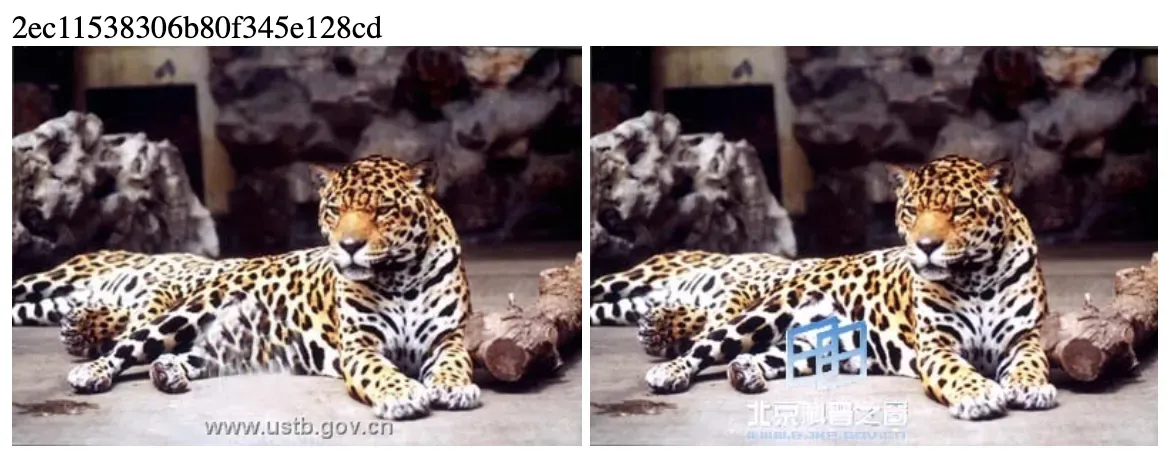
The applications of being able to find similar images go far beyond scanning for illegal content, though. It could be used to search for copyright violations, create a clone of Tineye, or an advanced photo library de-duplicator.
Image Ranking
It's not just factual representations that are encoded in CLIP's memory. It also knows about qualitative concepts as well (as we learned from the Unreal engine trick).
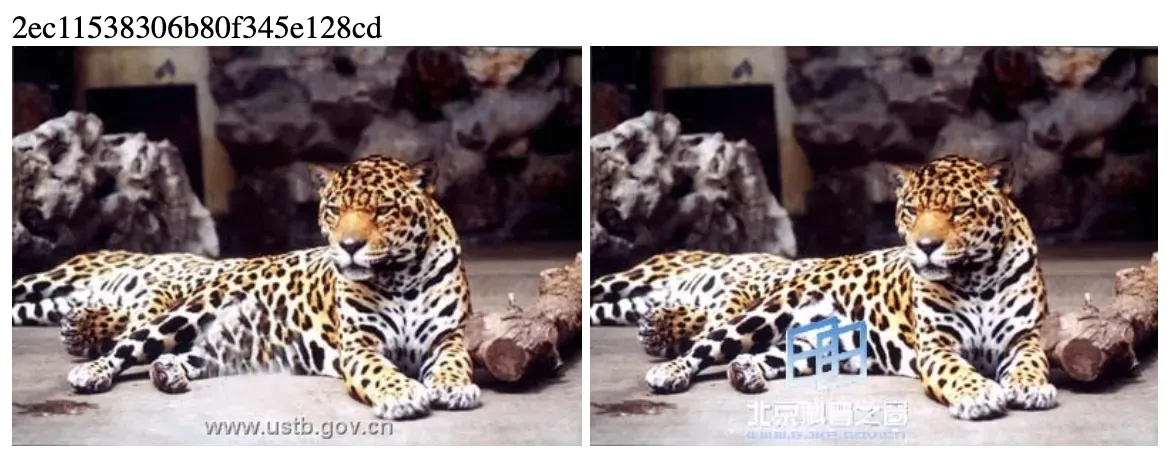
We used this to create a CLIP judged Pictionary-style game, but you could also use it to create a camera app that "scores" users' photos by "searching" for phrases like "award winning photograph" or "professional selfie of a model" to help users decide which images to keep and which ones to trash, for example.
Object Tracking
As an extension of image similarity, we've used CLIP to track objects across frames in a video. It uses an object detection model to find items of interest then crops the image and uses CLIP to determine if two detected objects are the same or difference instance of that object across different frames of a video.
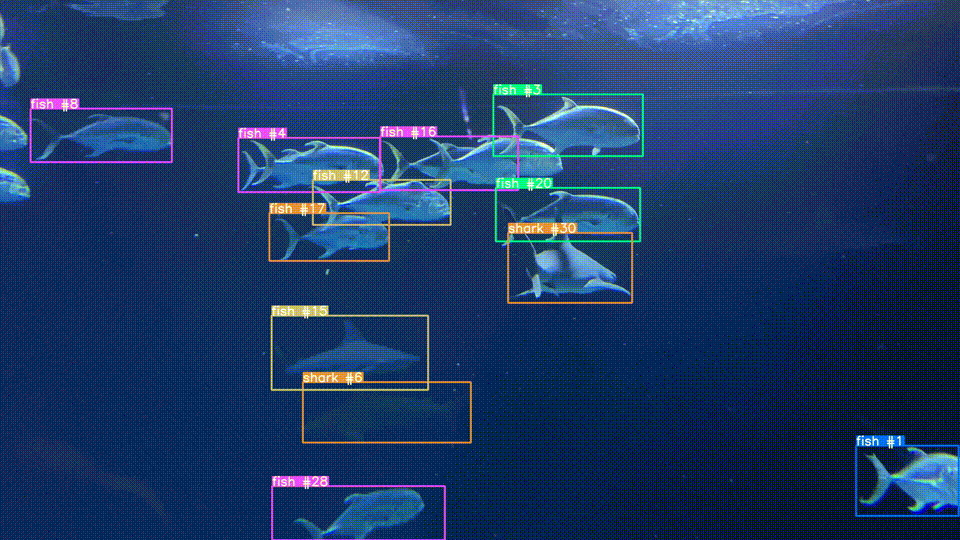
Robotics Control
The CLIPort model combines CLIP with another model to allow robots to perform abstract tasks like folding laundry or sorting cubes without having to be given explicit instructions for how to accomplish the objective.
Image Captioning
With the CLIP prefix captioning repo, the feature vectors from CLIP have been wired into GPT-2 to output an English description for a given image.
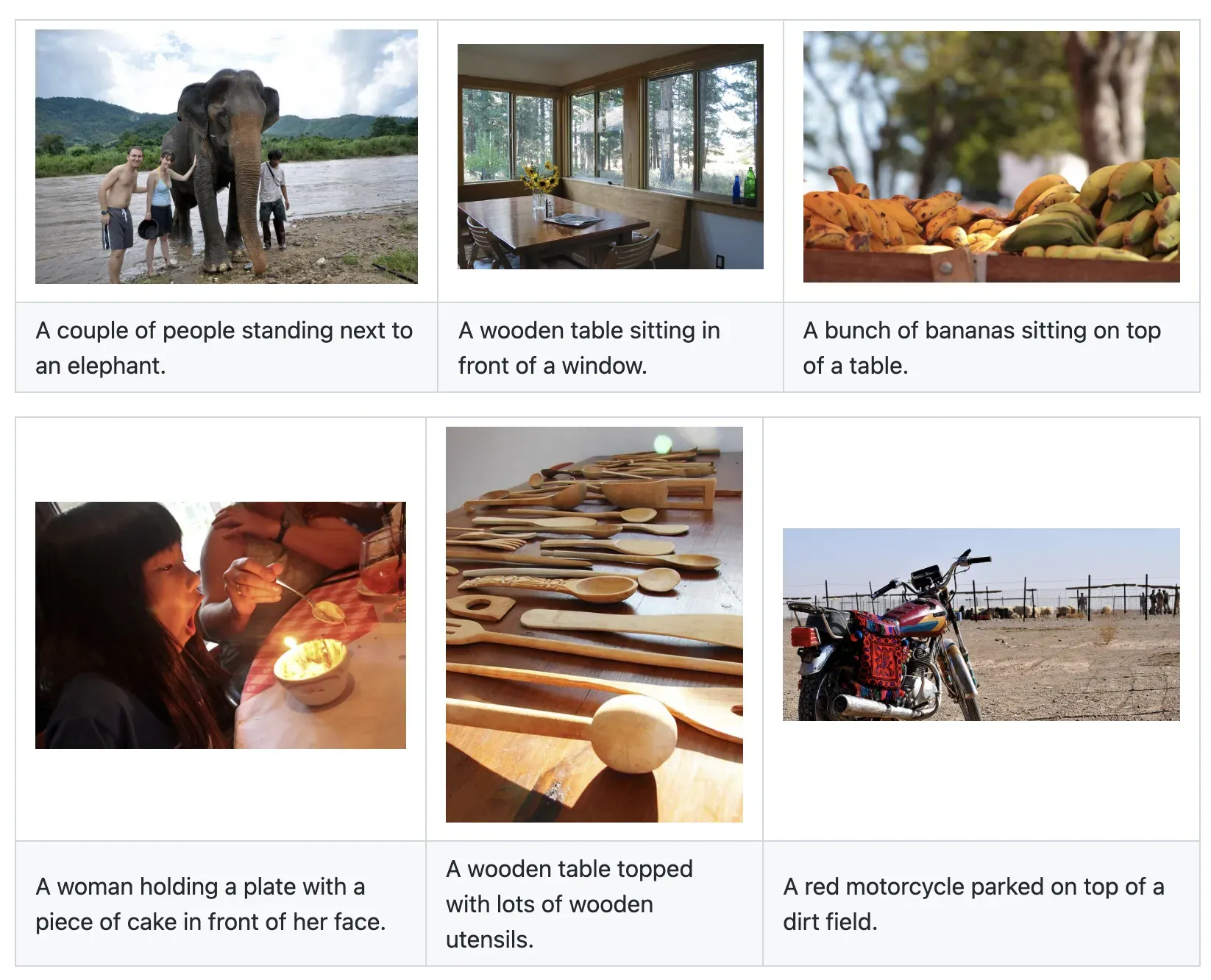
Deciphering Corrupted Images
In a new paper, called Inverse Problems Leveraging Pre-Trained Contrastive Representations, researchers have shown how CLIP can be used to interpret extremely distorted or corrupted images.
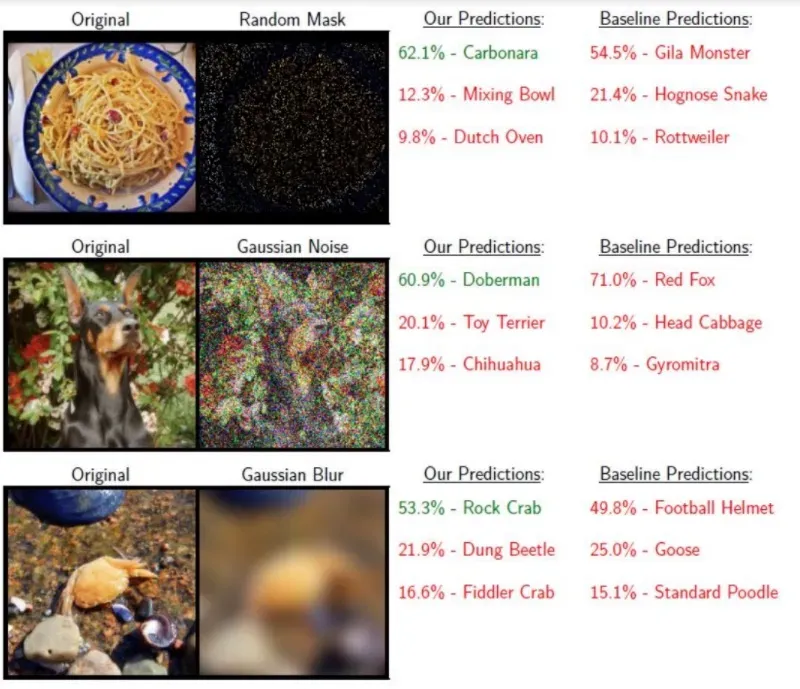
How to Use CLIP
You can use CLIP to classify images through Roboflow Inference, an open source computer vision inference server that runs on your hardware.
Let's show how to use CLIP on a webcam feed so that we can test the model in real time.
To get started, first install Inference and the Inference CLIP package:
pip install inference inference[clip]
Next, retrieve your API key from your Roboflow dashboard. If you do not already have a Roboflow account, you can create one for free.
Once you have retrieved your API key, set it in an environment variable called ROBOFLOW_API_KEY
:
export ROBOFLOW_API_KEY=""
Next, create a new Python file and add the following code:
import cv2
import inference
from inference.core.utils.postprocess import cosine_similarity
from inference.models import Clip
clip = Clip()
prompt = "a coffee cup"
text_embedding = clip.embed_text(prompt)
def render(result, image):
# get the cosine similarity between the prompt & the image
similarity = cosine_similarity(result["embeddings"][0], text_embedding[0])
# scale the result to 0-100 based on heuristic (~the best & worst values I've observed)
range = (0.15, 0.40)
similarity = (similarity-range[0])/(range[1]-range[0])
similarity = max(min(similarity, 1), 0)*100
# print the similarity
text = f"{similarity:.1f}%"
cv2.putText(image, text, (10, 310), cv2.FONT_HERSHEY_SIMPLEX, 12, (255, 255, 255), 30)
cv2.putText(image, text, (10, 310), cv2.FONT_HERSHEY_SIMPLEX, 12, (206, 6, 103), 16)
# print the prompt
cv2.putText(image, prompt, (20, 1050), cv2.FONT_HERSHEY_SIMPLEX, 2, (255, 255, 255), 10)
cv2.putText(image, prompt, (20, 1050), cv2.FONT_HERSHEY_SIMPLEX, 2, (206, 6, 103), 5)
# display the image
cv2.imshow("CLIP", image)
cv2.waitKey(1)
# start the stream
inference.Stream(
source="webcam",
model=clip,
output_channel_order="BGR",
use_main_thread=True,
on_prediction=render
)
In this code, we use the inference.Stream()
method to run CLIP over all frames in the video. We set the prompt a coffee cup
. The code above will calculate a CLIP embedding for the prompt. This CLIP vector will then be compared to CLIP vectors calculated for each frame in the video.
We show text on each frame that shows how similar CLIP thinks the embedding corresponding with the frame is to our prompt embedding.
In this example, if we hold up a coffee cup, the similarity will increase:
When the coffee cup comes into view, the percentage similarity increases; when the coffee cup goes out of view, the similarity decreases.
You could amend the code above to work with multiple prompts. Then, you could take the prompt with the most similar embedding to the embedding for each frame and use it as a label.
The following guides may be useful as you continue experimenting with CLIP:
- How to Analyze and Classify Video with CLIP
- Zero-Shot Content Moderation with OpenAI's New CLIP Model
- How to Build a Semantic Image Search Engine with Supabase and OpenAI CLIP
- Auto-Label Classification Datasets Using CLIP
Future OpenAI CLIP Use-Cases
CLIP will be used in many more creative ways in the future. We're working on a CLIP API to make it easier to try building these types of projects. If you'd like early access, please reach out.
Fine-Tuning CLIP
Unfortunately, for many hyper-specific use-cases (eg examining the output of microchip lithography) or identifying things invented since CLIP was trained in 2020 (for example, the unique characteristics of CLIP+VQGAN creations), CLIP isn't capable of performing well out of the box for all problems. It should be possible to extend CLIP (essentially using it as a fantastic checkpoint for transfer learning) with additional data.
Object detection
In much the same way we used CLIP to do object tracking, it's conceivable that you could use it for object detection as well. One naive way of doing this would be to feed every candidate anchor box into CLIP and determine which ones are the closest matches to your objects of interest.
Video Indexing
If you can classify images, it should be doable to classify frames of videos. In this way you could automatically split videos into scenes and create search indexes. Imagine searching YouTube for your company's logo and magically finding all of the places where someone happened to have used your product.
OpenAI CLIP (Contrastive Language-Image Pre-Training)
If you want to try CLIP yourself, try our tutorial or convert an object detection dataset into a classification dataset for use with CLIP.
Cite this Post
Use the following entry to cite this post in your research:
Brad Dwyer, Matt Brems. (Sep 1, 2024). What is CLIP? Contrastive Language-Image Pre-Training Explained.. Roboflow Blog: https://blog.roboflow.com/openai-clip/